Making News Recommendations Explainable with Large Language Models
DER SPIEGEL explores using Large Language Models (LLMs) to improve news article recommendations. An offline experiment assessed an LLM's ability to predict reader interest based on reading history.
Methodology:
Reader survey data provided a ground truth of preferences. Each participant's reading history and article interest ratings were used. Anthropic's Claude 3.5 Sonnet LLM, acting as a recommendation engine, received each reader's history (title and summary) to predict interest in new articles (scored 0-1000). A JSON output format ensured structured results. The LLM's predictions were compared to actual survey ratings. A detailed methodology is available in:
A Mixed-Methods Approach to Offline Evaluation of News Recommender Systems
Key Findings:
Impressive results were achieved. Precision@5 reached 56% – when recommending 5 articles, nearly 3 were among a user's top-rated articles. For 24% of users, 4 or 5 top articles were correctly predicted; for another 41%, 3 out of 5 were correct. This significantly outperforms random recommendations (38.8%), popularity-based recommendations (42.1%), and a previous embedding-based approach (45.4%).
The chart illustrates the performance uplift of the LLM approach over other methods.
Spearman correlation, a second metric, reached 0.41, substantially exceeding the embedding-based approach (0.17), indicating superior understanding of preference strength.
Explainability:
The LLM's explainability is a key advantage. An example shows how the system analyzes reading patterns and justifies recommendations:
<code>User has 221 articles in reading history Top 5 Predicted by Claude: ... (List of articles with scores and actual ratings) Claude's Analysis: ... (Analysis of reading patterns and scoring rationale)</code>
This transparency enhances trust and personalization.
Challenges and Future Directions:
High API costs ($0.21 per user) and processing speed (several seconds per user) pose scalability challenges. Exploring open-source models and prompt engineering could mitigate these. Incorporating additional data (reading time, article popularity) could further improve performance.
Conclusion:
The strong predictive power and explainability of LLMs make them valuable for news recommendation. Beyond recommendations, they offer new ways to analyze user behavior and content journeys, enabling personalized summaries and insights.
Acknowledgments
This research utilized anonymized, aggregated user data. Further discussion is welcome via LinkedIn.
References
[1] Dairui, Liu & Yang, Boming & Du, Honghui & Greene, Derek & Hurley, Neil & Lawlor, Aonghus & Dong, Ruihai & Li, Irene. (2024). RecPrompt: A Self-tuning Prompting Framework for News Recommendation Using Large Language Models.
The above is the detailed content of Making News Recommendations Explainable with Large Language Models. For more information, please follow other related articles on the PHP Chinese website!

Hot AI Tools

Undresser.AI Undress
AI-powered app for creating realistic nude photos

AI Clothes Remover
Online AI tool for removing clothes from photos.

Undress AI Tool
Undress images for free

Clothoff.io
AI clothes remover

Video Face Swap
Swap faces in any video effortlessly with our completely free AI face swap tool!

Hot Article

Hot Tools

Notepad++7.3.1
Easy-to-use and free code editor

SublimeText3 Chinese version
Chinese version, very easy to use

Zend Studio 13.0.1
Powerful PHP integrated development environment

Dreamweaver CS6
Visual web development tools

SublimeText3 Mac version
God-level code editing software (SublimeText3)

Hot Topics










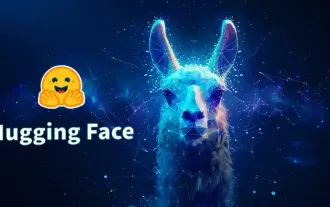
Meta's Llama 3.2: A Leap Forward in Multimodal and Mobile AI Meta recently unveiled Llama 3.2, a significant advancement in AI featuring powerful vision capabilities and lightweight text models optimized for mobile devices. Building on the success o
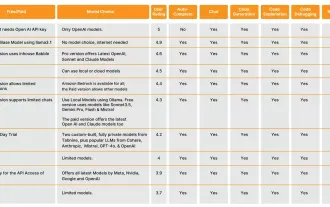
Hey there, Coding ninja! What coding-related tasks do you have planned for the day? Before you dive further into this blog, I want you to think about all your coding-related woes—better list those down. Done? – Let’
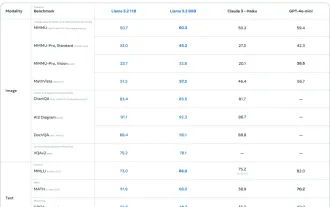
This week's AI landscape: A whirlwind of advancements, ethical considerations, and regulatory debates. Major players like OpenAI, Google, Meta, and Microsoft have unleashed a torrent of updates, from groundbreaking new models to crucial shifts in le
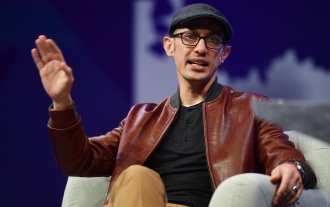
Shopify CEO Tobi Lütke's recent memo boldly declares AI proficiency a fundamental expectation for every employee, marking a significant cultural shift within the company. This isn't a fleeting trend; it's a new operational paradigm integrated into p
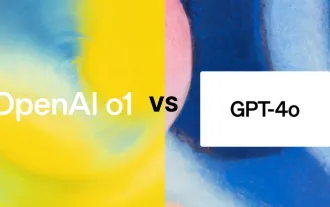
Introduction OpenAI has released its new model based on the much-anticipated “strawberry” architecture. This innovative model, known as o1, enhances reasoning capabilities, allowing it to think through problems mor
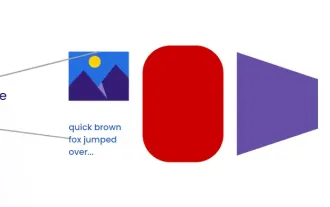
Introduction Imagine walking through an art gallery, surrounded by vivid paintings and sculptures. Now, what if you could ask each piece a question and get a meaningful answer? You might ask, “What story are you telling?
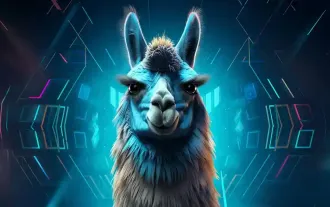
Meta's Llama 3.2: A Multimodal AI Powerhouse Meta's latest multimodal model, Llama 3.2, represents a significant advancement in AI, boasting enhanced language comprehension, improved accuracy, and superior text generation capabilities. Its ability t
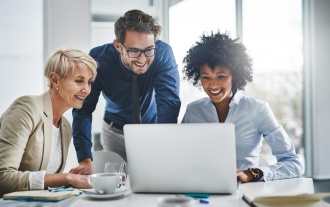
For those of you who might be new to my column, I broadly explore the latest advances in AI across the board, including topics such as embodied AI, AI reasoning, high-tech breakthroughs in AI, prompt engineering, training of AI, fielding of AI, AI re
