LightRAG: Simple and Fast Alternative to GraphRAG
LightRAG: A Lightweight Retrieval-Augmented Generation System
Large Language Models (LLMs) are rapidly evolving, but effectively integrating external knowledge remains a significant hurdle. Retrieval-Augmented Generation (RAG) techniques aim to improve LLM output by incorporating relevant information during generation. However, traditional RAG systems can be complex and resource-intensive. The HKU Data Science Lab addresses this with LightRAG, a more efficient alternative. LightRAG combines the power of knowledge graphs with vector retrieval, enabling efficient processing of textual information while maintaining the structured relationships within the data.
Key Learning Points:
- Limitations of traditional RAG and the need for LightRAG.
- LightRAG's architecture: dual-level retrieval and graph-based text indexing.
- Integration of graph structures and vector embeddings for efficient, context-rich retrieval.
- LightRAG's performance compared to GraphRAG across various domains.
Why LightRAG Outperforms Traditional RAG:
Traditional RAG systems often struggle with complex relationships between data points, resulting in fragmented responses. They use simple, flat data representations, lacking contextual understanding. For example, a query about the impact of electric vehicles on air quality and public transport might yield separate results on each topic, failing to connect them meaningfully. LightRAG addresses this limitation.
How LightRAG Functions:
LightRAG uses graph-based indexing and a dual-level retrieval mechanism for efficient and context-rich responses to complex queries.
Graph-Based Text Indexing:
This process involves:
- Chunking: Dividing documents into smaller segments.
- Entity Recognition: Using LLMs to identify and extract entities (names, dates, etc.) and their relationships.
- Knowledge Graph Construction: Building a knowledge graph representing the connections between entities. Redundancies are removed for optimization.
- Embedding Storage: Storing descriptions and relationships as vectors in a vector database.
Dual-Level Retrieval:
LightRAG employs two retrieval levels:
- Low-Level Retrieval: Focuses on specific entities and their attributes or connections. Retrieves detailed, specific data.
- High-Level Retrieval: Addresses broader concepts and themes. Gathers information spanning multiple entities, providing a comprehensive overview.
LightRAG vs. GraphRAG:
GraphRAG suffers from high token consumption and numerous LLM API calls due to its community-based traversal method. LightRAG, using vector-based search and retrieving entities/relationships instead of chunks, significantly reduces this overhead.
LightRAG Performance Benchmarks:
LightRAG was benchmarked against other RAG systems using GPT-4o-mini for evaluation across four domains (Agricultural, Computer Science, Legal, and Mixed). LightRAG consistently outperformed baselines, especially in diversity, particularly on the larger Legal dataset. This highlights its ability to generate varied and rich responses.
Hands-On Python Implementation (Google Colab):
The following steps outline a basic implementation using OpenAI models:
Step 1: Install Libraries
!pip install lightrag-hku aioboto3 tiktoken nano_vectordb !sudo apt update !sudo apt install -y pciutils !pip install langchain-ollama !curl -fsSL https://ollama.com/install.sh | sh !pip install ollama==0.4.2
Step 2: Import Libraries and Set API Key
from lightrag import LightRAG, QueryParam from lightrag.llm import gpt_4o_mini_complete import os os.environ['OPENAI_API_KEY'] = '' # Replace with your key import nest_asyncio nest_asyncio.apply()
Step 3: Initialize LightRAG and Load Data
WORKING_DIR = "./content" if not os.path.exists(WORKING_DIR): os.mkdir(WORKING_DIR) rag = LightRAG(working_dir=WORKING_DIR, llm_model_func=gpt_4o_mini_complete) with open("./Coffe.txt") as f: # Replace with your data file rag.insert(f.read())
Step 4 & 5: Querying (Hybrid and Naive Modes) (Examples provided in the original text)
Conclusion:
LightRAG significantly improves upon traditional RAG systems by addressing their limitations in handling complex relationships and contextual understanding. Its graph-based indexing and dual-level retrieval lead to more comprehensive and relevant responses, making it a valuable advancement in the field.
Key Takeaways:
- LightRAG overcomes traditional RAG's limitations in integrating interconnected information.
- Its dual-level retrieval system adapts to both specific and broad queries.
- Entity recognition and knowledge graph construction optimize information retrieval.
- The combination of graph structures and vector embeddings enhances contextual understanding.
Frequently Asked Questions: (Similar to the original text, but rephrased for conciseness) (This section would be included here, similar to the original.)
(Note: The image URLs remain unchanged.)
The above is the detailed content of LightRAG: Simple and Fast Alternative to GraphRAG. For more information, please follow other related articles on the PHP Chinese website!

Hot AI Tools

Undresser.AI Undress
AI-powered app for creating realistic nude photos

AI Clothes Remover
Online AI tool for removing clothes from photos.

Undress AI Tool
Undress images for free

Clothoff.io
AI clothes remover

AI Hentai Generator
Generate AI Hentai for free.

Hot Article

Hot Tools

Notepad++7.3.1
Easy-to-use and free code editor

SublimeText3 Chinese version
Chinese version, very easy to use

Zend Studio 13.0.1
Powerful PHP integrated development environment

Dreamweaver CS6
Visual web development tools

SublimeText3 Mac version
God-level code editing software (SublimeText3)

Hot Topics


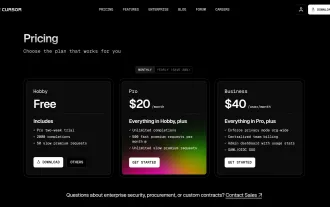
Vibe coding is reshaping the world of software development by letting us create applications using natural language instead of endless lines of code. Inspired by visionaries like Andrej Karpathy, this innovative approach lets dev
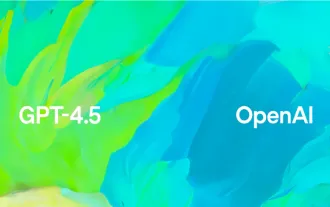
February 2025 has been yet another game-changing month for generative AI, bringing us some of the most anticipated model upgrades and groundbreaking new features. From xAI’s Grok 3 and Anthropic’s Claude 3.7 Sonnet, to OpenAI’s G
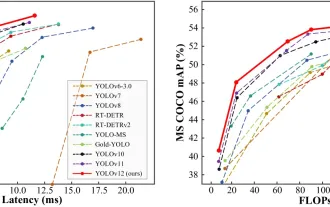
YOLO (You Only Look Once) has been a leading real-time object detection framework, with each iteration improving upon the previous versions. The latest version YOLO v12 introduces advancements that significantly enhance accuracy
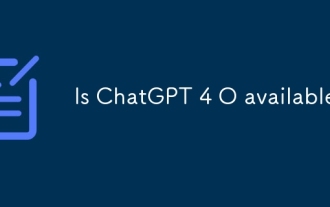
ChatGPT 4 is currently available and widely used, demonstrating significant improvements in understanding context and generating coherent responses compared to its predecessors like ChatGPT 3.5. Future developments may include more personalized interactions and real-time data processing capabilities, further enhancing its potential for various applications.
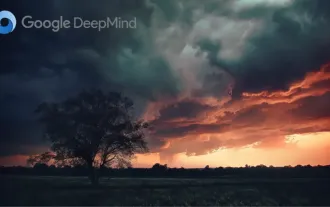
Google DeepMind's GenCast: A Revolutionary AI for Weather Forecasting Weather forecasting has undergone a dramatic transformation, moving from rudimentary observations to sophisticated AI-powered predictions. Google DeepMind's GenCast, a groundbreak
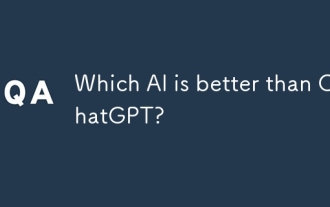
The article discusses AI models surpassing ChatGPT, like LaMDA, LLaMA, and Grok, highlighting their advantages in accuracy, understanding, and industry impact.(159 characters)
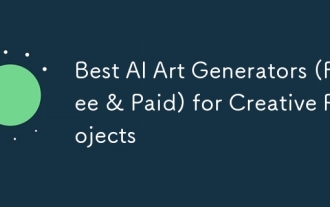
The article reviews top AI art generators, discussing their features, suitability for creative projects, and value. It highlights Midjourney as the best value for professionals and recommends DALL-E 2 for high-quality, customizable art.
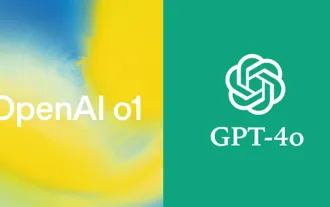
OpenAI's o1: A 12-Day Gift Spree Begins with Their Most Powerful Model Yet December's arrival brings a global slowdown, snowflakes in some parts of the world, but OpenAI is just getting started. Sam Altman and his team are launching a 12-day gift ex
