Data Splitting: Breaking Down the Problem
During World War II, the extraordinary efforts of the Six Triple Eight exemplified ingenuity in overcoming logistical challenges. Faced with an overwhelming backlog of mail for soldiers, this all-Black Women's Army Corps unit adopted creative methods to sort and deliver parcels. Each team specialized in unique techniques: some handled parcels directly, others used identifying material clues on packages to determine destinations, and even scents, such as perfume, were leveraged to trace letters' origins. As a last resort, they read the letters to ensure delivery.
This approach is remarkably similar to how we split datasets in machine learning—breaking down the workload to ensure accuracy and effectiveness. In machine learning, data is divided into training and test sets, ensuring the model learns effectively while its performance is evaluated fairly. Let's explore this further.
Why is Data Splitting Important?
Balanced Learning: Training the model on a subset of data allows it to generalize patterns rather than memorize examples.
Fair Evaluation: The test set acts as unseen data, enabling us to assess the model’s ability to perform on real-world tasks.
Reduced Bias: By ensuring random distribution, we avoid skewing results toward overrepresented categories.
Just as the Six Triple Eight ensured every letter was accounted for with specialized methods, splitting data ensures every aspect of the dataset is appropriately represented for model evaluation.
Here’s an article that ties the data-splitting process in machine learning to the innovative methods used by the Six Triple Eight to manage mail, along with an explanation of the Python code for dataset splitting:
Data Splitting: Breaking Down the Problem
During World War II, the extraordinary efforts of the Six Triple Eight exemplified ingenuity in overcoming logistical challenges. Faced with an overwhelming backlog of mail for soldiers, this all-Black Women's Army Corps unit adopted creative methods to sort and deliver parcels. Each team specialized in unique techniques: some handled parcels directly, others used identifying material clues on packages to determine destinations, and even scents, such as perfume, were leveraged to trace letters' origins. As a last resort, they read the letters to ensure delivery.
This approach is remarkably similar to how we split datasets in machine learning—breaking down the workload to ensure accuracy and effectiveness. In machine learning, data is divided into training and test sets, ensuring the model learns effectively while its performance is evaluated fairly. Let's explore this further.
Why is Data Splitting Important?
Splitting data is crucial for:
Balanced Learning: Training the model on a subset of data allows it to generalize patterns rather than memorize examples.
Fair Evaluation: The test set acts as unseen data, enabling us to assess the model’s ability to perform on real-world tasks.
Reduced Bias: By ensuring random distribution, we avoid skewing results toward overrepresented categories.
Just as the Six Triple Eight ensured every letter was accounted for with specialized methods, splitting data ensures every aspect of the dataset is appropriately represented for model evaluation.
Python Code for Dataset Splitting
Here’s a practical implementation of dataset splitting in Python:
import csv import os import random # Create a dataset directory os.makedirs('dataset', exist_ok=True) # Simulate rows of data (replace `df.iterrows()` with your DataFrame) rows = [{'text': row['text'].strip(), 'label': row['category']} for idx, row in df.iterrows()] # Ensure reproducibility with a fixed random seed random.seed(42) random.shuffle(rows) # Split data into test and train sets num_test = 500 splits = {'test': rows[0:num_test], 'train': rows[num_test:]} # Save the splits as CSV files for split in ['train', 'test']: with open(f'dataset/{split}.csv', 'w', newline='') as f: writer = csv.DictWriter(f, fieldnames=['text', 'label']) writer.writeheader() for row in splits[split]: writer.writerow(row)
Lessons from the Six Triple Eight
Just as the Six Triple Eight divided their workload and leveraged diverse methods to ensure mail delivery, splitting data in machine learning is essential to optimize performance. It allows us to train and test models effectively, ensuring they can handle real-world complexities.
The Six Triple Eight’s innovation reminds us of the importance of adaptability and strategy—principles that resonate in both historical feats and modern data science.
The above is the detailed content of Data Splitting: Breaking Down the Problem. For more information, please follow other related articles on the PHP Chinese website!

Hot AI Tools

Undresser.AI Undress
AI-powered app for creating realistic nude photos

AI Clothes Remover
Online AI tool for removing clothes from photos.

Undress AI Tool
Undress images for free

Clothoff.io
AI clothes remover

Video Face Swap
Swap faces in any video effortlessly with our completely free AI face swap tool!

Hot Article

Hot Tools

Notepad++7.3.1
Easy-to-use and free code editor

SublimeText3 Chinese version
Chinese version, very easy to use

Zend Studio 13.0.1
Powerful PHP integrated development environment

Dreamweaver CS6
Visual web development tools

SublimeText3 Mac version
God-level code editing software (SublimeText3)

Hot Topics










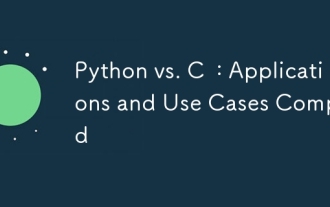
Python is suitable for data science, web development and automation tasks, while C is suitable for system programming, game development and embedded systems. Python is known for its simplicity and powerful ecosystem, while C is known for its high performance and underlying control capabilities.
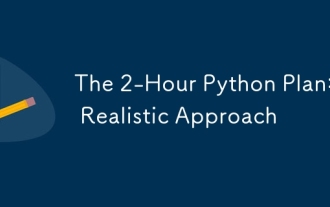
You can learn basic programming concepts and skills of Python within 2 hours. 1. Learn variables and data types, 2. Master control flow (conditional statements and loops), 3. Understand the definition and use of functions, 4. Quickly get started with Python programming through simple examples and code snippets.
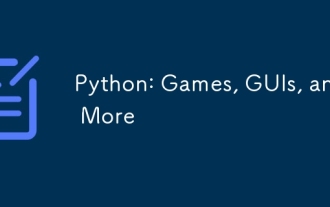
Python excels in gaming and GUI development. 1) Game development uses Pygame, providing drawing, audio and other functions, which are suitable for creating 2D games. 2) GUI development can choose Tkinter or PyQt. Tkinter is simple and easy to use, PyQt has rich functions and is suitable for professional development.
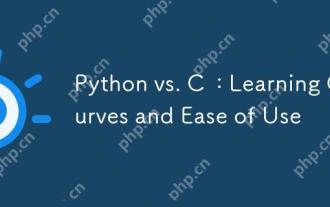
Python is easier to learn and use, while C is more powerful but complex. 1. Python syntax is concise and suitable for beginners. Dynamic typing and automatic memory management make it easy to use, but may cause runtime errors. 2.C provides low-level control and advanced features, suitable for high-performance applications, but has a high learning threshold and requires manual memory and type safety management.
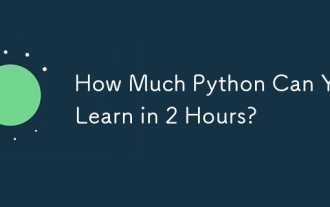
You can learn the basics of Python within two hours. 1. Learn variables and data types, 2. Master control structures such as if statements and loops, 3. Understand the definition and use of functions. These will help you start writing simple Python programs.
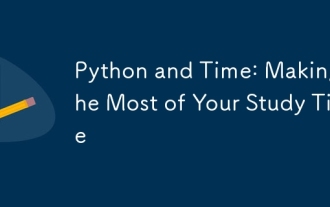
To maximize the efficiency of learning Python in a limited time, you can use Python's datetime, time, and schedule modules. 1. The datetime module is used to record and plan learning time. 2. The time module helps to set study and rest time. 3. The schedule module automatically arranges weekly learning tasks.
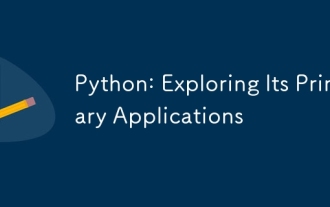
Python is widely used in the fields of web development, data science, machine learning, automation and scripting. 1) In web development, Django and Flask frameworks simplify the development process. 2) In the fields of data science and machine learning, NumPy, Pandas, Scikit-learn and TensorFlow libraries provide strong support. 3) In terms of automation and scripting, Python is suitable for tasks such as automated testing and system management.
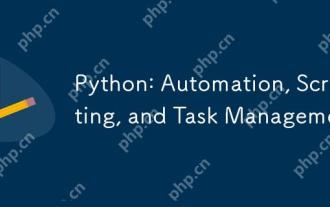
Python excels in automation, scripting, and task management. 1) Automation: File backup is realized through standard libraries such as os and shutil. 2) Script writing: Use the psutil library to monitor system resources. 3) Task management: Use the schedule library to schedule tasks. Python's ease of use and rich library support makes it the preferred tool in these areas.
