


Building an NBA Stats Pipeline with AWS, Python, and DynamoDB
This tutorial details the creation of an automated NBA statistics data pipeline using AWS services, Python, and DynamoDB. Whether you're a sports data enthusiast or an AWS learner, this hands-on project provides valuable experience in real-world data processing.
Project Overview
This pipeline automatically retrieves NBA statistics from the SportsData API, processes the data, and stores it in DynamoDB. The AWS services used include:
- DynamoDB: Data storage
- Lambda: Serverless execution
- CloudWatch: Monitoring and logging
Prerequisites
Before starting, ensure you have:
- Basic Python skills
- An AWS account
- The AWS CLI installed and configured
- A SportsData API key
Project Setup
Clone the repository and install dependencies:
git clone https://github.com/nolunchbreaks/nba-stats-pipeline.git cd nba-stats-pipeline pip install -r requirements.txt
Environment Configuration
Create a .env
file in the project root with these variables:
<code>SPORTDATA_API_KEY=your_api_key_here AWS_REGION=us-east-1 DYNAMODB_TABLE_NAME=nba-player-stats</code>
Project Structure
The project's directory structure is as follows:
<code>nba-stats-pipeline/ ├── src/ │ ├── __init__.py │ ├── nba_stats.py │ └── lambda_function.py ├── tests/ ├── requirements.txt ├── README.md └── .env</code>
Data Storage and Structure
DynamoDB Schema
The pipeline stores NBA team statistics in DynamoDB using this schema:
- Partition Key: TeamID
- Sort Key: Timestamp
- Attributes: Team statistics (win/loss, points per game, conference standings, division rankings, historical metrics)
AWS Infrastructure
DynamoDB Table Configuration
Configure the DynamoDB table as follows:
- Table Name:
nba-player-stats
- Primary Key:
TeamID
(String) - Sort Key:
Timestamp
(Number) - Provisioned Capacity: Adjust as needed
Lambda Function Configuration (if using Lambda)
- Runtime: Python 3.9
- Memory: 256MB
- Timeout: 30 seconds
- Handler:
lambda_function.lambda_handler
Error Handling and Monitoring
The pipeline includes robust error handling for API failures, DynamoDB throttling, data transformation issues, and invalid API responses. CloudWatch logs all events in structured JSON for performance monitoring, debugging, and ensuring successful data processing.
Resource Cleanup
After completing the project, clean up AWS resources:
git clone https://github.com/nolunchbreaks/nba-stats-pipeline.git cd nba-stats-pipeline pip install -r requirements.txt
Key Takeaways
This project highlighted:
- AWS Service Integration: Effective use of multiple AWS services for a cohesive data pipeline.
- Error Handling: The importance of thorough error handling in production environments.
- Monitoring: Essential role of logging and monitoring in maintaining data pipelines.
- Cost Management: Awareness of AWS resource usage and cleanup.
Future Enhancements
Possible project extensions include:
- Real-time game statistics integration
- Data visualization implementation
- API endpoints for data access
- Advanced data analysis capabilities
Conclusion
This NBA statistics pipeline demonstrates the power of combining AWS services and Python for building functional data pipelines. It's a valuable resource for those interested in sports analytics or AWS data processing. Share your experiences and suggestions for improvement!
Follow for more AWS and Python tutorials! Appreciate a ❤️ and a ? if you found this helpful!
The above is the detailed content of Building an NBA Stats Pipeline with AWS, Python, and DynamoDB. For more information, please follow other related articles on the PHP Chinese website!

Hot AI Tools

Undresser.AI Undress
AI-powered app for creating realistic nude photos

AI Clothes Remover
Online AI tool for removing clothes from photos.

Undress AI Tool
Undress images for free

Clothoff.io
AI clothes remover

Video Face Swap
Swap faces in any video effortlessly with our completely free AI face swap tool!

Hot Article

Hot Tools

Notepad++7.3.1
Easy-to-use and free code editor

SublimeText3 Chinese version
Chinese version, very easy to use

Zend Studio 13.0.1
Powerful PHP integrated development environment

Dreamweaver CS6
Visual web development tools

SublimeText3 Mac version
God-level code editing software (SublimeText3)

Hot Topics
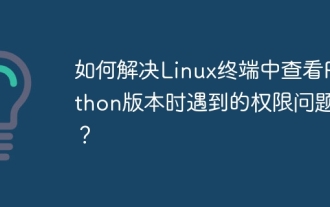
Solution to permission issues when viewing Python version in Linux terminal When you try to view Python version in Linux terminal, enter python...
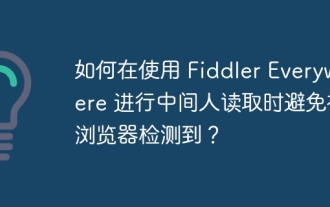
How to avoid being detected when using FiddlerEverywhere for man-in-the-middle readings When you use FiddlerEverywhere...
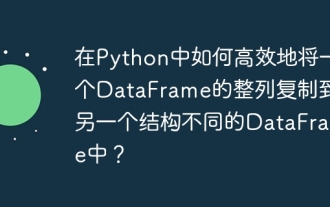
When using Python's pandas library, how to copy whole columns between two DataFrames with different structures is a common problem. Suppose we have two Dats...
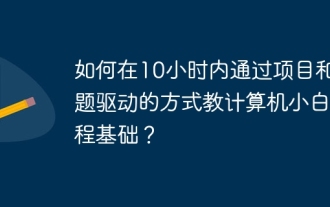
How to teach computer novice programming basics within 10 hours? If you only have 10 hours to teach computer novice some programming knowledge, what would you choose to teach...
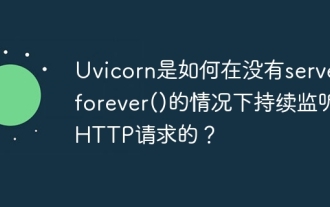
How does Uvicorn continuously listen for HTTP requests? Uvicorn is a lightweight web server based on ASGI. One of its core functions is to listen for HTTP requests and proceed...
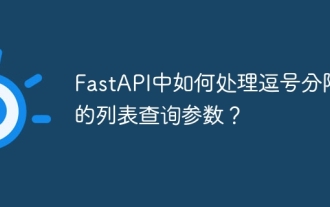
Fastapi ...
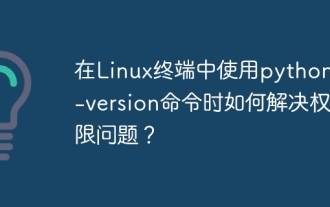
Using python in Linux terminal...
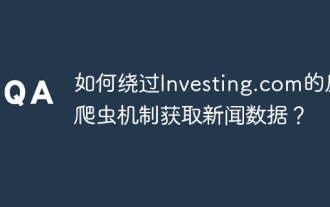
Understanding the anti-crawling strategy of Investing.com Many people often try to crawl news data from Investing.com (https://cn.investing.com/news/latest-news)...
