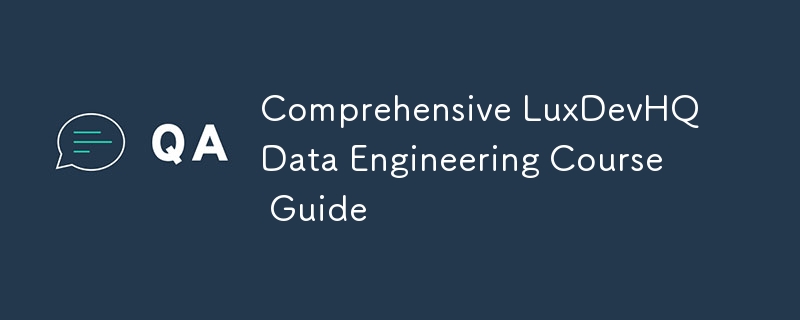
This intensive 16-week (4-month) data engineering bootcamp provides comprehensive training in Python, SQL, cloud platforms (Azure and AWS), Apache Airflow, Kafka, Spark, and more.
Schedule:
-
Monday - Thursday: Lectures and hands-on exercises.
-
Friday: Industry mentorship or collaborative peer projects.
-
Saturday: Dedicated lab sessions and project-based learning.
Module 1: Data Engineering Fundamentals (Weeks 1-4)
Week 1: Onboarding & Setup
-
Monday: Welcome, course overview, career paths, tool introductions.
-
Tuesday: Cloud computing overview (Azure & AWS).
-
Wednesday: Data governance, security, and compliance.
-
Thursday: SQL fundamentals and PostgreSQL setup.
-
Friday: Peer project: Environment setup challenges.
-
Saturday (Lab): Mini-project: Basic pipeline using PostgreSQL and Azure Blob Storage.
Week 2: Mastering SQL
-
Monday: Core SQL (SELECT, WHERE, JOIN, GROUP BY).
-
Tuesday: Advanced SQL (recursive queries, window functions, CTEs).
-
Wednesday: Query optimization and execution plans.
-
Thursday: Data modeling (normalization, denormalization, star schemas).
-
Friday: Job shadowing: Observing SQL query optimization techniques.
-
Saturday (Lab): Mini-project: Star schema design and SQL-based data analysis.
Week 3: Data Pipeline Introduction
-
Monday: ETL/ELT workflow theory.
-
Tuesday: Lab: Python-based ETL pipeline for CSV data.
-
Wednesday: ETL best practices.
-
Thursday: Lab: Python ETL pipeline for batch data processing.
-
Friday: Peer project: Collaborative ETL workflow design.
-
Saturday (Lab): Mini-project: ETL pipeline for sales data processing.
Week 4: Apache Airflow Fundamentals
-
Monday: Introduction to Apache Airflow, DAGs, and scheduling.
-
Tuesday: Lab: Setting up and creating a basic Airflow DAG.
-
Wednesday: Airflow DAG best practices and scheduling.
-
Thursday: Lab: Integrating Airflow with PostgreSQL and Azure Blob Storage.
-
Friday: Job shadowing: Real-world Airflow pipeline observation.
-
Saturday (Lab): Mini-project: Automating an ETL pipeline with Airflow.
Module 2: Intermediate Skills (Weeks 5-8)
Week 5: Data Warehousing & Lakes
-
Monday: Data warehousing (OLAP vs. OLTP, partitioning, clustering).
-
Tuesday: Lab: Working with Amazon Redshift and Snowflake.
-
Wednesday: Data lakes and Lakehouse architecture.
-
Thursday: Lab: Setting up Delta Lake.
-
Friday: Peer project: Implementing a data warehouse and data lake model.
-
Saturday (Lab): Mini-project: Designing and implementing a basic Lakehouse architecture.
Week 6: Data Governance & Security
-
Monday: Data governance frameworks and security principles.
-
Tuesday: Lab: Using AWS Lake Formation for access control.
-
Wednesday: Managing sensitive data and compliance (GDPR, HIPAA).
-
Thursday: Lab: Implementing security policies in S3 and Azure Blob Storage.
-
Friday: Job shadowing: Observing the application of governance policies.
-
Saturday (Lab): Mini-project: Securing cloud data using AWS and Azure.
Week 7: Real-Time Data with Kafka
-
Monday: Introduction to Apache Kafka for real-time data streaming.
-
Tuesday: Lab: Setting up a Kafka producer and consumer.
-
Wednesday: Kafka topics, partitions, and message brokers.
-
Thursday: Lab: Integrating Kafka with PostgreSQL for real-time updates.
-
Friday: Peer project: Building a real-time Kafka pipeline.
-
Saturday (Lab): Mini-project: Streaming e-commerce data with Kafka.
Week 8: Batch vs. Stream Processing
-
Monday: Batch vs. stream processing comparison.
-
Tuesday: Lab: Batch processing with PySpark.
-
Wednesday: Combining batch and stream processing workflows.
-
Thursday: Lab: Real-time processing with Apache Flink and Spark Streaming.
-
Friday: Job shadowing: Observing a real-time processing pipeline.
-
Saturday (Lab): Mini-project: Building a hybrid batch/real-time pipeline.
Module 3: Advanced Data Engineering (Weeks 9-12)
Week 9: ML Integration in Data Pipelines
-
Monday: Overview of ML workflows in data engineering.
-
Tuesday: Lab: Data preprocessing for ML using Pandas and PySpark.
-
Wednesday: Feature engineering and automated feature extraction.
-
Thursday: Lab: Automating feature extraction using Apache Airflow.
-
Friday: Peer project: Building a pipeline integrating ML models.
-
Saturday (Lab): Mini-project: Building an ML-powered recommendation system.
Week 10: Spark & PySpark for Big Data
-
Monday: Introduction to Apache Spark.
-
Tuesday: Lab: Setting up Spark and PySpark.
-
Wednesday: Spark RDDs, DataFrames, and SQL.
-
Thursday: Lab: Analyzing large datasets using Spark SQL.
-
Friday: Peer project: Building a PySpark pipeline for large-scale data processing.
-
Saturday (Lab): Mini-project: Analyzing big datasets with Spark and PySpark.
Week 11: Advanced Apache Airflow
-
Monday: Advanced Airflow features (XCom, task dependencies).
-
Tuesday: Lab: Implementing dynamic DAGs and task dependencies.
-
Wednesday: Airflow scheduling, monitoring, and error handling.
-
Thursday: Lab: Creating complex DAGs for multi-step ETL pipelines.
-
Friday: Job shadowing: Observing advanced Airflow pipeline implementations.
-
Saturday (Lab): Mini-project: Designing an advanced Airflow DAG.
Week 12: Data Lakes & Delta Lake
-
Monday: Data lakes, Lakehouses, and Delta Lake architecture.
-
Tuesday: Lab: Setting up Delta Lake on AWS.
-
Wednesday: Managing schema evolution in Delta Lake.
-
Thursday: Lab: Implementing batch and real-time data loading to Delta Lake.
-
Friday: Peer project: Designing a Lakehouse architecture.
-
Saturday (Lab): Mini-project: Implementing a scalable Delta Lake architecture.
Module 4: Capstone Projects (Weeks 13-16)
Weeks 13-16: Capstone Project Development & Presentation
These weeks focus on developing and presenting two major capstone projects: a batch data pipeline (e-commerce sales analytics) and a real-time data pipeline (IoT sensor monitoring), culminating in an integrated solution showcasing both. The final week involves project presentations to industry professionals and instructors.
The above is the detailed content of Comprehensive LuxDevHQ Data Engineering Course Guide. For more information, please follow other related articles on the PHP Chinese website!