


How to efficiently add continuous sequence numbers to data columns in Python so that the same value has the same sequence number?
How to efficiently add consecutive numbers to a data column in Python, assigning the same number to identical values?
This task involves creating a sequential counter that resets whenever the value in a specific column changes. The most efficient way to achieve this in Python leverages the power of the pandas
library. Pandas provides vectorized operations that are significantly faster than iterating through rows.
Here's how you can do it:
import pandas as pd # Sample data data = {'col1': ['A', 'A', 'B', 'B', 'B', 'C', 'A', 'A', 'D']} df = pd.DataFrame(data) # Efficiently assign sequential numbers df['col2'] = (df['col1'] != df['col1'].shift()).cumsum() print(df)
This code first uses df['col1'].shift()
to create a lagged version of the 'col1' column. Comparing this lagged version with the original column (df['col1'] != df['col1'].shift()
) identifies where the values change. The .cumsum()
method then cumulatively sums the boolean results, effectively creating a sequential counter that increments only when a new value is encountered. This assigns a unique consecutive number to each group of identical values in 'col1', storing the result in a new column named 'col2'.
What's the most efficient Python method for creating a sequential ID based on duplicate values in a column?
The most efficient method builds upon the previous approach, refining it to generate more descriptive sequential IDs. Instead of simply assigning consecutive numbers, we can create IDs that explicitly reflect the grouping. This is achieved by combining the group identifier with a sequential counter within each group.
import pandas as pd data = {'col1': ['A', 'A', 'B', 'B', 'B', 'C', 'A', 'A', 'D']} df = pd.DataFrame(data) df['group_id'] = (df['col1'] != df['col1'].shift()).cumsum() df['sequential_id'] = df.groupby('group_id').cumcount() + 1 df['final_id'] = df['col1'] + '_' + df['sequential_id'].astype(str) print(df)
This enhanced code first identifies groups using the same method as before. Then, df.groupby('group_id').cumcount()
generates a sequential counter within each group. We add 1 to start the count from 1 instead of 0. Finally, we concatenate the original value from 'col1' with the sequential ID to create a more informative unique identifier in 'final_id'. This method efficiently handles large datasets due to pandas' vectorized operations.
Can Python handle adding sequential numbering to a column while grouping by identical values?
Yes, Python, specifically with the pandas library, excels at this task. The previous examples demonstrate this capability. The groupby()
method, combined with .cumcount()
, provides a powerful and efficient way to add sequential numbering within groups defined by identical values in a column. The efficiency stems from pandas' ability to perform these operations on the entire DataFrame at once, avoiding slow row-by-row iteration.
How can I optimize Python code to generate unique sequential IDs for groups of identical values within a column?
Optimizing the code for generating unique sequential IDs primarily focuses on leveraging pandas' vectorized operations and avoiding explicit loops. The previous examples already showcase this optimization. To further enhance performance for extremely large datasets:
-
Avoid unnecessary data copies: Be mindful of operations that create unnecessary copies of the DataFrame. Pandas' in-place operations (using
inplace=True
) can sometimes improve performance. However, often the performance gains are negligible compared to the readability cost. - Chunking (for extremely large datasets): If your dataset is so large that it exceeds available memory, consider processing it in chunks. Read and process the data in smaller, manageable pieces, then concatenate the results.
- Consider alternative data structures (rarely necessary): While pandas is generally efficient, for exceptionally specific scenarios with billions of rows, specialized libraries or databases optimized for this type of operation might offer a marginal performance increase. However, the complexity of integrating these alternatives often outweighs the benefit for most practical applications.
The solutions provided above using pandas are generally highly optimized for most real-world scenarios involving sequential ID generation based on grouping. Focusing on efficient pandas techniques is the most effective approach for optimization.
The above is the detailed content of How to efficiently add continuous sequence numbers to data columns in Python so that the same value has the same sequence number?. For more information, please follow other related articles on the PHP Chinese website!

Hot AI Tools

Undresser.AI Undress
AI-powered app for creating realistic nude photos

AI Clothes Remover
Online AI tool for removing clothes from photos.

Undress AI Tool
Undress images for free

Clothoff.io
AI clothes remover

Video Face Swap
Swap faces in any video effortlessly with our completely free AI face swap tool!

Hot Article

Hot Tools

Notepad++7.3.1
Easy-to-use and free code editor

SublimeText3 Chinese version
Chinese version, very easy to use

Zend Studio 13.0.1
Powerful PHP integrated development environment

Dreamweaver CS6
Visual web development tools

SublimeText3 Mac version
God-level code editing software (SublimeText3)

Hot Topics
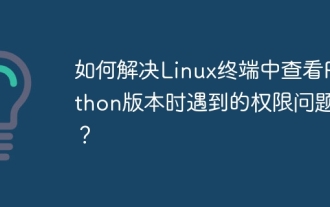
Solution to permission issues when viewing Python version in Linux terminal When you try to view Python version in Linux terminal, enter python...
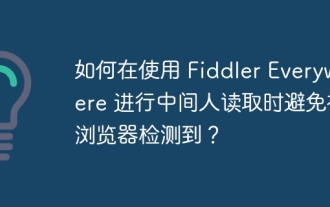
How to avoid being detected when using FiddlerEverywhere for man-in-the-middle readings When you use FiddlerEverywhere...
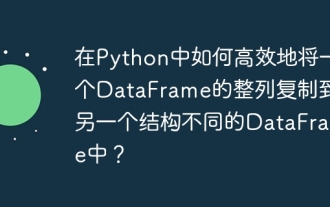
When using Python's pandas library, how to copy whole columns between two DataFrames with different structures is a common problem. Suppose we have two Dats...
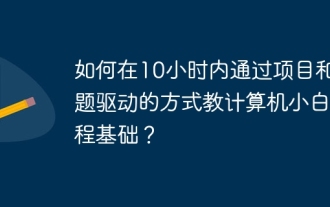
How to teach computer novice programming basics within 10 hours? If you only have 10 hours to teach computer novice some programming knowledge, what would you choose to teach...
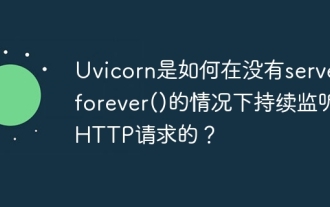
How does Uvicorn continuously listen for HTTP requests? Uvicorn is a lightweight web server based on ASGI. One of its core functions is to listen for HTTP requests and proceed...
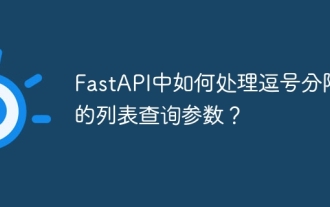
Fastapi ...
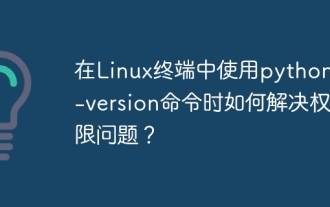
Using python in Linux terminal...
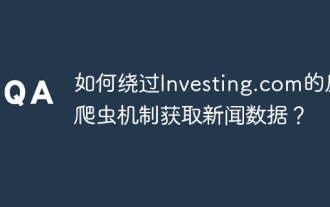
Understanding the anti-crawling strategy of Investing.com Many people often try to crawl news data from Investing.com (https://cn.investing.com/news/latest-news)...
