How Does Search-o1 Improve Logical Flow in AI Reasoning?
AI's rapid advancements are pushing the boundaries of machine capabilities, exceeding expectations from just a few years ago. Large Reasoning Models (LRMs, exemplified by OpenAI-o1) are sophisticated systems tackling complex problems through a step-by-step approach. These models don't just solve problems; they methodically reason, employing reinforcement learning to refine their logic and produce detailed, coherent solutions. This deliberate process, often called "slow thinking," enhances logical clarity. However, a significant limitation remains: knowledge gaps. LRMs can encounter uncertainties that propagate errors, compromising final accuracy. Traditional solutions like increasing model size and expanding datasets, while helpful, have limitations, and even Retrieval-Augmented Generation (RAG) methods struggle with highly complex reasoning.
Search-o1, a framework developed by researchers at Renmin University of China and Tsinghua University, addresses these limitations. It seamlessly integrates task instructions, questions, and dynamically retrieved knowledge into a cohesive reasoning chain, facilitating logical solutions. Search-o1 augments LRMs with an agentic RAG mechanism and a Reason-in-Documents module to refine retrieved information.
Table of Contents
- What is Search-o1?
- Traditional Reasoning
- Agentic RAG
- The Search-o1 Framework
- Search-o1 Performance Across Benchmarks
- Science QA (GPoQA)
- Mathematical Problems
- LiveCodeBench (Code Reasoning)
- Chemistry Case Study from the GPQA Dataset
- The Problem
- The Model's Strategy
- Reasoning and Solution
- Key Insights
- Conclusion
What is Search-o1?
Unlike traditional models that struggle with incomplete knowledge or basic RAG methods that often retrieve excessive, irrelevant information, Search-o1 introduces a crucial Reason-in-Documents module. This module distills extensive data into concise, logical steps, ensuring accuracy and coherence.
The framework operates iteratively, dynamically searching for and extracting relevant documents, transforming them into precise reasoning steps, and refining the process until a complete solution is obtained. It surpasses traditional reasoning (hampered by knowledge gaps) and basic RAG methods (which disrupt reasoning flow). Through an agentic mechanism for knowledge integration and maintaining coherence, Search-o1 ensures reliable and accurate reasoning, establishing a new standard for complex problem-solving in AI.
Search-o1 tackles knowledge gaps in LRMs by seamlessly integrating external knowledge retrieval without disrupting logical flow. The research compared three approaches: traditional reasoning, agentic RAG, and the Search-o1 framework.
1. Traditional Reasoning
Determining the number of carbon atoms in a three-step chemical reaction's final product serves as an example. Traditional methods struggle when encountering knowledge gaps, such as lacking the structure of trans-Cinnamaldehyde. Without accurate information, the model relies on assumptions, potentially leading to errors.
2. Agentic RAG
Agentic RAG allows autonomous knowledge retrieval. If uncertain about a compound's structure, it generates specific queries (e.g., "structure of trans-Cinnamaldehyde"). However, directly incorporating lengthy, often irrelevant retrieved documents disrupts the reasoning process and reduces coherence due to verbose and tangential information.
3. Search-o1
Search-o1 enhances agentic RAG with the Reason-in-Documents module. This module refines retrieved documents into concise reasoning steps, seamlessly integrating external knowledge while preserving logical flow. Considering the current query, retrieved documents, and the evolving reasoning chain, it generates coherent, interconnected steps iteratively until a conclusive answer is reached.
Search-o1 Performance Across Benchmarks
Three challenging reasoning tasks were evaluated:
- Advanced Science QA (PhD-level questions in Physics, Chemistry, Biology),
- Complex Mathematical Problems (difficult problems from MATH500 and AMC23),
- Live Coding Challenges (real-world coding tasks categorized by difficulty).
1. Science QA (GPoQA)
- Direct Reasoning (No Retrieval): Models like Qwen2.5-32B (57.0%) and QwQ-32B (68.4%) lagged behind Search-o1 (77.9%).
- Retrieval-Augmented Reasoning: RAG-QwQ-32B (76.7%) performed well but still fell short of Search-o1's accuracy. Search-o1 demonstrated superior performance in Physics (78.9%) and Chemistry (47.3%).
2. Math Benchmarks
- Direct Reasoning: QwQ-32B (83.2%) performed best among direct methods, but Search-o1 (86.4%) surpassed it.
- Retrieval-Augmented Reasoning: RAG-QwQ-32B (85.0%) was close, but Search-o1 maintained a lead, highlighting the benefit of its structured reasoning.
3. LiveCodeBench (Code Reasoning)
- Direct Reasoning: Qwen2.5-Coder-32B (22.5%) and QwQ-32B (33.0%) were outperformed by Search-o1 (33.0%).
- Retrieval-Augmented Reasoning: RAG methods significantly underperformed compared to Search-o1.
Key Findings:
- Superior Performance: Search-o1 consistently outperformed other methods due to its iterative reasoning approach.
- Reason-in-Documents Module's Impact: This module ensured focused reasoning, providing an advantage over direct and RAG approaches.
- Robustness: While some methods excelled in specific tasks, Search-o1 demonstrated balanced performance across all categories.
Search-o1 proved the most effective method across all tasks, setting a new standard by combining retrieval and structured reasoning. The framework addresses knowledge insufficiency by integrating RAG with the Reason-in-Documents module, enabling more effective use of external knowledge. This forms a strong foundation for future research in retrieval systems, document analysis, and intelligent problem-solving.
Chemistry Case Study from the GPQA Dataset
This case study illustrates how Search-o1 answers a chemistry question from the GPQA dataset using retrieval-augmented reasoning.
The Problem
Determine the number of carbon atoms in the final product of a multi-step reaction involving trans-cinnamaldehyde.
The Model's Strategy
- Problem Decomposition: The model analyzed the reaction step-by-step, identifying key components and how carbon atoms are added.
- External Knowledge Retrieval: The model queried information about reaction mechanisms, retrieving data on Grignard reagent reactions with aldehydes and the structure of trans-cinnamaldehyde.
- Subsequent Reaction Analysis: The model tracked carbon atom changes throughout each reaction step.
- Initial Structure Verification: The model verified the initial carbon atom count in trans-cinnamaldehyde.
- Final Reaction Analysis: The model analyzed the final reaction, determining the total carbon atoms in the final product.
Reasoning and Solution
The model concluded that the final product contains 11 carbon atoms (starting with 9, adding one from the Grignard reaction, and another in the final step). The answer is 11.
Key Insights
- Effective Knowledge Use: Targeted searches filled knowledge gaps.
- Iterative Reasoning: Methodical step-by-step analysis ensured accuracy.
- Error Checking: The model re-evaluated assumptions, ensuring accuracy.
Conclusion
Search-o1 represents a significant advancement in LRMs, addressing knowledge insufficiency. By integrating agentic RAG and the Reason-in-Documents module, it enables seamless, iterative reasoning that incorporates external knowledge while maintaining logical coherence. Its superior performance across diverse domains sets a new standard for complex problem-solving in AI. This innovation enhances reasoning accuracy and opens avenues for research in retrieval systems, document analysis, and intelligent problem-solving, bridging the gap between knowledge retrieval and logical reasoning. Search-o1 establishes a robust foundation for the future of AI, enabling more effective solutions to complex challenges.
The above is the detailed content of How Does Search-o1 Improve Logical Flow in AI Reasoning?. For more information, please follow other related articles on the PHP Chinese website!

Hot AI Tools

Undresser.AI Undress
AI-powered app for creating realistic nude photos

AI Clothes Remover
Online AI tool for removing clothes from photos.

Undress AI Tool
Undress images for free

Clothoff.io
AI clothes remover

AI Hentai Generator
Generate AI Hentai for free.

Hot Article

Hot Tools

Notepad++7.3.1
Easy-to-use and free code editor

SublimeText3 Chinese version
Chinese version, very easy to use

Zend Studio 13.0.1
Powerful PHP integrated development environment

Dreamweaver CS6
Visual web development tools

SublimeText3 Mac version
God-level code editing software (SublimeText3)

Hot Topics


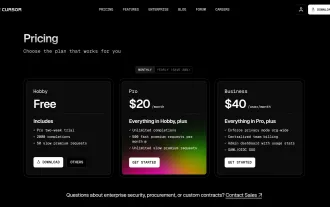
Vibe coding is reshaping the world of software development by letting us create applications using natural language instead of endless lines of code. Inspired by visionaries like Andrej Karpathy, this innovative approach lets dev
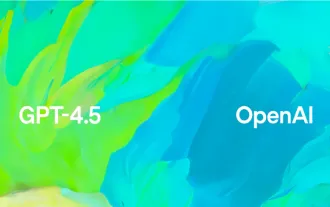
February 2025 has been yet another game-changing month for generative AI, bringing us some of the most anticipated model upgrades and groundbreaking new features. From xAI’s Grok 3 and Anthropic’s Claude 3.7 Sonnet, to OpenAI’s G
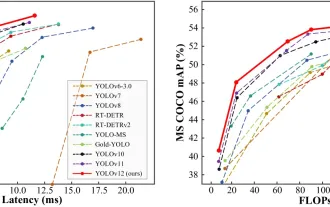
YOLO (You Only Look Once) has been a leading real-time object detection framework, with each iteration improving upon the previous versions. The latest version YOLO v12 introduces advancements that significantly enhance accuracy
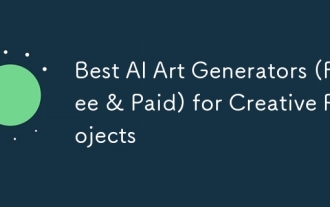
The article reviews top AI art generators, discussing their features, suitability for creative projects, and value. It highlights Midjourney as the best value for professionals and recommends DALL-E 2 for high-quality, customizable art.
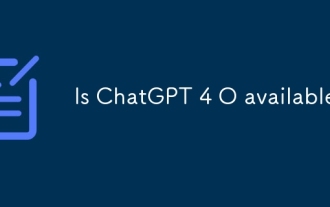
ChatGPT 4 is currently available and widely used, demonstrating significant improvements in understanding context and generating coherent responses compared to its predecessors like ChatGPT 3.5. Future developments may include more personalized interactions and real-time data processing capabilities, further enhancing its potential for various applications.
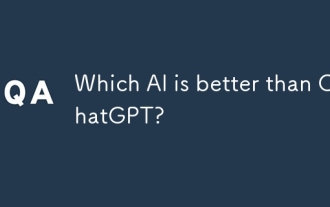
The article discusses AI models surpassing ChatGPT, like LaMDA, LLaMA, and Grok, highlighting their advantages in accuracy, understanding, and industry impact.(159 characters)
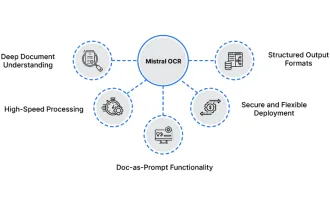
Mistral OCR: Revolutionizing Retrieval-Augmented Generation with Multimodal Document Understanding Retrieval-Augmented Generation (RAG) systems have significantly advanced AI capabilities, enabling access to vast data stores for more informed respons
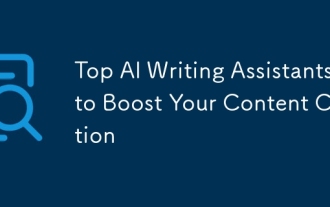
The article discusses top AI writing assistants like Grammarly, Jasper, Copy.ai, Writesonic, and Rytr, focusing on their unique features for content creation. It argues that Jasper excels in SEO optimization, while AI tools help maintain tone consist
