Training Large Language Models: From TRPO to GRPO
DeepSeek: A Deep Dive into Reinforcement Learning for LLMs
DeepSeek's recent success, achieving impressive performance at lower costs, highlights the importance of Large Language Model (LLM) training methods. This article focuses on the Reinforcement Learning (RL) aspect, exploring TRPO, PPO, and the newer GRPO algorithms. We'll minimize complex math to make it accessible, assuming basic familiarity with machine learning, deep learning, and LLMs.
Three Pillars of LLM Training
LLM training typically involves three key phases:
- Pre-training: The model learns to predict the next token in a sequence from preceding tokens using a massive dataset.
- Supervised Fine-Tuning (SFT): Targeted data refines the model, aligning it with specific instructions.
- Reinforcement Learning (RLHF): This stage, the focus of this article, further refines responses to better match human preferences through direct feedback.
Reinforcement Learning Fundamentals
Reinforcement learning involves an agent interacting with an environment. The agent exists in a specific state, taking actions to transition to new states. Each action results in a reward from the environment, guiding the agent's future actions. Think of a robot navigating a maze: its position is the state, movements are actions, and reaching the exit provides a positive reward.
RL in LLMs: A Detailed Look
In LLM training, the components are:
- Agent: The LLM itself.
- Environment: External factors like user prompts, feedback systems, and contextual information.
- Actions: The tokens the LLM generates in response to a query.
- State: The current query and the generated tokens (partial response).
- Rewards: Usually determined by a separate reward model trained on human-annotated data, ranking responses to assign scores. Higher-quality responses receive higher rewards. Simpler, rule-based rewards are possible in specific cases, such as DeepSeekMath.
The policy determines which action to take. For an LLM, it's a probability distribution over possible tokens, used to sample the next token. RL training adjusts the policy's parameters (model weights) to favor higher-reward tokens. The policy is often represented as:
The core of RL is finding the optimal policy. Unlike supervised learning, we use rewards to guide policy adjustments.
TRPO (Trust Region Policy Optimization)
TRPO uses an advantage function, analogous to the loss function in supervised learning, but derived from rewards:
TRPO maximizes a surrogate objective, constrained to prevent large policy deviations from the previous iteration, ensuring stability:
PPO (Proximal Policy Optimization)
PPO, now preferred for LLMs like ChatGPT and Gemini, simplifies TRPO by using a clipped surrogate objective, implicitly limiting policy updates and improving computational efficiency. The PPO objective function is:
GRPO (Group Relative Policy Optimization)
GRPO streamlines training by eliminating the separate value model. For each query, it generates a group of responses and calculates the advantage as a z-score based on their rewards:
This simplifies the process and is well-suited for LLMs' ability to generate multiple responses. GRPO also incorporates a KL divergence term, comparing the current policy to a reference policy. The final GRPO formulation is:
Conclusion
Reinforcement learning, particularly PPO and the newer GRPO, is crucial for modern LLM training. Each method builds upon RL fundamentals, offering different approaches to balance stability, efficiency, and human alignment. DeepSeek's success leverages these advancements, along with other innovations. Reinforcement learning is poised to play an increasingly dominant role in advancing LLM capabilities.
References: (The references remain the same, just reformatted for better readability)
- [1] "Foundations of Large Language Models", 2025. https://www.php.cn/link/fbf8ca43dcc014c2c94549d6b8ca0375
- [2] "Reinforcement Learning." Enaris. Available at: https://www.php.cn/link/20e169b48c8f869887e2bbe1c5c3ea65
- [3] Y. Gokhale. "Introduction to LLMs and the Generative AI Part 5: RLHF," Medium, 2023. Available at: https://www.php.cn/link/b24b1810f41d38b55728a9f56b043d35
- [4] L. Weng. "An Overview of Reinforcement Learning," 2018. Available at: https://www.php.cn/link/fc42bad715bcb9767ddd95a239552434
- [5] "DeepSeek-R1: Incentivizing Reasoning Capability in LLMs via Reinforcement Learning", 2025. https://www.php.cn/link/d0ae1e3078807c85d78d64f4ded5cdcb
- [6] "DeepSeekMath: Pushing the Limits of Mathematical Reasoning in Open Language Models", 2025. https://www.php.cn/link/f8b18593cdbb1ce289330560a44e33aa
- [7] "Trust Region Policy Optimization", 2017. https://www.php.cn/link/77a44d5cfb595b3545d61aa742268c9b
The above is the detailed content of Training Large Language Models: From TRPO to GRPO. For more information, please follow other related articles on the PHP Chinese website!

Hot AI Tools

Undresser.AI Undress
AI-powered app for creating realistic nude photos

AI Clothes Remover
Online AI tool for removing clothes from photos.

Undress AI Tool
Undress images for free

Clothoff.io
AI clothes remover

Video Face Swap
Swap faces in any video effortlessly with our completely free AI face swap tool!

Hot Article

Hot Tools

Notepad++7.3.1
Easy-to-use and free code editor

SublimeText3 Chinese version
Chinese version, very easy to use

Zend Studio 13.0.1
Powerful PHP integrated development environment

Dreamweaver CS6
Visual web development tools

SublimeText3 Mac version
God-level code editing software (SublimeText3)

Hot Topics










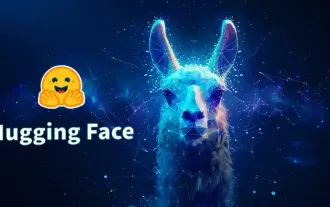
Meta's Llama 3.2: A Leap Forward in Multimodal and Mobile AI Meta recently unveiled Llama 3.2, a significant advancement in AI featuring powerful vision capabilities and lightweight text models optimized for mobile devices. Building on the success o
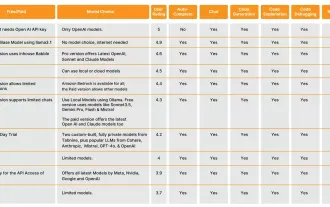
Hey there, Coding ninja! What coding-related tasks do you have planned for the day? Before you dive further into this blog, I want you to think about all your coding-related woes—better list those down. Done? – Let’
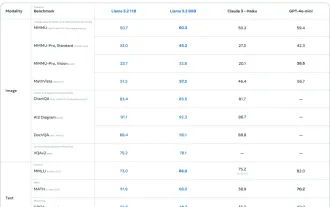
This week's AI landscape: A whirlwind of advancements, ethical considerations, and regulatory debates. Major players like OpenAI, Google, Meta, and Microsoft have unleashed a torrent of updates, from groundbreaking new models to crucial shifts in le
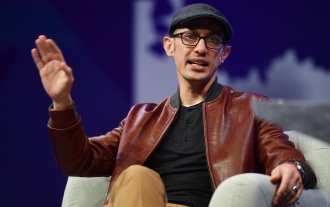
Shopify CEO Tobi Lütke's recent memo boldly declares AI proficiency a fundamental expectation for every employee, marking a significant cultural shift within the company. This isn't a fleeting trend; it's a new operational paradigm integrated into p
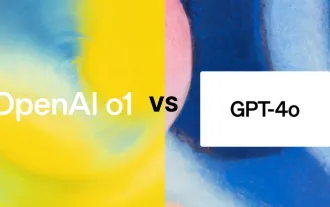
Introduction OpenAI has released its new model based on the much-anticipated “strawberry” architecture. This innovative model, known as o1, enhances reasoning capabilities, allowing it to think through problems mor
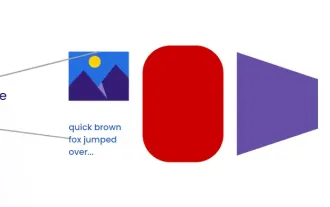
Introduction Imagine walking through an art gallery, surrounded by vivid paintings and sculptures. Now, what if you could ask each piece a question and get a meaningful answer? You might ask, “What story are you telling?
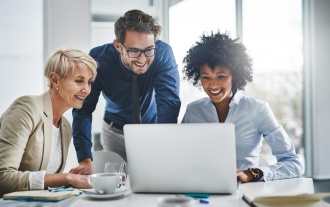
For those of you who might be new to my column, I broadly explore the latest advances in AI across the board, including topics such as embodied AI, AI reasoning, high-tech breakthroughs in AI, prompt engineering, training of AI, fielding of AI, AI re
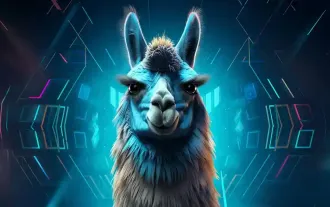
Meta's Llama 3.2: A Multimodal AI Powerhouse Meta's latest multimodal model, Llama 3.2, represents a significant advancement in AI, boasting enhanced language comprehension, improved accuracy, and superior text generation capabilities. Its ability t
