Data Preprocessing: Exploring the Keys to Data Preparation
This article explores data preprocessing: its importance, and how to clean, transform, integrate, and reduce data.
Key Concepts:
Data preprocessing is crucial for data analysis and machine learning. It transforms raw data into a structured format for effective analysis and modeling. This involves several key techniques:
- Data Cleaning: Addresses inaccuracies and inconsistencies. This includes handling missing values (through removal or imputation), removing duplicates, and managing outliers.
- Data Transformation: Modifies data to improve its suitability for analysis. Methods include log and square root transformations to normalize distributions and mitigate outlier effects.
- Data Integration: Consolidates data from multiple sources into a unified dataset, enhancing completeness and resolving inconsistencies.
- Data Reduction: Streamlines data by removing redundancy and focusing on essential features, improving processing efficiency. Techniques include aggregation and dimensionality reduction.
The ultimate goal is to enhance data quality and reliability, directly impacting machine learning model performance and the accuracy of data-driven decisions.
Why is Data Preprocessing Necessary?
Real-world data is often imperfect. Raw data frequently contains missing values, outliers, inconsistencies, and noise. These imperfections hinder analysis, compromising the reliability and accuracy of results. Data from diverse sources may also differ in scale, units, and format, making direct comparison difficult. Preprocessing addresses these challenges.
Data Cleaning Techniques:
- Handling Missing Values: Methods include removing rows/columns with missing data (dropna), imputing missing values using statistical measures (mean, median, mode) (fillna), or employing machine learning algorithms (e.g., KNNImputer) for more sophisticated imputation.
-
Handling Duplicates: Identifying and removing duplicate rows using functions like
duplicated()
anddrop_duplicates()
. - Handling Outliers: Identifying outliers using techniques like z-scores or the interquartile range (IQR). Outliers can be removed or the data can be transformed (e.g., log transformation, square root transformation) to lessen their impact.
Data Transformation Methods:
Log and square root transformations are commonly used to normalize data distribution and reduce the influence of outliers.
Data Integration Strategies:
Combining data from multiple sources (e.g., using pd.merge()
in Pandas) creates a comprehensive dataset for analysis. Careful consideration of key identifiers (e.g., customer IDs) is crucial for accurate merging.
Data Reduction Approaches:
Techniques like data cube aggregation, dimensionality reduction, data compression, and numerosity reduction help manage large datasets while preserving essential information.
Conclusion:
Effective data preprocessing is analogous to preparing ingredients for a recipe. Just as careful preparation leads to a better dish, meticulous data preprocessing results in more accurate and reliable data analysis and machine learning model performance. Thorough data exploration and understanding of data patterns are essential before selecting preprocessing techniques. Validation and testing are crucial for evaluating the effectiveness of different methods.
The above is the detailed content of Data Preprocessing: Exploring the Keys to Data Preparation. For more information, please follow other related articles on the PHP Chinese website!

Hot AI Tools

Undresser.AI Undress
AI-powered app for creating realistic nude photos

AI Clothes Remover
Online AI tool for removing clothes from photos.

Undress AI Tool
Undress images for free

Clothoff.io
AI clothes remover

Video Face Swap
Swap faces in any video effortlessly with our completely free AI face swap tool!

Hot Article

Hot Tools

Notepad++7.3.1
Easy-to-use and free code editor

SublimeText3 Chinese version
Chinese version, very easy to use

Zend Studio 13.0.1
Powerful PHP integrated development environment

Dreamweaver CS6
Visual web development tools

SublimeText3 Mac version
God-level code editing software (SublimeText3)

Hot Topics
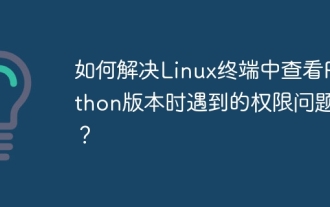
Solution to permission issues when viewing Python version in Linux terminal When you try to view Python version in Linux terminal, enter python...
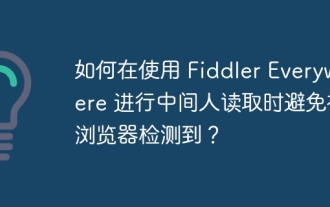
How to avoid being detected when using FiddlerEverywhere for man-in-the-middle readings When you use FiddlerEverywhere...
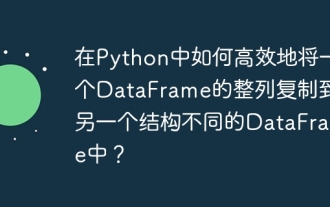
When using Python's pandas library, how to copy whole columns between two DataFrames with different structures is a common problem. Suppose we have two Dats...
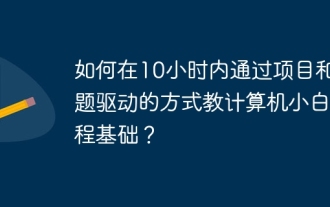
How to teach computer novice programming basics within 10 hours? If you only have 10 hours to teach computer novice some programming knowledge, what would you choose to teach...
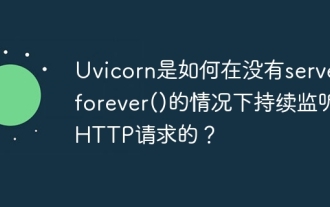
How does Uvicorn continuously listen for HTTP requests? Uvicorn is a lightweight web server based on ASGI. One of its core functions is to listen for HTTP requests and proceed...
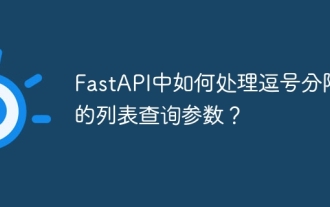
Fastapi ...
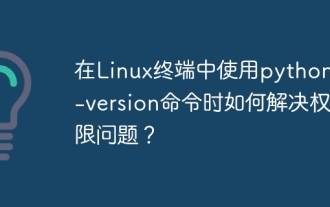
Using python in Linux terminal...
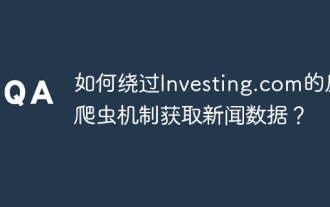
Understanding the anti-crawling strategy of Investing.com Many people often try to crawl news data from Investing.com (https://cn.investing.com/news/latest-news)...
