Guide on YOLOv11 Model Building from Scratch using PyTorch
YOLOv11: A Deep Dive into the Architecture and Implementation of a Cutting-Edge Object Detection Model
YOLO (You Only Look Once) models are renowned for their efficiency and accuracy in computer vision tasks, including object detection, segmentation, pose estimation, and more. This article focuses on the architecture and implementation of the latest iteration, YOLOv11, using PyTorch. While Ultralytics, the creators, prioritize practical application over formal research papers, we'll dissect its design and build a functional model.
Understanding YOLOv11's Architecture
YOLOv11, like its predecessors, employs a three-part architecture: backbone, neck, and head.
-
Backbone: Extracts features using efficient bottleneck-based blocks (C3K2, a refinement of YOLOv8's C2F). This backbone, leveraging DarkNet and DarkFPN, produces three feature maps (P3, P4, P5) representing different levels of detail.
-
Neck: Processes the backbone's output, fusing features across scales using upsampling and concatenation. A crucial component is the C2PSA block, incorporating Partial Spatial Attention (PSA) modules to enhance focus on relevant spatial information in low-level features.
-
Head: Handles task-specific predictions. For object detection, it includes:
- DFL (Distribution Focal Loss): Refines bounding box predictions.
- Box Detection: Predicts bounding box coordinates.
- Class Detection: Predicts object classes. The model also supports segmentation and keypoint detection heads.
Core Building Blocks: Convolution and Bottleneck Layers
The model relies heavily on:
- Convolutional Layers: Extract features from input data.
- Bottleneck Layers: Improve efficiency and learning by using two convolutional layers with a shortcut connection (concatenation or residual addition).
- C3K and C3K2 Modules: Optimized convolutional blocks for efficient feature extraction.
- SPPF (Spatial Pyramid Pooling Fast): Enhances the model's ability to detect objects of varying sizes.
Code Implementation Highlights (PyTorch)
The following code snippets illustrate key components:
(Simplified for brevity; refer to the original article for complete code.)
# Simplified Conv Block class Conv(nn.Module): def __init__(self, in_ch, out_ch, activation, ...): # ... (Initialization code) ... def forward(self, x): return activation(self.norm(self.conv(x))) # Simplified Bottleneck Block (Residual) class Residual(nn.Module): def __init__(self, ch, e=0.5): # ... (Initialization code) ... def forward(self, x): return x + self.conv2(self.conv1(x)) # Simplified SPPF class SPPF(nn.Module): def __init__(self, c1, c2, k=5): # ... (Initialization code) ... def forward(self, x): # ... (MaxPooling and concatenation) ... return self.cv2(torch.cat((x, y1, y2, self.m(y2)), 1)) # ... (Other key blocks: C3K, C3K2, PSA, Attention, PSABlock, DFL) ...
Model Construction and Testing
The complete YOLOv11 model is constructed by combining the backbone, neck, and head. Different model sizes (nano, small, medium, large, xlarge) are achieved by adjusting parameters like depth and width. The provided code includes a YOLOv11
class to facilitate this.
Model testing with a random input tensor demonstrates the output structure (feature maps in training mode, concatenated predictions in evaluation mode). Further processing (Non-Maximum Suppression) is necessary to obtain final object detections.
Conclusion
YOLOv11 represents a significant advancement in object detection, offering a powerful and efficient architecture. Its design prioritizes practical applications, making it a valuable tool for real-world AI projects. The detailed architecture and code snippets provide a solid foundation for understanding and further development. Remember to consult the original article for the complete, runnable code.
The above is the detailed content of Guide on YOLOv11 Model Building from Scratch using PyTorch. For more information, please follow other related articles on the PHP Chinese website!

Hot AI Tools

Undresser.AI Undress
AI-powered app for creating realistic nude photos

AI Clothes Remover
Online AI tool for removing clothes from photos.

Undress AI Tool
Undress images for free

Clothoff.io
AI clothes remover

Video Face Swap
Swap faces in any video effortlessly with our completely free AI face swap tool!

Hot Article

Hot Tools

Notepad++7.3.1
Easy-to-use and free code editor

SublimeText3 Chinese version
Chinese version, very easy to use

Zend Studio 13.0.1
Powerful PHP integrated development environment

Dreamweaver CS6
Visual web development tools

SublimeText3 Mac version
God-level code editing software (SublimeText3)

Hot Topics
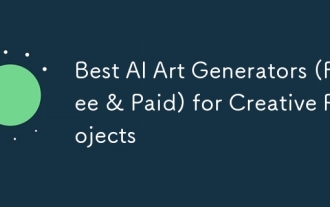
The article reviews top AI art generators, discussing their features, suitability for creative projects, and value. It highlights Midjourney as the best value for professionals and recommends DALL-E 2 for high-quality, customizable art.
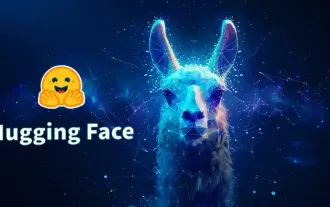
Meta's Llama 3.2: A Leap Forward in Multimodal and Mobile AI Meta recently unveiled Llama 3.2, a significant advancement in AI featuring powerful vision capabilities and lightweight text models optimized for mobile devices. Building on the success o
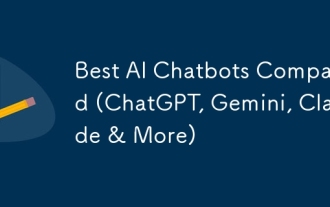
The article compares top AI chatbots like ChatGPT, Gemini, and Claude, focusing on their unique features, customization options, and performance in natural language processing and reliability.
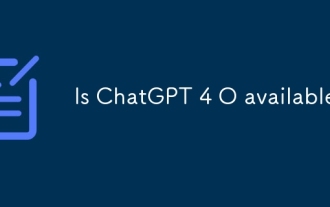
ChatGPT 4 is currently available and widely used, demonstrating significant improvements in understanding context and generating coherent responses compared to its predecessors like ChatGPT 3.5. Future developments may include more personalized interactions and real-time data processing capabilities, further enhancing its potential for various applications.
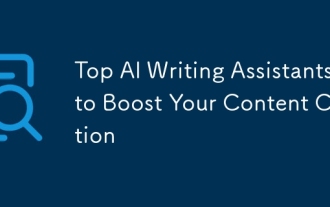
The article discusses top AI writing assistants like Grammarly, Jasper, Copy.ai, Writesonic, and Rytr, focusing on their unique features for content creation. It argues that Jasper excels in SEO optimization, while AI tools help maintain tone consist
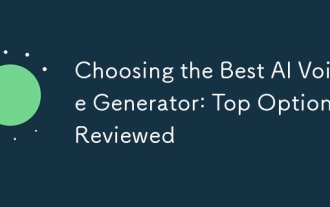
The article reviews top AI voice generators like Google Cloud, Amazon Polly, Microsoft Azure, IBM Watson, and Descript, focusing on their features, voice quality, and suitability for different needs.
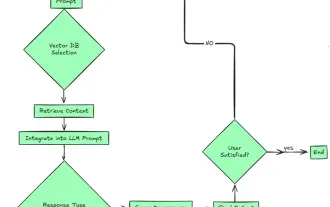
2024 witnessed a shift from simply using LLMs for content generation to understanding their inner workings. This exploration led to the discovery of AI Agents – autonomous systems handling tasks and decisions with minimal human intervention. Buildin
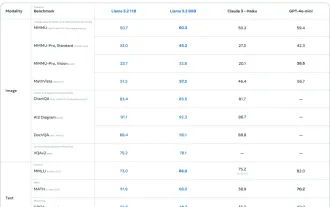
This week's AI landscape: A whirlwind of advancements, ethical considerations, and regulatory debates. Major players like OpenAI, Google, Meta, and Microsoft have unleashed a torrent of updates, from groundbreaking new models to crucial shifts in le
