


How Do I Query Complex Data Types (Arrays, Maps, Structs) in Spark SQL DataFrames?
Accessing Complex Data in Spark SQL DataFrames
Spark SQL supports complex data types like arrays and maps. However, querying these requires specific approaches. This guide details how to effectively query these structures:
Arrays:
Several methods exist for accessing array elements:
-
getItem
method: This DataFrame API method directly accesses elements by index.df.select($"an_array".getItem(1)).show
Copy after login -
Hive bracket syntax: This SQL-like syntax offers an alternative.
SELECT an_array[1] FROM df
Copy after login -
User-Defined Functions (UDFs): UDFs provide flexibility for more complex array manipulations.
val get_ith = udf((xs: Seq[Int], i: Int) => Try(xs(i)).toOption) df.select(get_ith($"an_array", lit(1))).show
Copy after login -
Built-in functions: Spark offers built-in functions like
transform
,filter
,aggregate
, and thearray_*
family for array processing.
Maps:
Accessing map values involves similar techniques:
-
getField
method: Retrieves values using the key.df.select($"a_map".getField("foo")).show
Copy after login -
Hive bracket syntax: Provides a SQL-like approach.
SELECT a_map['foo'] FROM df
Copy after login -
Dot syntax: A concise way to access map fields.
df.select($"a_map.foo").show
Copy after login -
UDFs: For customized map operations.
val get_field = udf((kvs: Map[String, String], k: String) => kvs.get(k)) df.select(get_field($"a_map", lit("foo"))).show
Copy after login -
*`map_
functions:** Functions like
map_keysand
map_values` are available for map manipulation.
Structs:
Accessing struct fields is straightforward:
-
Dot syntax: The most direct method.
df.select($"a_struct.x").show
Copy after login -
Raw SQL: An alternative using SQL syntax.
SELECT a_struct.x FROM df
Copy after login
Arrays of Structs:
Querying nested structures requires combining the above techniques:
-
Nested dot syntax: Access fields within structs within arrays.
df.select($"an_array_of_structs.foo").show
Copy after login -
Combined methods: Using
getItem
to access array elements and then dot syntax for struct fields.df.select($"an_array_of_structs.vals".getItem(1).getItem(1)).show
Copy after login
User-Defined Types (UDTs):
UDTs are typically accessed using UDFs.
Important Considerations:
-
Context: Some methods might only work with
HiveContext
, depending on your Spark version. - Nested Field Support: Not all operations support deeply nested fields.
- Efficiency: Schema flattening or collection explosion might improve performance for complex queries.
-
Wildcard: The wildcard character (
*
) can be used with dot syntax to select multiple fields.
This guide provides a comprehensive overview of querying complex data types in Spark SQL DataFrames. Remember to choose the method best suited for your specific needs and data structure.
The above is the detailed content of How Do I Query Complex Data Types (Arrays, Maps, Structs) in Spark SQL DataFrames?. For more information, please follow other related articles on the PHP Chinese website!

Hot AI Tools

Undresser.AI Undress
AI-powered app for creating realistic nude photos

AI Clothes Remover
Online AI tool for removing clothes from photos.

Undress AI Tool
Undress images for free

Clothoff.io
AI clothes remover

Video Face Swap
Swap faces in any video effortlessly with our completely free AI face swap tool!

Hot Article

Hot Tools

Notepad++7.3.1
Easy-to-use and free code editor

SublimeText3 Chinese version
Chinese version, very easy to use

Zend Studio 13.0.1
Powerful PHP integrated development environment

Dreamweaver CS6
Visual web development tools

SublimeText3 Mac version
God-level code editing software (SublimeText3)

Hot Topics
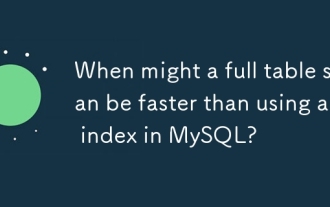
Full table scanning may be faster in MySQL than using indexes. Specific cases include: 1) the data volume is small; 2) when the query returns a large amount of data; 3) when the index column is not highly selective; 4) when the complex query. By analyzing query plans, optimizing indexes, avoiding over-index and regularly maintaining tables, you can make the best choices in practical applications.
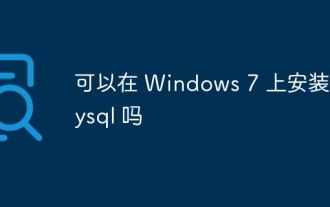
Yes, MySQL can be installed on Windows 7, and although Microsoft has stopped supporting Windows 7, MySQL is still compatible with it. However, the following points should be noted during the installation process: Download the MySQL installer for Windows. Select the appropriate version of MySQL (community or enterprise). Select the appropriate installation directory and character set during the installation process. Set the root user password and keep it properly. Connect to the database for testing. Note the compatibility and security issues on Windows 7, and it is recommended to upgrade to a supported operating system.
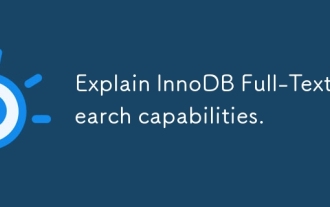
InnoDB's full-text search capabilities are very powerful, which can significantly improve database query efficiency and ability to process large amounts of text data. 1) InnoDB implements full-text search through inverted indexing, supporting basic and advanced search queries. 2) Use MATCH and AGAINST keywords to search, support Boolean mode and phrase search. 3) Optimization methods include using word segmentation technology, periodic rebuilding of indexes and adjusting cache size to improve performance and accuracy.
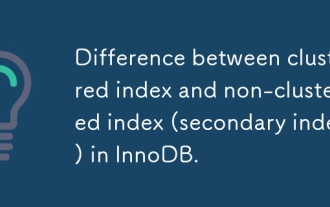
The difference between clustered index and non-clustered index is: 1. Clustered index stores data rows in the index structure, which is suitable for querying by primary key and range. 2. The non-clustered index stores index key values and pointers to data rows, and is suitable for non-primary key column queries.
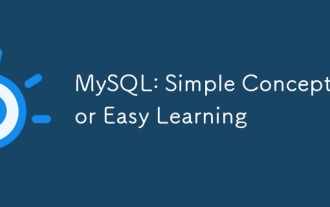
MySQL is an open source relational database management system. 1) Create database and tables: Use the CREATEDATABASE and CREATETABLE commands. 2) Basic operations: INSERT, UPDATE, DELETE and SELECT. 3) Advanced operations: JOIN, subquery and transaction processing. 4) Debugging skills: Check syntax, data type and permissions. 5) Optimization suggestions: Use indexes, avoid SELECT* and use transactions.
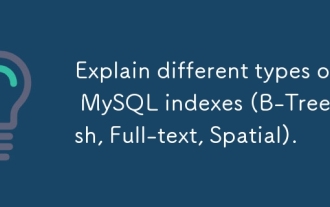
MySQL supports four index types: B-Tree, Hash, Full-text, and Spatial. 1.B-Tree index is suitable for equal value search, range query and sorting. 2. Hash index is suitable for equal value searches, but does not support range query and sorting. 3. Full-text index is used for full-text search and is suitable for processing large amounts of text data. 4. Spatial index is used for geospatial data query and is suitable for GIS applications.
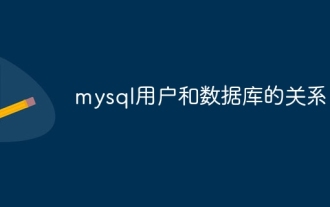
In MySQL database, the relationship between the user and the database is defined by permissions and tables. The user has a username and password to access the database. Permissions are granted through the GRANT command, while the table is created by the CREATE TABLE command. To establish a relationship between a user and a database, you need to create a database, create a user, and then grant permissions.
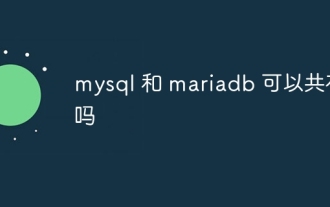
MySQL and MariaDB can coexist, but need to be configured with caution. The key is to allocate different port numbers and data directories to each database, and adjust parameters such as memory allocation and cache size. Connection pooling, application configuration, and version differences also need to be considered and need to be carefully tested and planned to avoid pitfalls. Running two databases simultaneously can cause performance problems in situations where resources are limited.
