


Quick and Dirty Document Analysis: Combining GOT-OCR and LLama in Python
Let's explore a way to do OCR LLM analysis for an image. Will this be the best way given by an expert with decades of experience? Not really. But it comes from someone who takes a similar approach in real life. Think of this as a weekend project version with practical snippets rather than production-ready code. Let's dig in!
What's our goal here?
We're going to build a simple pipeline that can take an image (or PDF), extract text from it using OCR, and then analyze that text using an LLM to get useful metadata. This could be handy for automatically categorizing documents, analyzing incoming correspondence, or building a smart document management system. We'll do it using some popular open-source tools and keep things relatively straightforward.
And yeah, everything below assumes you're already pretty comfortable with HF transformers. If not, check out https://huggingface.co/docs/transformers/en/quicktour - seems like a solid place to start. Though I never did and just learned from examples. I'll get to it... eventually.
What packages do we need?
We'll use torch and transformers for the heavy lifting, plus pymupdf and rich to make our lives easier with some user-friendly console output (I like the rich, so basically we're using it for fun).
import json import time import fitz import torch from transformers import AutoModel, AutoTokenizer, pipeline from rich.console import Console console = Console()
Prepare the image
First off, what image should we use as input? Since we're using Hugging Face here for the primary job, let's use the first page of their leading web page as our test subject. It's a good candidate with both text and complicated formatting - perfect for putting our OCR through its paces.
For a more realistic solution, let's assume our input is a PDF (because let's face it, that's what you'll probably deal with in the real world). We'll need to convert it to PNG format for our model to process:
INPUT_PDF_FILE = "./data/ocr_hf_main_page.pdf" OUTPUT_PNG_FILE = "./data/ocr_hf_main_page.png" doc = fitz.open(INPUT_PDF_FILE) page = doc.load_page(0) pixmap = page.get_pixmap(dpi=300) img = pixmap.tobytes() with console.status("Converting PDF to PNG...", spinner="monkey"): with open(OUTPUT_PNG_FILE, "wb") as f: f.write(img)
Do the real OCR here
I've played around with various OCR solutions for this task. Sure, there's tesseract and plenty of other options out there. But for my test case, I got the best results with GOT-OCR2_0 (https://huggingface.co/stepfun-ai/GOT-OCR2_0). So let's jump right in with that:
tokenizer = AutoTokenizer.from_pretrained( "ucaslcl/GOT-OCR2_0", device_map="cuda", trust_remote_code=True, ) model = AutoModel.from_pretrained( "ucaslcl/GOT-OCR2_0", trust_remote_code=True, low_cpu_mem_usage=True, use_safetensors=True, pad_token_id=tokenizer.eos_token_id, ) model = model.eval().cuda()
What's going on here? Well, default AutoModel and AutoTokenizer, the only special enough part is we're setting up the model to use cuda. And this isn't optional. The model requires CUDA support to run.
Now that we've defined our model, let's actually put it to work on our saved file. Also, we will measure the time and print it. Useful not only to compare with different models, but also to understand if it's even feasible for your use case to wait so long (although it's very quick for our case):
import json import time import fitz import torch from transformers import AutoModel, AutoTokenizer, pipeline from rich.console import Console console = Console()
And here's what we get from our original image:
INPUT_PDF_FILE = "./data/ocr_hf_main_page.pdf" OUTPUT_PNG_FILE = "./data/ocr_hf_main_page.png" doc = fitz.open(INPUT_PDF_FILE) page = doc.load_page(0) pixmap = page.get_pixmap(dpi=300) img = pixmap.tobytes() with console.status("Converting PDF to PNG...", spinner="monkey"): with open(OUTPUT_PNG_FILE, "wb") as f: f.write(img)
^ all the text, no formatting, but it's intentional.
GOT-OCR2_0 is pretty flexible - it can output in different formats, including HTML. Here are some other ways you can use it:
tokenizer = AutoTokenizer.from_pretrained( "ucaslcl/GOT-OCR2_0", device_map="cuda", trust_remote_code=True, ) model = AutoModel.from_pretrained( "ucaslcl/GOT-OCR2_0", trust_remote_code=True, low_cpu_mem_usage=True, use_safetensors=True, pad_token_id=tokenizer.eos_token_id, ) model = model.eval().cuda()
Finally try LLM
Now comes the fun part - picking an LLM. There's been endless discussion about which one's best, with articles everywhere you look. But let's keep it simple: what's the LLM everyone and their dog has heard of? Llama. So we'll use Llama-3.2-1B to process out text.
What can we get from the text? Think basic stuff like text classification, sentiment analysis, language detection, etc. Imagine you're building a system to automatically categorize uploaded documents or sort incoming faxes for a pharmacy.
I'll skip the deep dive into prompt engineering (that's a whole other article and I don't believe I will be writing any), but here's the basic idea:
def run_ocr_for_file(func: callable, text: str): start_time = time.time() res = func() final_time = time.time() - start_time console.rule(f"[bold red] {text} [/bold red]") console.print(res) console.rule(f"Time: {final_time} seconds") return res result_text = None with console.status( "Running OCR for the result file...", spinner="monkey", ): result_text = run_ocr_for_file( lambda: model.chat( tokenizer, OUTPUT_PNG_FILE, ocr_type="ocr", ), "plain texts OCR", )
By the way, am I doing something hilariously stupid here with prompt/content? Let me know. Pretty new to the "prompt engineering" and do not take it seriously enough yet.
The model sometimes wraps the result in markdown code blocks, so we need to handle that (if anyone knows a cleaner way, I'm all ears):
Hugging Face- The Al community building the future. https: / / hugging face. co/ Search models, datasets, users. . . Following 0 All Models Datasets Spaces Papers Collections Community Posts Up votes Likes New Follow your favorite Al creators Refresh List black- forest- labs· Advancing state- of- the- art image generation Follow stability a i· Sharing open- source image generation models Follow bria a i· Specializing in advanced image editing models Follow Trending last 7 days All Models Datasets Spaces deep see k- a i/ Deep Seek- V 3 Updated 3 days ago· 40 k· 877 deep see k- a i/ Deep Seek- V 3- Base Updated 3 days ago· 6.34 k· 1.06 k 2.39 k TRELLIS Q wen/ QV Q- 72 B- Preview 88888888888888888888 888888888888888888 301 Gemini Co der 1 of 3 2025-01-01,9:38 p. m
And here's what we typically get as output:
# format texts OCR: result_text = model.chat( tokenizer, image_file, ocr_type='format', ) # fine-grained OCR: result_text = model.chat( tokenizer, image_file, ocr_type='ocr', ocr_box='', ) # ... ocr_type='format', ocr_box='') # ... ocr_type='ocr', ocr_color='') # ... ocr_type='format', ocr_color='') # multi-crop OCR: # ... ocr_type='ocr') # ... ocr_type='format') # render the formatted OCR results: result_text = model.chat( tokenizer, image_file, ocr_type='format', render=True, save_render_file = './demo.html', )
To sum up
We've built a little pipeline that can take a PDF, extract its text using some pretty good OCR, and then analyze that text using an LLM to get useful metadata. Is it production-ready? Probably not. But it's a solid starting point if you're looking to build something similar. The cool thing is how we combined different open-source tools to create something useful - from PDF handling to OCR to LLM analysis.
You can easily extend this. Maybe add better error handling, support for multiple pages, or try different LLMs. Or maybe hook it up to a document management system. Hope you will. It might be a fun task.
Remember, this is just one way to do it - there are probably dozens of other approaches that might work better for your specific use case. But hopefully, this gives you a good starting point for your own experiments! Or a perfect place to teach me in the comments how it's done.
The above is the detailed content of Quick and Dirty Document Analysis: Combining GOT-OCR and LLama in Python. For more information, please follow other related articles on the PHP Chinese website!

Hot AI Tools

Undresser.AI Undress
AI-powered app for creating realistic nude photos

AI Clothes Remover
Online AI tool for removing clothes from photos.

Undress AI Tool
Undress images for free

Clothoff.io
AI clothes remover

AI Hentai Generator
Generate AI Hentai for free.

Hot Article

Hot Tools

Notepad++7.3.1
Easy-to-use and free code editor

SublimeText3 Chinese version
Chinese version, very easy to use

Zend Studio 13.0.1
Powerful PHP integrated development environment

Dreamweaver CS6
Visual web development tools

SublimeText3 Mac version
God-level code editing software (SublimeText3)

Hot Topics


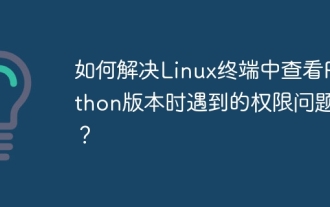
Solution to permission issues when viewing Python version in Linux terminal When you try to view Python version in Linux terminal, enter python...
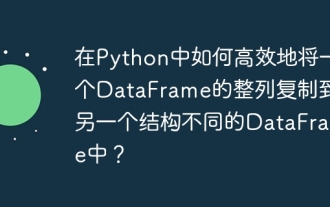
When using Python's pandas library, how to copy whole columns between two DataFrames with different structures is a common problem. Suppose we have two Dats...
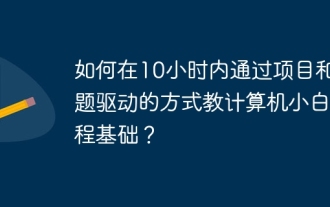
How to teach computer novice programming basics within 10 hours? If you only have 10 hours to teach computer novice some programming knowledge, what would you choose to teach...
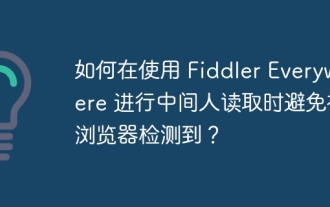
How to avoid being detected when using FiddlerEverywhere for man-in-the-middle readings When you use FiddlerEverywhere...
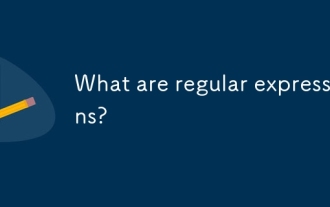
Regular expressions are powerful tools for pattern matching and text manipulation in programming, enhancing efficiency in text processing across various applications.
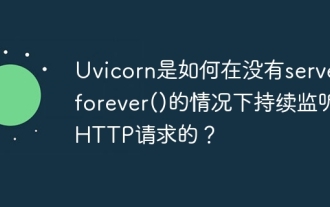
How does Uvicorn continuously listen for HTTP requests? Uvicorn is a lightweight web server based on ASGI. One of its core functions is to listen for HTTP requests and proceed...
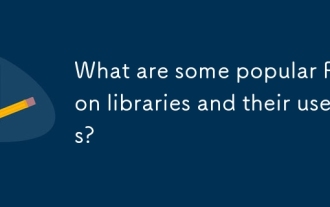
The article discusses popular Python libraries like NumPy, Pandas, Matplotlib, Scikit-learn, TensorFlow, Django, Flask, and Requests, detailing their uses in scientific computing, data analysis, visualization, machine learning, web development, and H
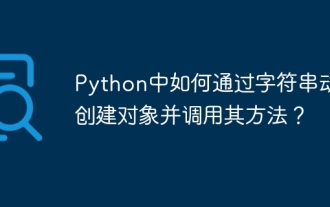
In Python, how to dynamically create an object through a string and call its methods? This is a common programming requirement, especially if it needs to be configured or run...
