Using proxy IP for data cleaning and preprocessing
Big data necessitates robust data cleaning and preprocessing. To ensure data accuracy and efficiency, data scientists employ various techniques. Using proxy IPs significantly enhances data acquisition efficiency and security. This article details how proxy IPs aid data cleaning and preprocessing, providing practical code examples.
I. The Crucial Role of Proxy IPs in Data Cleaning and Preprocessing
1.1 Overcoming Data Acquisition Barriers
Data acquisition is often the initial step. Many sources impose geographic or access frequency limitations. Proxy IPs, particularly high-quality services like 98IP proxy, bypass these restrictions, enabling access to diverse data sources.
1.2 Accelerated Data Acquisition
Proxy IPs distribute requests, preventing single IP blocks or rate limits from target websites. Rotating multiple proxies improves acquisition speed and stability.
1.3 Protecting Privacy and Security
Direct data acquisition exposes the user's real IP, risking privacy breaches. Proxy IPs mask the real IP, safeguarding privacy and mitigating malicious attacks.
II. Implementing Proxy IPs for Data Cleaning and Preprocessing
2.1 Selecting a Reliable Proxy IP Service
Choosing a dependable proxy provider is vital. 98IP Proxy, a professional provider, offers high-quality resources ideal for data cleaning and preprocessing.
2.2 Configuring Proxy IPs
Before data acquisition, configure the proxy IP within your code or tool. Here's a Python example using the requests
library:
import requests # Proxy IP address and port proxy = 'http://:<port number="">' # Target URL url = 'http://example.com/data' # Configuring Request Headers for Proxy IPs headers = { 'User-Agent': 'Mozilla/5.0 (Windows NT 10.0; Win64; x64) AppleWebKit/537.36 (KHTML, like Gecko) Chrome/58.0.3029.110 Safari/537.3'} # Send a GET request response = requests.get(url, headers=headers, proxies={'http': proxy, 'https': proxy}) # Output response content print(response.text)
2.3 Data Cleaning and Preprocessing Techniques
Post-acquisition, data cleaning and preprocessing are essential. This involves removing duplicates, handling missing values, type conversion, format standardization, and more. A simple example:
import pandas as pd # Data assumed fetched and saved as 'data.csv' df = pd.read_csv('data.csv') # Removing duplicates df = df.drop_duplicates() # Handling missing values (example: mean imputation) df = df.fillna(df.mean()) # Type conversion (assuming 'date_column' is a date) df['date_column'] = pd.to_datetime(df['date_column']) # Format standardization (lowercase strings) df['string_column'] = df['string_column'].str.lower() # Output cleaned data print(df.head())
2.4 Rotating Proxy IPs to Prevent Blocking
To avoid IP blocks from frequent requests, use a proxy IP pool and rotate them. A simple example:
import random import requests # Proxy IP pool proxy_pool = ['http://:<port number="">', 'http://:<port number="">', ...] # Target URL list urls = ['http://example.com/data1', 'http://example.com/data2', ...] # Send requests and retrieve data for url in urls: proxy = random.choice(proxy_pool) response = requests.get(url, headers=headers, proxies={'http': proxy, 'https': proxy}) # Process response content (e.g., save to file or database) # ...
III. Conclusion and Future Outlook
Proxy IPs are instrumental in efficient and secure data cleaning and preprocessing. They overcome acquisition limitations, accelerate data retrieval, and protect user privacy. By selecting suitable services, configuring proxies, cleaning data, and rotating IPs, you significantly enhance the process. As big data technology evolves, the application of proxy IPs will become even more prevalent. This article provides valuable insights into effectively utilizing proxy IPs for data cleaning and preprocessing.
The above is the detailed content of Using proxy IP for data cleaning and preprocessing. For more information, please follow other related articles on the PHP Chinese website!

Hot AI Tools

Undresser.AI Undress
AI-powered app for creating realistic nude photos

AI Clothes Remover
Online AI tool for removing clothes from photos.

Undress AI Tool
Undress images for free

Clothoff.io
AI clothes remover

AI Hentai Generator
Generate AI Hentai for free.

Hot Article

Hot Tools

Notepad++7.3.1
Easy-to-use and free code editor

SublimeText3 Chinese version
Chinese version, very easy to use

Zend Studio 13.0.1
Powerful PHP integrated development environment

Dreamweaver CS6
Visual web development tools

SublimeText3 Mac version
God-level code editing software (SublimeText3)

Hot Topics


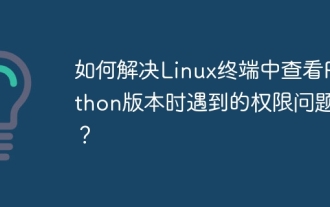
Solution to permission issues when viewing Python version in Linux terminal When you try to view Python version in Linux terminal, enter python...
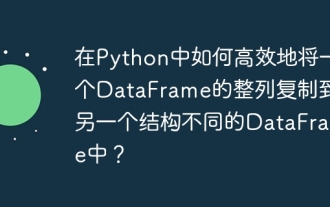
When using Python's pandas library, how to copy whole columns between two DataFrames with different structures is a common problem. Suppose we have two Dats...
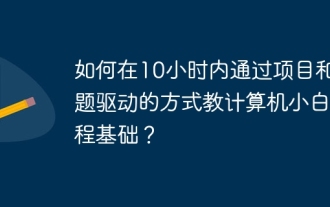
How to teach computer novice programming basics within 10 hours? If you only have 10 hours to teach computer novice some programming knowledge, what would you choose to teach...
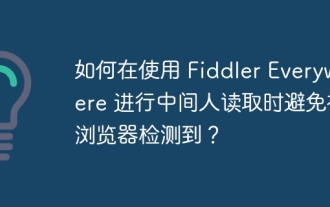
How to avoid being detected when using FiddlerEverywhere for man-in-the-middle readings When you use FiddlerEverywhere...
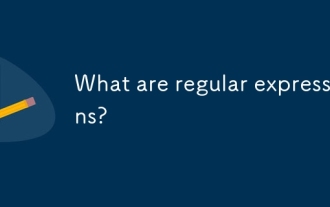
Regular expressions are powerful tools for pattern matching and text manipulation in programming, enhancing efficiency in text processing across various applications.
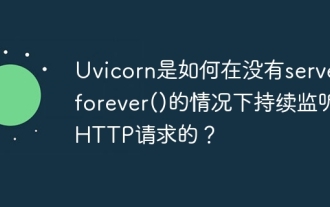
How does Uvicorn continuously listen for HTTP requests? Uvicorn is a lightweight web server based on ASGI. One of its core functions is to listen for HTTP requests and proceed...
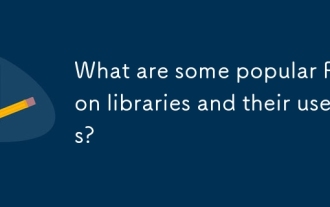
The article discusses popular Python libraries like NumPy, Pandas, Matplotlib, Scikit-learn, TensorFlow, Django, Flask, and Requests, detailing their uses in scientific computing, data analysis, visualization, machine learning, web development, and H
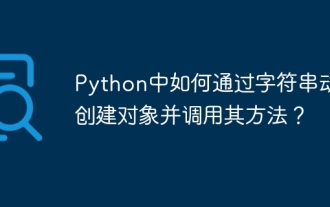
In Python, how to dynamically create an object through a string and call its methods? This is a common programming requirement, especially if it needs to be configured or run...
