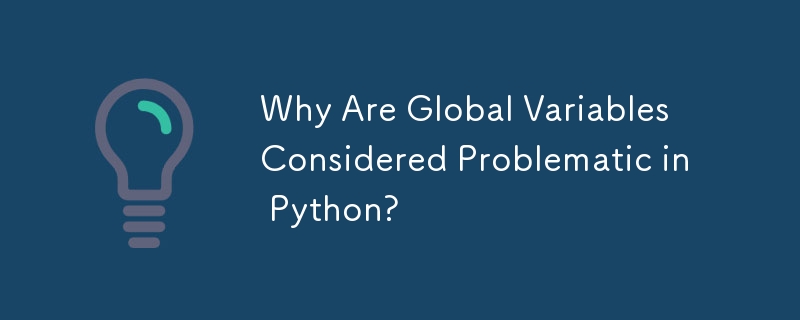
The Pitfalls of Global Variables
While Python is often hailed as a beginner-friendly language, it's essential to understand the potential drawbacks of certain programming practices. One such practice is the use of global variables, often considered an anti-pattern.
Understanding the Evil of Global Variables
Global variables, unlike local variables that are confined within a specific function or scope, can be accessed and modified by any function or code block within the same program. This unrestricted access can lead to a host of problems:
-
Hidden Side Effects: Global variables can cause functions to exhibit unexpected or misleading side effects by silently modifying their values. This can make it extremely difficult to trace errors or anticipate the behavior of a program.
-
Spaghetti Code Complexity: As the number of global variables increases, the program's structure can become convoluted and intertwined, making it challenging to understand and maintain.
-
Conflicting Modifications: Multiple functions simultaneously accessing and modifying a global variable can lead to data races, causing inconsistent or corrupted data.
Exemptions to the Rule
It's important to note that global constants, which represent immutable values that never change, are distinct from global variables. Global constants are generally considered harmless and are commonly used to define configuration settings or constants.
Alternatives to Global Variables
To avoid the pitfalls of global variables, consider alternative approaches:
-
Module-Level Variables: Define variables within a specific module and import them as needed. This provides encapsulation and limits exposure to other parts of the program.
-
Singleton Objects: Create a class representing a single instance that encapsulates the global state. This allows controlled access and modification of the data.
-
Dependency Injection: Pass necessary data as arguments to functions or methods, avoiding direct access to global variables.
Exploring the Resources
For further insights on the perils of global variables, refer to the following resources:
- [Global Variables Are Bad](https://c2.com/cgi/wiki?GlobalVariablesAreBad)
- [Why is Global State so Evil?](https://softwareengineering.stackexchange.com/questions/30506/why-is-global-state-so-evil)
- [Are global variables bad?](https://stackoverflow.com/questions/2682095/are-global-variables-bad)
The above is the detailed content of Why Are Global Variables Considered Problematic in Python?. For more information, please follow other related articles on the PHP Chinese website!