Training Language Models on Google Colab
Fine-tuning large language models (LLMs) like BERT, Llama, BART, and those from Mistral AI and others can be computationally intensive. Lacking a local GPU, Google Colab provides a free alternative, but its transient nature presents challenges in preserving your progress. This guide demonstrates how to leverage Google Drive to overcome this limitation, enabling you to save and resume your LLM training across multiple Colab sessions.
The solution involves using Google Drive to store intermediate results and model checkpoints. This ensures your work persists even after the Colab environment is reset. You'll need a Google account with sufficient Drive space. Create two folders in your Drive: "data" (for your training dataset) and "checkpoints" (to store model checkpoints).
Mounting Google Drive in Colab:
Begin by mounting your Google Drive within your Colab notebook using this command:
from google.colab import drive drive.mount('/content/drive')
Verify access by listing the contents of your data and checkpoints directories:
!ls /content/drive/MyDrive/data !ls /content/drive/MyDrive/checkpoints
If authorization is required, a pop-up window will appear. Ensure you grant the necessary access permissions. If the commands fail, re-run the mounting cell and check your permissions.
Saving and Loading Checkpoints:
The core of the solution lies in creating functions to save and load model checkpoints. These functions will serialize your model's state, optimizer, scheduler, and other relevant information to your "checkpoints" folder.
Save Checkpoint Function:
import torch import os def save_checkpoint(epoch, model, optimizer, scheduler, loss, model_name, overwrite=True): checkpoint = { 'epoch': epoch, 'model_state_dict': model.state_dict(), 'optimizer_state_dict': optimizer.state_dict(), 'scheduler_state_dict': scheduler.state_dict(), 'loss': loss } direc = get_checkpoint_dir(model_name) #Assumed function to construct directory path if overwrite: file_path = os.path.join(direc, 'checkpoint.pth') else: file_path = os.path.join(direc, f'epoch_{epoch}_checkpoint.pth') os.makedirs(direc, exist_ok=True) # Create directory if it doesn't exist torch.save(checkpoint, file_path) print(f"Checkpoint saved at epoch {epoch}") #Example get_checkpoint_dir function (adapt to your needs) def get_checkpoint_dir(model_name): return os.path.join("/content/drive/MyDrive/checkpoints", model_name)
Load Checkpoint Function:
import torch import os def load_checkpoint(model_name, model, optimizer, scheduler): direc = get_checkpoint_dir(model_name) if os.path.exists(direc): #Find checkpoint with highest epoch (adapt to your naming convention) checkpoints = [f for f in os.listdir(direc) if f.endswith('.pth')] if checkpoints: latest_checkpoint = max(checkpoints, key=lambda x: int(x.split('_')[-2]) if '_' in x else 0) file_path = os.path.join(direc, latest_checkpoint) checkpoint = torch.load(file_path, map_location=torch.device('cpu')) model.load_state_dict(checkpoint['model_state_dict']) optimizer.load_state_dict(checkpoint['optimizer_state_dict']) scheduler.load_state_dict(checkpoint['scheduler_state_dict']) epoch = checkpoint['epoch'] loss = checkpoint['loss'] print(f"Checkpoint loaded from epoch {epoch}") return epoch, loss else: print("No checkpoints found in directory.") return 0, None else: print(f"No checkpoint directory found for {model_name}, starting from epoch 1.") return 0, None
Integrating into your Training Loop:
Integrate these functions into your training loop. The loop should check for existing checkpoints before starting training. If a checkpoint is found, it resumes training from the saved epoch.
EPOCHS = 10 for exp in experiments: # Assuming 'experiments' is a list of your experiment configurations model, optimizer, scheduler = initialise_model_components(exp) # Your model initialization function train_loader, val_loader = generate_data_loaders(exp) # Your data loader function start_epoch, prev_loss = load_checkpoint(exp, model, optimizer, scheduler) for epoch in range(start_epoch, EPOCHS): print(f'Epoch {epoch + 1}/{EPOCHS}') # YOUR TRAINING CODE HERE... (training loop) save_checkpoint(epoch + 1, model, optimizer, scheduler, train_loss, exp) #Save after each epoch
This structure allows for seamless resumption of training even if the Colab session terminates. Remember to adapt the get_checkpoint_dir
function and checkpoint file naming conventions to match your specific needs. This improved example handles potential errors more gracefully and provides a more robust solution. Remember to replace placeholder functions (initialise_model_components
, generate_data_loaders
) with your actual implementations.
The above is the detailed content of Training Language Models on Google Colab. For more information, please follow other related articles on the PHP Chinese website!

Hot AI Tools

Undresser.AI Undress
AI-powered app for creating realistic nude photos

AI Clothes Remover
Online AI tool for removing clothes from photos.

Undress AI Tool
Undress images for free

Clothoff.io
AI clothes remover

Video Face Swap
Swap faces in any video effortlessly with our completely free AI face swap tool!

Hot Article

Hot Tools

Notepad++7.3.1
Easy-to-use and free code editor

SublimeText3 Chinese version
Chinese version, very easy to use

Zend Studio 13.0.1
Powerful PHP integrated development environment

Dreamweaver CS6
Visual web development tools

SublimeText3 Mac version
God-level code editing software (SublimeText3)

Hot Topics










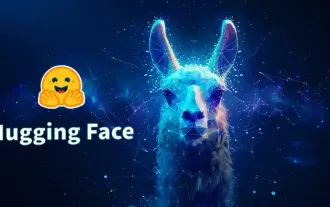
Meta's Llama 3.2: A Leap Forward in Multimodal and Mobile AI Meta recently unveiled Llama 3.2, a significant advancement in AI featuring powerful vision capabilities and lightweight text models optimized for mobile devices. Building on the success o
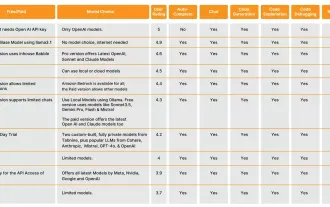
Hey there, Coding ninja! What coding-related tasks do you have planned for the day? Before you dive further into this blog, I want you to think about all your coding-related woes—better list those down. Done? – Let’
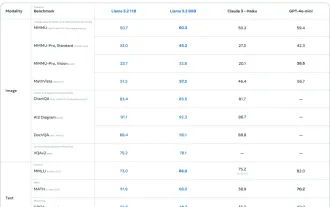
This week's AI landscape: A whirlwind of advancements, ethical considerations, and regulatory debates. Major players like OpenAI, Google, Meta, and Microsoft have unleashed a torrent of updates, from groundbreaking new models to crucial shifts in le
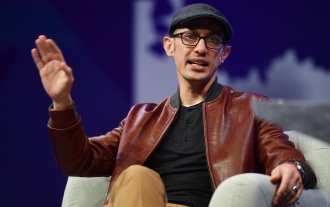
Shopify CEO Tobi Lütke's recent memo boldly declares AI proficiency a fundamental expectation for every employee, marking a significant cultural shift within the company. This isn't a fleeting trend; it's a new operational paradigm integrated into p
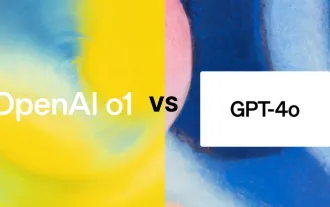
Introduction OpenAI has released its new model based on the much-anticipated “strawberry” architecture. This innovative model, known as o1, enhances reasoning capabilities, allowing it to think through problems mor
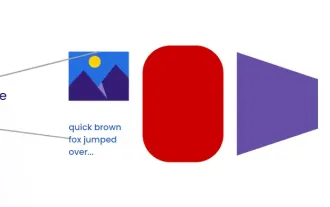
Introduction Imagine walking through an art gallery, surrounded by vivid paintings and sculptures. Now, what if you could ask each piece a question and get a meaningful answer? You might ask, “What story are you telling?
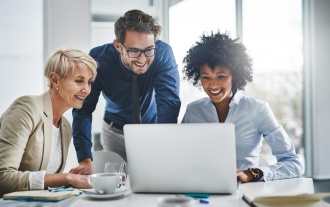
For those of you who might be new to my column, I broadly explore the latest advances in AI across the board, including topics such as embodied AI, AI reasoning, high-tech breakthroughs in AI, prompt engineering, training of AI, fielding of AI, AI re
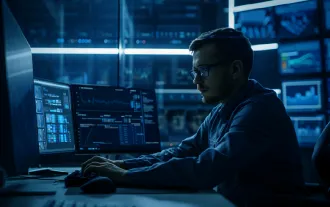
SQL's ALTER TABLE Statement: Dynamically Adding Columns to Your Database In data management, SQL's adaptability is crucial. Need to adjust your database structure on the fly? The ALTER TABLE statement is your solution. This guide details adding colu
