8 Types of Chunking for RAG Systems - Analytics Vidhya
Unlocking the Power of Chunking in Retrieval-Augmented Generation (RAG): A Deep Dive
Efficiently processing large volumes of text data is crucial for building robust and effective Retrieval-Augmented Generation (RAG) systems. This article explores various chunking strategies, vital for optimizing data handling and improving the performance of AI-powered applications. We'll delve into different approaches, highlighting their strengths and weaknesses, and offering practical examples.
Table of Contents
- What is Chunking in RAG?
- The Importance of Chunking
- Understanding RAG Architecture and Chunking
- Common Challenges with RAG Systems
- Selecting the Optimal Chunking Strategy
- Character-Based Text Chunking
- Recursive Character Text Splitting with LangChain
- Document-Specific Chunking (HTML, Python, JSON, etc.)
- Semantic Chunking with LangChain and OpenAI
- Agentic Chunking (LLM-Driven Chunking)
- Section-Based Chunking
- Contextual Chunking for Enhanced Retrieval
- Late Chunking for Preserving Long-Range Context
- Conclusion
What is Chunking in RAG?
Chunking is the process of dividing large text documents into smaller, more manageable units. This is essential for RAG systems because language models have limited context windows. Chunking ensures that relevant information remains within these limits, maximizing the signal-to-noise ratio and improving model performance. The goal is not just to split the data, but to optimize its presentation to the model for enhanced retrievability and accuracy.
Why is Chunking Important?
Anton Troynikov, co-founder of Chroma, emphasizes that irrelevant data within the context window significantly reduces application effectiveness. Chunking is vital for:
- Overcoming Context Window Limits: Ensures key information isn't lost due to size restrictions.
- Improving Signal-to-Noise Ratio: Filters out irrelevant content, enhancing model accuracy.
- Boosting Retrieval Efficiency: Facilitates faster and more precise retrieval of relevant information.
- Task-Specific Optimization: Allows tailoring chunking strategies to specific application needs (e.g., summarization vs. question-answering).
RAG Architecture and Chunking
The RAG architecture involves three key stages:
- Chunking: Raw data is split into smaller, meaningful chunks.
- Embedding: Chunks are converted into vector embeddings.
- Retrieval & Generation: Relevant chunks are retrieved based on user queries, and the LLM generates a response using the retrieved information.
Challenges in RAG Systems
RAG systems face several challenges:
- Retrieval Issues: Inaccurate or incomplete retrieval of relevant information.
- Generation Difficulties: Hallucinations, irrelevant or biased outputs.
- Integration Problems: Difficulty combining retrieved information coherently.
Choosing the Right Chunking Strategy
The ideal chunking strategy depends on several factors: content type, embedding model, and anticipated user queries. Consider the structure and density of the content, the token limitations of the embedding model, and the types of questions users are likely to ask.
1. Character-Based Text Chunking
This simple method splits text into fixed-size chunks based on character count, regardless of semantic meaning. While straightforward, it often disrupts sentence structure and context. Example using Python:
text = "Clouds come floating into my life..." chunks = [] chunk_size = 35 chunk_overlap = 5 # ... (Chunking logic as in the original example)
2. Recursive Character Text Splitting with LangChain
This approach recursively splits text using multiple separators (e.g., double newlines, single newlines, spaces) and merges smaller chunks to optimize for a target character size. It's more sophisticated than character-based chunking, offering better context preservation. Example using LangChain:
# ... (LangChain installation and code as in the original example)
3. Document-Specific Chunking
This method adapts chunking to different document formats (HTML, Python, Markdown, etc.) using format-specific separators. This ensures that the chunking respects the inherent structure of the document. Examples using LangChain for Python and Markdown are provided in the original response.
4. Semantic Chunking with LangChain and OpenAI
Semantic chunking divides text based on semantic meaning, using techniques like sentence embeddings to identify natural breakpoints. This approach ensures that each chunk represents a coherent idea. Example using LangChain and OpenAI embeddings:
# ... (OpenAI API key setup and code as in the original example)
5. Agentic Chunking (LLM-Driven Chunking)
Agentic chunking utilizes an LLM to identify natural breakpoints in the text, resulting in more contextually relevant chunks. This approach leverages the LLM's understanding of language and context to produce more meaningful segments. Example using OpenAI API:
text = "Clouds come floating into my life..." chunks = [] chunk_size = 35 chunk_overlap = 5 # ... (Chunking logic as in the original example)
6. Section-Based Chunking
This method leverages the document's inherent structure (headings, subheadings, sections) to define chunks. It's particularly effective for well-structured documents like research papers or reports. Example using PyMuPDF and Latent Dirichlet Allocation (LDA) for topic-based chunking:
# ... (LangChain installation and code as in the original example)
7. Contextual Chunking
Contextual chunking focuses on preserving semantic context within each chunk. This ensures that the retrieved information is coherent and relevant. Example using LangChain and a custom prompt:
# ... (OpenAI API key setup and code as in the original example)
8. Late Chunking
Late chunking delays chunking until after generating embeddings for the entire document. This preserves long-range contextual dependencies, improving the accuracy of embeddings and retrieval. Example using the Jina embeddings model:
# ... (OpenAI API key setup and code as in the original example)
Conclusion
Effective chunking is paramount for building high-performing RAG systems. The choice of chunking strategy significantly impacts the quality of information retrieval and the coherence of the generated responses. By carefully considering the characteristics of the data and the specific requirements of the application, developers can select the most appropriate chunking method to optimize their RAG system's performance. Remember to always prioritize maintaining contextual integrity and relevance within each chunk.
The above is the detailed content of 8 Types of Chunking for RAG Systems - Analytics Vidhya. For more information, please follow other related articles on the PHP Chinese website!

Hot AI Tools

Undresser.AI Undress
AI-powered app for creating realistic nude photos

AI Clothes Remover
Online AI tool for removing clothes from photos.

Undress AI Tool
Undress images for free

Clothoff.io
AI clothes remover

Video Face Swap
Swap faces in any video effortlessly with our completely free AI face swap tool!

Hot Article

Hot Tools

Notepad++7.3.1
Easy-to-use and free code editor

SublimeText3 Chinese version
Chinese version, very easy to use

Zend Studio 13.0.1
Powerful PHP integrated development environment

Dreamweaver CS6
Visual web development tools

SublimeText3 Mac version
God-level code editing software (SublimeText3)

Hot Topics
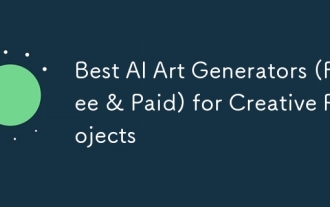
The article reviews top AI art generators, discussing their features, suitability for creative projects, and value. It highlights Midjourney as the best value for professionals and recommends DALL-E 2 for high-quality, customizable art.
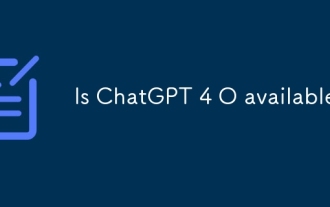
ChatGPT 4 is currently available and widely used, demonstrating significant improvements in understanding context and generating coherent responses compared to its predecessors like ChatGPT 3.5. Future developments may include more personalized interactions and real-time data processing capabilities, further enhancing its potential for various applications.
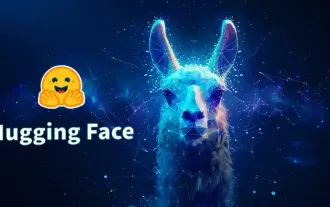
Meta's Llama 3.2: A Leap Forward in Multimodal and Mobile AI Meta recently unveiled Llama 3.2, a significant advancement in AI featuring powerful vision capabilities and lightweight text models optimized for mobile devices. Building on the success o
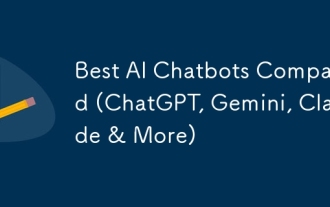
The article compares top AI chatbots like ChatGPT, Gemini, and Claude, focusing on their unique features, customization options, and performance in natural language processing and reliability.
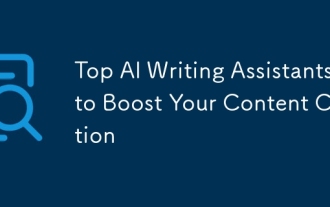
The article discusses top AI writing assistants like Grammarly, Jasper, Copy.ai, Writesonic, and Rytr, focusing on their unique features for content creation. It argues that Jasper excels in SEO optimization, while AI tools help maintain tone consist
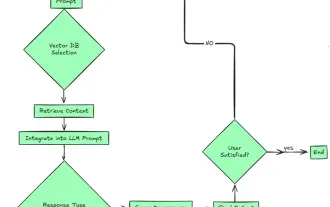
2024 witnessed a shift from simply using LLMs for content generation to understanding their inner workings. This exploration led to the discovery of AI Agents – autonomous systems handling tasks and decisions with minimal human intervention. Buildin
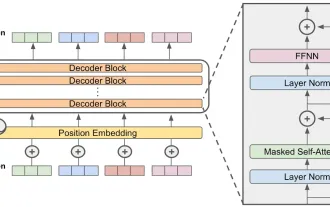
Falcon 3: A Revolutionary Open-Source Large Language Model Falcon 3, the latest iteration in the acclaimed Falcon series of LLMs, represents a significant advancement in AI technology. Developed by the Technology Innovation Institute (TII), this open
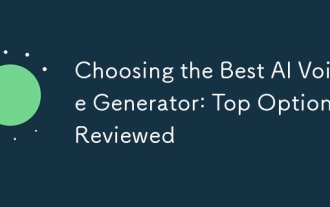
The article reviews top AI voice generators like Google Cloud, Amazon Polly, Microsoft Azure, IBM Watson, and Descript, focusing on their features, voice quality, and suitability for different needs.
