Building an AI Sales Agent: From Voice to Pitch.
Project Overview
In the EnCode 2025 challenge, my goal is to create an AI sales agent capable of high-quality, natural and smooth voice interaction, and strive to achieve ultra-low latency, like an experience like talking to a real person. Ultimately, I built a system that could handle a complete sales conversation for an online coaching center, from greeting a potential customer to understanding their needs and recommending relevant courses, all in a positive, friendly, human-like voice. Imagine a salesperson who is tireless and always looking her best!
Technology stack
- Voice Processing: Whisper Large V3 Turbo (ensures clear speech recognition)
- Core logic: LLaMA 3.3 70B (realizing intelligent dialogue)
- Voice output: F5 TTS (generates natural and smooth voice responses)
- Database: Pinecone vector database (for context management and information retrieval)
- Demo platform: Google Colab
How the system works
The system follows three main steps:
- Speech to text (STT)
- Large Scale Language Model (LLM)
- Text to Speech (TTS)
Flowchart: User -> STT -> LLM -> TTS -> User
Detailed process:
- Customer Speaks -> Whisper transcribes the text.
- Phase manager (using regular expressions) tracks conversation phases.
- Pinecone extracts relevant data from the database.
- LLaMA 3.3 70B Build the perfect reply.
- F5 TTS converts text into natural speech.
Main functions
- Intelligent voice selection: Provides 6 different AI voices (2 male and 4 female)
- Context-aware reply: Based on vector similarity search technology
- Structured dialogue flow: Controlled by a dedicated stage manager
Current limitations
- Demo environment: Running based on Google Colab.
- Memory Limit: Context window limit of 8k tokens.
- Computing resource consumption: The resource usage is large.
- API Dependencies: Core functionality depends on multiple APIs.
- High latency: There is a certain latency problem.
Experience summary
Technical aspects:
- Application of vector database: Using Pinecone vector database allowed me to realize how vector database can change the rules of the game when the context window is limited. The millisecond-level similarity search function can effectively process conversation history and training data, and is very powerful.
- Importance of Stage Management: By making the conversation stage clear, you can easily incorporate examples relevant to that stage, such as how to pitch, what questions to ask, etc.
- Web Integration: Using fastapi for efficient front-end and back-end data interaction is crucial. With webhooks, we are able to exchange data throughout the conversation and stay connected while only initiating an AI call once.
System design:
- Importance of Chunking:Breaking audio into 5-second long segments for processing instead of waiting for complete sentences significantly improves the user experience and reduces processing time. This requires finding the best balance between accuracy and speed.
- Advantages of modular architecture: Decomposing the system into independent services (STT, LLM, TTS) greatly simplifies the development and debugging process. When a problem occurs, you can quickly locate the part that needs to be fixed.
Actual limitations:
- API Cost: Managing multiple API calls (Whisper, LLAMA) taught me the importance of optimizing API usage. Minimizing the number of API calls while maintaining speed is a big challenge.
- Reducing latency: Reducing latency is very difficult when data is constantly being fetched and processed from the internet. In the future, I will try to minimize the number of times I transfer or download data from the internet.
Unexpected challenges:
- Prompt word engineering: Prompt word engineering is crucial, it determines whether the model can express coherently like a human, or whether it will repeat the same sentences.
- Context Window Limitation: The 8k token limit forces me to manage context smartly. Instead of storing all the information, getting the relevant pieces from a vector database allowed me to design a structure for the LLM that contained all the necessary information.
Future Plans
- Use multi-threading technology to reduce latency.
- Added multi-language support.
- Add more types of bots, such as "lead bots" to contact customers after an initial lead to close a deal.
Experience Project
https://www.php.cn/link/55e2c9d06a7261846e96b8bb2d4e1fe5
GitHub ---
Welcome to put forward your valuable suggestions in the comment area!
The above is the detailed content of Building an AI Sales Agent: From Voice to Pitch.. For more information, please follow other related articles on the PHP Chinese website!

Hot AI Tools

Undresser.AI Undress
AI-powered app for creating realistic nude photos

AI Clothes Remover
Online AI tool for removing clothes from photos.

Undress AI Tool
Undress images for free

Clothoff.io
AI clothes remover

Video Face Swap
Swap faces in any video effortlessly with our completely free AI face swap tool!

Hot Article

Hot Tools

Notepad++7.3.1
Easy-to-use and free code editor

SublimeText3 Chinese version
Chinese version, very easy to use

Zend Studio 13.0.1
Powerful PHP integrated development environment

Dreamweaver CS6
Visual web development tools

SublimeText3 Mac version
God-level code editing software (SublimeText3)

Hot Topics




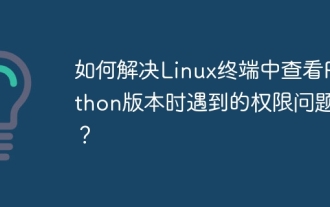
Solution to permission issues when viewing Python version in Linux terminal When you try to view Python version in Linux terminal, enter python...
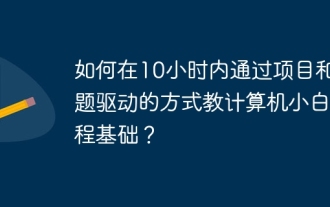
How to teach computer novice programming basics within 10 hours? If you only have 10 hours to teach computer novice some programming knowledge, what would you choose to teach...
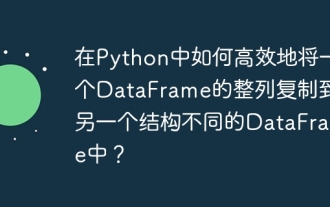
When using Python's pandas library, how to copy whole columns between two DataFrames with different structures is a common problem. Suppose we have two Dats...
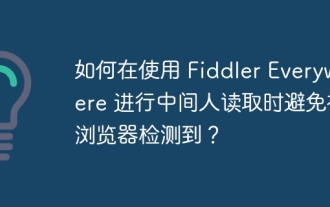
How to avoid being detected when using FiddlerEverywhere for man-in-the-middle readings When you use FiddlerEverywhere...
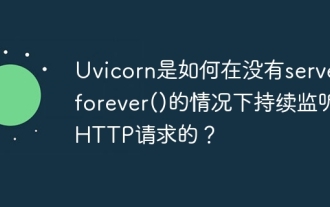
How does Uvicorn continuously listen for HTTP requests? Uvicorn is a lightweight web server based on ASGI. One of its core functions is to listen for HTTP requests and proceed...
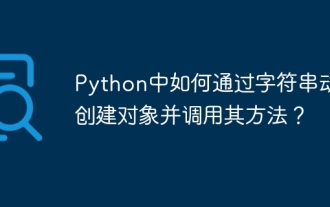
In Python, how to dynamically create an object through a string and call its methods? This is a common programming requirement, especially if it needs to be configured or run...
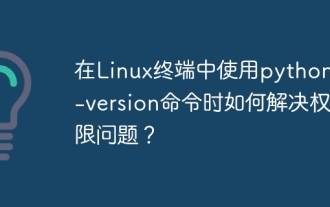
Using python in Linux terminal...
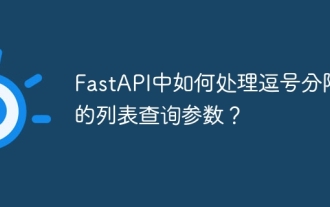
Fastapi ...
