Weaviate Tutorial: Unlocking the Power of Vector Search
Weaviate: Your Open-Source Vector Search Engine for Unstructured Data
Weaviate is a powerful, open-source vector search engine designed for handling unstructured data like text, images, and audio. This tutorial will guide you through its core concepts, setup, data management, and querying using its intuitive GraphQL interface. We'll also explore Python integration and best practices for optimal performance.
What is Weaviate?
Weaviate uniquely combines object storage with vector search, enabling powerful similarity-based querying. Its cloud-native and real-time architecture ensures scalability, while optional modules cater to various data types (text, image, etc.). This modularity allows for customization based on your specific needs.
Understanding Embeddings and Vector Databases
Traditional databases struggle with unstructured data. Weaviate solves this by leveraging embeddings – numerical representations of unstructured data generated by machine learning models. These embeddings allow for efficient similarity comparisons, crucial for tasks like semantic search and question answering. Vector databases, unlike traditional relational databases, are optimized for storing and querying these vector representations.
Text Embedding Model - Image Source
Image Source
Pinecone offers a compelling alternative; explore our "Mastering Vector Databases with Pinecone" tutorial for more details.
Setting Up Weaviate
Weaviate is easily installed via Docker Compose (recommended) or manual installation. After installation, verify functionality with a simple query. For Docker Compose:
docker compose up -d
Other deployment options include Weaviate's managed cloud service and the AWS marketplace. The Python client is installed using pip:
pip install -U weaviate-client
Core Weaviate Concepts
- Data Objects: Data items organized into "Classes," each with defined "Properties." Each object is represented by a vector.
- Modules: Extend Weaviate's functionality (vectorization, backups, etc.). The core engine functions as a vector database even without modules.
- Storage: Provides real-time, reliable data persistence with robust handling of failures. Queries return complete data objects, not just IDs. Filtering happens before vector search for precise result counts.
- GraphQL: Weaviate's primary interface, offering efficient and flexible data access compared to REST. Its graph-based nature excels with cross-referenced data.
Image Source
Creating Classes and Objects
Classes define the structure of your data. You can create them manually or use Weaviate's autoschema feature. Here's a Python example of manual class creation:
docker compose up -d
A more comprehensive example, including vectorization and properties:
pip install -U weaviate-client
Objects are added to classes. Vectors can be explicitly provided or generated by Weaviate.
class_name = "Item description" class_object = {"class": class_name} client.schema.create_class(class_object)
End-to-End Python Example
This example demonstrates creating a class and adding objects using the Python client. Remember to install the client (pip install -U weaviate-client
) and have a running Weaviate instance (cloud or embedded).
{ "class": "Article", "vectorizer": "text2vec-cohere", "vectorIndexConfig": { "distance": "cosine" }, "moduleConfig": { "generative-openai": {} }, "properties": [ // ... property definitions ... ] }
Data Source (Credit: Weaviate Official)
Weaviate Best Practices
- Schema Design: Flexible, scalable schemas with unique identifiers and semantic types.
- Data Import: Utilize batch import for efficiency and REST APIs for automation. Use supported formats (JSON, CSV).
- Query Optimization: Leverage GraphQL, pagination, and caching for optimal performance.
ChromaDB provides another excellent open-source vector database option; explore our ChromaDB tutorial for more information.
Conclusion
Weaviate offers a robust and flexible solution for managing and querying unstructured data. Its vector-based approach, combined with its user-friendly GraphQL interface and Python client, makes it an ideal choice for various machine learning and AI applications. Consider exploring our webinar on "Vector Databases for Data Science with Weaviate in Python" to further enhance your knowledge.
The above is the detailed content of Weaviate Tutorial: Unlocking the Power of Vector Search. For more information, please follow other related articles on the PHP Chinese website!

Hot AI Tools

Undresser.AI Undress
AI-powered app for creating realistic nude photos

AI Clothes Remover
Online AI tool for removing clothes from photos.

Undress AI Tool
Undress images for free

Clothoff.io
AI clothes remover

AI Hentai Generator
Generate AI Hentai for free.

Hot Article

Hot Tools

Notepad++7.3.1
Easy-to-use and free code editor

SublimeText3 Chinese version
Chinese version, very easy to use

Zend Studio 13.0.1
Powerful PHP integrated development environment

Dreamweaver CS6
Visual web development tools

SublimeText3 Mac version
God-level code editing software (SublimeText3)

Hot Topics


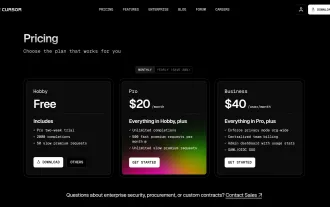
Vibe coding is reshaping the world of software development by letting us create applications using natural language instead of endless lines of code. Inspired by visionaries like Andrej Karpathy, this innovative approach lets dev
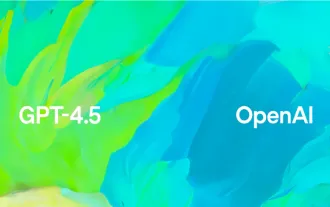
February 2025 has been yet another game-changing month for generative AI, bringing us some of the most anticipated model upgrades and groundbreaking new features. From xAI’s Grok 3 and Anthropic’s Claude 3.7 Sonnet, to OpenAI’s G
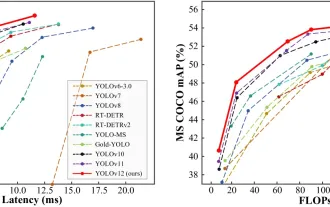
YOLO (You Only Look Once) has been a leading real-time object detection framework, with each iteration improving upon the previous versions. The latest version YOLO v12 introduces advancements that significantly enhance accuracy
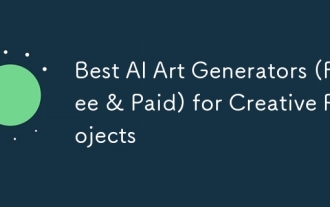
The article reviews top AI art generators, discussing their features, suitability for creative projects, and value. It highlights Midjourney as the best value for professionals and recommends DALL-E 2 for high-quality, customizable art.
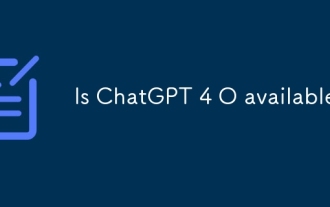
ChatGPT 4 is currently available and widely used, demonstrating significant improvements in understanding context and generating coherent responses compared to its predecessors like ChatGPT 3.5. Future developments may include more personalized interactions and real-time data processing capabilities, further enhancing its potential for various applications.
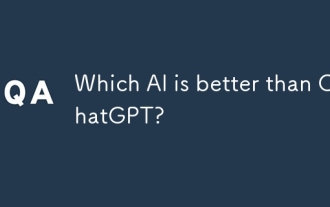
The article discusses AI models surpassing ChatGPT, like LaMDA, LLaMA, and Grok, highlighting their advantages in accuracy, understanding, and industry impact.(159 characters)
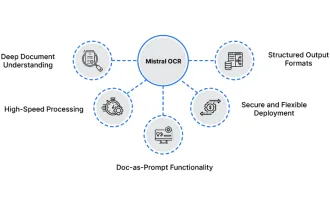
Mistral OCR: Revolutionizing Retrieval-Augmented Generation with Multimodal Document Understanding Retrieval-Augmented Generation (RAG) systems have significantly advanced AI capabilities, enabling access to vast data stores for more informed respons
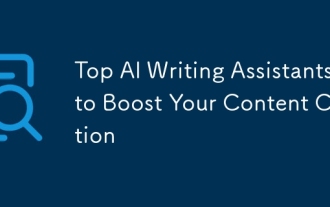
The article discusses top AI writing assistants like Grammarly, Jasper, Copy.ai, Writesonic, and Rytr, focusing on their unique features for content creation. It argues that Jasper excels in SEO optimization, while AI tools help maintain tone consist
