OpenAI Function Calling Tutorial: Generate Structured Output
Using OpenAI Without Function Calling
In this section, we will generate responses using the GPT-3.5-Turbo model without function calling to see if we get consistent output or not.
Before installing the OpenAI Python API, you must obtain an API key and set it up on your local system. Follow the GPT-3.5 and GPT-4 via the OpenAI API in Python tutorial to learn how to get the API key and set it up. The tutorial also includes examples of setting up environment variables in DataLab, DataCamp's AI-enabled data notebook.
For further assistance, check out the code in OpenAI Function Calling workbook on DataLab.
Upgrade the OpenAI Python API to V1 using:
pip install --upgrade openai -q
After that, initiate the OpenAI client using the API key.
import os from openai import OpenAI client = OpenAI( api_key=os.environ['OPENAI_API_KEY'], )
Note: OpenAI no longer offers free credits to new users, so you have to buy them to use the API.
We will write a random student description. Either you can come up with your own text or use ChatGPT to generate one for you.
student_1_description = "David Nguyen is a sophomore majoring in computer science at Stanford University. He is Asian American and has a 3.8 GPA. David is known for his programming skills and is an active member of the university's Robotics Club. He hopes to pursue a career in artificial intelligence after graduating."
In the next part, we will write a prompt to extract student information from the text and return the output as a JSON object. We will extract the name, major, school, grades, and clubs in the student description.
# A simple prompt to extract information from "student_description" in a JSON format. prompt1 = f''' Please extract the following information from the given text and return it as a JSON object: name major school grades club This is the body of text to extract the information from: {student_1_description} '''
Add the prompt to the OpenAI API chat completion module to generate the response.
# Generating response back from gpt-3.5-turbo openai_response = client.chat.completions.create( model = 'gpt-3.5-turbo', messages = [{'role': 'user', 'content': prompt_1}] ) openai_response.choices[0].message.content
The response is quite good. Let’s convert it into JSON to understand it better.
'{\n "name": "David Nguyen",\n "major": "computer science",\n "school": "Stanford University",\n "grades": "3.8 GPA",\n "club": "Robotics Club"\n}'
We will use the `json` library to convert the text into a JSON object.
import json # Loading the response as a JSON object json_response = json.loads(openai_response.choices[0].message.content) json_response
The final result is pretty much perfect. So, why do we need Function Calling?
{'name': 'David Nguyen', 'major': 'computer science', 'school': 'Stanford University', 'grades': '3.8 GPA', 'club': 'Robotics Club'}
Let’s try the same prompt, but using a different student description.
student_2_description="Ravi Patel is a sophomore majoring in computer science at the University of Michigan. He is South Asian Indian American and has a 3.7 GPA. Ravi is an active member of the university's Chess Club and the South Asian Student Association. He hopes to pursue a career in software engineering after graduating."
We will just change the student description text in the prompt.
prompt2 = f''' Please extract the following information from the given text and return it as a JSON object: name major school grades club This is the body of text to extract the information from: {student_2_description} '''
And, run the chat completion function using the second prompt.
# Generating response back from gpt-3.5-turbo openai_response = client.chat.completions.create( model = 'gpt-3.5-turbo', messages = [{'role': 'user', 'content': prompt_2}] ) # Loading the response as a JSON object json_response = json.loads(openai_response.choices[0].message.content) json_response
As you can see, it is not consistent. Instead of returning one club, it has returned the list of clubs joined by Ravi. It is also different from the first student.
{'name': 'Ravi Patel', 'major': 'computer science', 'school': 'University of Michigan', 'grades': '3.7 GPA', 'club': ['Chess Club', 'South Asian Student Association']}
OpenAI Function Calling Example
To resolve this issue, we will now use a recently introduced feature called Function Calling. It is essential to create a custom function to add necessary information to a list of dictionaries so that the OpenAI API can understand its functionality.
- name: write the Python function name that you have recently created.
- description: the functionality of the function.
- parameters: within the “properties”, we will write the name of the arguments, type, and description. It will help OpenAI API to identify the world that we are looking for.
Note: Make sure you are following the correct pattern. Learn more about function calling by reading the official documentation.
pip install --upgrade openai -q
Next, we will generate responses for two student descriptions using a custom function added to the "functions" argument. After that, we will convert the text response into a JSON object and print it.
import os from openai import OpenAI client = OpenAI( api_key=os.environ['OPENAI_API_KEY'], )
As we can see, we got uniform output. We even got grades in numeric instead of string. Consistent output is essential for creating bug-free AI applications.
student_1_description = "David Nguyen is a sophomore majoring in computer science at Stanford University. He is Asian American and has a 3.8 GPA. David is known for his programming skills and is an active member of the university's Robotics Club. He hopes to pursue a career in artificial intelligence after graduating."
Multiple Custom Functions
You can add multiple custom functions to the chat completion function. In this section, we will see the magical capabilities of OpenAI API and how it automatically selects the correct function and returns the right arguments.
In the Python list of the dictionary, we will add another function called “extract_school_info,” which will help us extract university information from the text.
To achieve this, you have to add another dictionary of a function with name, description, and parameters.
# A simple prompt to extract information from "student_description" in a JSON format. prompt1 = f''' Please extract the following information from the given text and return it as a JSON object: name major school grades club This is the body of text to extract the information from: {student_1_description} '''
We will generate a “Stanford University” description using ChatGPT to test our function.
# Generating response back from gpt-3.5-turbo openai_response = client.chat.completions.create( model = 'gpt-3.5-turbo', messages = [{'role': 'user', 'content': prompt_1}] ) openai_response.choices[0].message.content
Create the list of student and school descriptions and pass it through the OpenAI chat completion function to generate the response. Make sure you have provided the updated custom function.
'{\n "name": "David Nguyen",\n "major": "computer science",\n "school": "Stanford University",\n "grades": "3.8 GPA",\n "club": "Robotics Club"\n}'
The GPT-3.5-Turbo model has automatically selected the correct function for different description types. We get perfect JSON output for the student and the school.
import json # Loading the response as a JSON object json_response = json.loads(openai_response.choices[0].message.content) json_response
We can even look under the name that the repose is generated using the “extract_school_info” function.
Applications of Function Calling
In this section, we will build a stable text summarizer that will summarize the school and student information in a certain way.
First, we will create two Python functions, extract_student_info and extract_school_info, that take the arguments from function calling and return a summarized string.
pip install --upgrade openai -q
- Create the Python list, which consists of student one description, random prompt, and school one description. The random prompt is added to validate the automatic function calling mechanic.
- We will generate the response using each text in the `descriptions` list.
- If a function call is used, we will get the name of the function and, based on it, apply the relevant arguments to the function using the response. Otherwise, return the normal response.
- Print the outputs of all three samples.
import os from openai import OpenAI client = OpenAI( api_key=os.environ['OPENAI_API_KEY'], )
- Sample#1: The GPT model has selected “extract_student_info,” and we got a short summary about the student.
- Sample#2: The GPT model has not selected any function and treated the prompt as a regular question, and as a result, we got the biography of Abraham Lincoln.
- Sample#3: The GPT model has selected “extract_school_info,” and we got a short summary about Stanford University.
student_1_description = "David Nguyen is a sophomore majoring in computer science at Stanford University. He is Asian American and has a 3.8 GPA. David is known for his programming skills and is an active member of the university's Robotics Club. He hopes to pursue a career in artificial intelligence after graduating."
Conclusion
In this tutorial, we learned about OpenAI's function calling. We also learned how to use it to generate consistent outputs, create multiple functions, and build a reliable text summarizer.
If you want to learn more about the OpenAI API, consider taking the Working with OpenAI API course and using the OpenAI API in Python cheat sheet to create your first AI-powered project.
FAQs
How does OpenAI function calling handle complex nested JSON outputs?
OpenAI function calling allows you to define nested JSON structures in the function schema. By specifying the hierarchical relationships within the parameters property, you can ensure the model generates properly nested and structured JSON outputs for complex data requirements.
Can OpenAI function calling be used with external APIs or databases?
Yes, OpenAI function calling can be integrated with external APIs or databases by creating custom functions that execute API calls or database queries based on the arguments passed from the model. This enables dynamic interactions with external systems while maintaining consistent and structured responses.
What happens if the model’s function call doesn’t match any defined functions?
If the model’s function call doesn’t match a defined function or the provided schema, the function call is not triggered, and the model treats the input as a standard text-based prompt, returning a typical text-based response instead. This ensures flexibility in handling varied input types.
Earn a Top AI Certification
Demonstrate you can effectively and responsibly use AI.Get Certified, Get HiredThe above is the detailed content of OpenAI Function Calling Tutorial: Generate Structured Output. For more information, please follow other related articles on the PHP Chinese website!

Hot AI Tools

Undresser.AI Undress
AI-powered app for creating realistic nude photos

AI Clothes Remover
Online AI tool for removing clothes from photos.

Undress AI Tool
Undress images for free

Clothoff.io
AI clothes remover

AI Hentai Generator
Generate AI Hentai for free.

Hot Article

Hot Tools

Notepad++7.3.1
Easy-to-use and free code editor

SublimeText3 Chinese version
Chinese version, very easy to use

Zend Studio 13.0.1
Powerful PHP integrated development environment

Dreamweaver CS6
Visual web development tools

SublimeText3 Mac version
God-level code editing software (SublimeText3)

Hot Topics


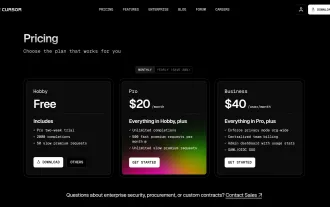
Vibe coding is reshaping the world of software development by letting us create applications using natural language instead of endless lines of code. Inspired by visionaries like Andrej Karpathy, this innovative approach lets dev
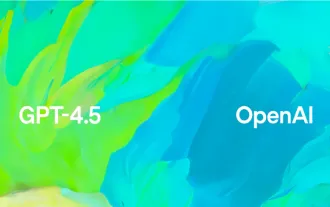
February 2025 has been yet another game-changing month for generative AI, bringing us some of the most anticipated model upgrades and groundbreaking new features. From xAI’s Grok 3 and Anthropic’s Claude 3.7 Sonnet, to OpenAI’s G
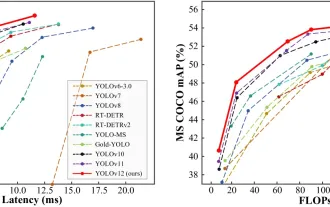
YOLO (You Only Look Once) has been a leading real-time object detection framework, with each iteration improving upon the previous versions. The latest version YOLO v12 introduces advancements that significantly enhance accuracy
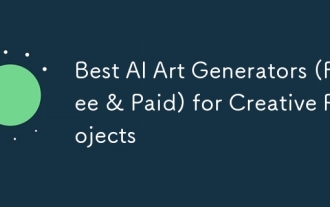
The article reviews top AI art generators, discussing their features, suitability for creative projects, and value. It highlights Midjourney as the best value for professionals and recommends DALL-E 2 for high-quality, customizable art.
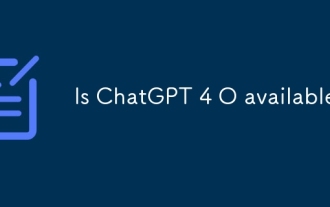
ChatGPT 4 is currently available and widely used, demonstrating significant improvements in understanding context and generating coherent responses compared to its predecessors like ChatGPT 3.5. Future developments may include more personalized interactions and real-time data processing capabilities, further enhancing its potential for various applications.
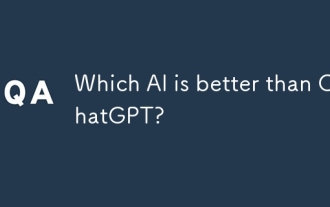
The article discusses AI models surpassing ChatGPT, like LaMDA, LLaMA, and Grok, highlighting their advantages in accuracy, understanding, and industry impact.(159 characters)
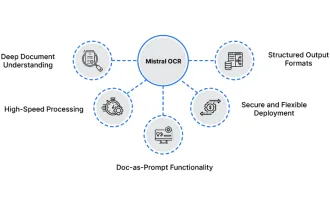
Mistral OCR: Revolutionizing Retrieval-Augmented Generation with Multimodal Document Understanding Retrieval-Augmented Generation (RAG) systems have significantly advanced AI capabilities, enabling access to vast data stores for more informed respons
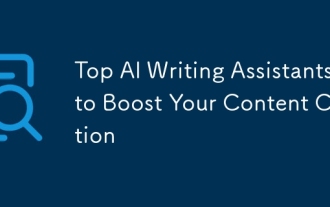
The article discusses top AI writing assistants like Grammarly, Jasper, Copy.ai, Writesonic, and Rytr, focusing on their unique features for content creation. It argues that Jasper excels in SEO optimization, while AI tools help maintain tone consist
