How to Use Python Generators for Memory Efficiency?
Python generators enhance memory efficiency by yielding values on demand, unlike lists which load all data at once. This is crucial for large datasets, preventing memory errors and improving performance. Generators are ideal for processing data str
How to Use Python Generators for Memory Efficiency?
Python generators are a powerful tool for improving memory efficiency, especially when dealing with large datasets. They achieve this by producing values one at a time, on demand, instead of creating the entire dataset in memory at once. This is done using the yield
keyword instead of return
within a function. A generator function doesn't return a value directly; instead, it returns a generator object. This object can then be iterated over, producing each value as needed.
Let's illustrate with an example. Suppose you want to generate a sequence of numbers from 1 to 10,000,000. A list-based approach would consume significant memory:
my_list = list(range(10000000)) # Consumes a lot of memory
A generator-based approach, however, is far more memory-efficient:
def my_generator(): for i in range(10000000): yield i my_gen = my_generator() # Creates a generator object; no memory consumed yet for num in my_gen: # Process each number individually. Only one number is in memory at a time. print(num) #This will print numbers one by one. You can replace this with your processing logic.
The key difference lies in when the values are generated. The list approach creates all 10 million numbers immediately. The generator approach creates each number only when it's requested during iteration. This lazy evaluation is the core of a generator's memory efficiency. You can also use generator expressions for concise generator creation:
my_gen_expression = (i for i in range(10000000)) #Similar to above, but more concise for num in my_gen_expression: print(num)
What are the key advantages of using generators over lists in Python for large datasets?
The primary advantage of generators over lists for large datasets is memory efficiency. Lists store all their elements in memory simultaneously, leading to high memory consumption for large datasets that might exceed available RAM. Generators, on the other hand, generate values on demand, keeping memory usage minimal. This prevents MemoryError
exceptions and allows processing of datasets far larger than available RAM.
Beyond memory efficiency, generators also offer:
- Improved performance: Because generators don't need to generate all values upfront, they can often be faster, especially when only a portion of the data is needed. The time spent creating unnecessary elements is saved.
- Code clarity: For complex data transformations, generators can lead to more readable and maintainable code by breaking down the process into smaller, manageable steps.
- Infinite sequences: Generators can easily represent infinite sequences, which is impossible with lists. For instance, a generator can produce prime numbers indefinitely.
How can I improve the performance of my Python code by leveraging generators to handle memory-intensive tasks?
Leveraging generators to improve performance in memory-intensive tasks involves strategically replacing list comprehensions or loops that create large lists in memory with generator expressions or generator functions. This reduces the memory footprint and can significantly speed up processing, especially for I/O-bound tasks.
Consider a scenario where you need to process a large file line by line:
Inefficient (using lists):
with open("large_file.txt", "r") as f: lines = f.readlines() # Reads entire file into memory processed_lines = [line.strip().upper() for line in lines] # Processes the entire list in memory
Efficient (using generators):
def process_file(filename): with open(filename, "r") as f: for line in f: yield line.strip().upper() for processed_line in process_file("large_file.txt"): # Process each line individually print(processed_line)
The generator version processes each line individually as it's read from the file, avoiding loading the entire file into memory. This is crucial for files much larger than available RAM. Similarly, you can apply this principle to other memory-intensive operations like database queries or network requests where you process results iteratively rather than loading everything at once.
When is it most beneficial to employ Python generators to optimize memory usage in my applications?
Python generators are most beneficial when:
-
Dealing with extremely large datasets: When the data size exceeds available RAM, generators are essential to avoid
MemoryError
exceptions. - Processing data streams: When working with continuous data streams (e.g., network data, sensor readings), generators provide an efficient way to process data as it arrives without buffering the entire stream.
- Performing iterative calculations: When performing calculations on a sequence where the result of one step depends on the previous one, generators can be used to avoid storing intermediate results in memory.
- Improving code readability: For complex data transformations, generators can simplify the code by breaking down the process into smaller, more manageable steps, leading to improved maintainability.
- Creating infinite sequences: Generators are the only practical way to represent and work with infinite sequences in Python.
In essence, anytime you find yourself working with data that might not fit comfortably in memory, or where lazy evaluation can improve performance, Python generators should be a strong consideration. They provide a powerful and efficient way to handle large datasets and streaming data, significantly enhancing the performance and scalability of your applications.
The above is the detailed content of How to Use Python Generators for Memory Efficiency?. For more information, please follow other related articles on the PHP Chinese website!

Hot AI Tools

Undresser.AI Undress
AI-powered app for creating realistic nude photos

AI Clothes Remover
Online AI tool for removing clothes from photos.

Undress AI Tool
Undress images for free

Clothoff.io
AI clothes remover

Video Face Swap
Swap faces in any video effortlessly with our completely free AI face swap tool!

Hot Article

Hot Tools

Notepad++7.3.1
Easy-to-use and free code editor

SublimeText3 Chinese version
Chinese version, very easy to use

Zend Studio 13.0.1
Powerful PHP integrated development environment

Dreamweaver CS6
Visual web development tools

SublimeText3 Mac version
God-level code editing software (SublimeText3)

Hot Topics
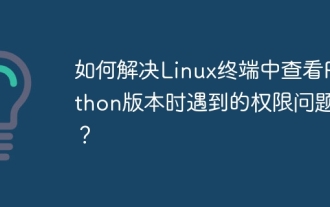
Solution to permission issues when viewing Python version in Linux terminal When you try to view Python version in Linux terminal, enter python...
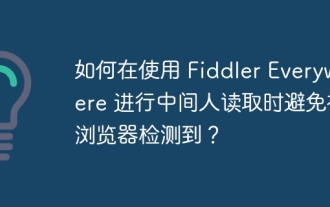
How to avoid being detected when using FiddlerEverywhere for man-in-the-middle readings When you use FiddlerEverywhere...
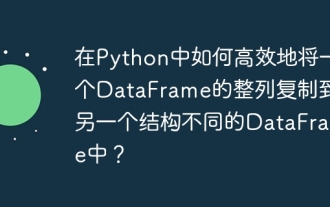
When using Python's pandas library, how to copy whole columns between two DataFrames with different structures is a common problem. Suppose we have two Dats...
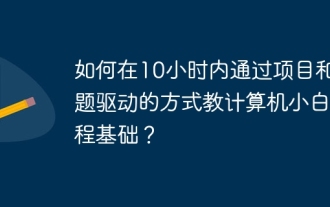
How to teach computer novice programming basics within 10 hours? If you only have 10 hours to teach computer novice some programming knowledge, what would you choose to teach...
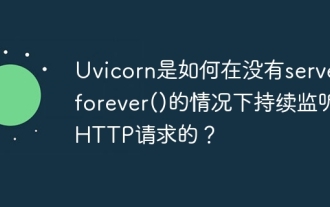
How does Uvicorn continuously listen for HTTP requests? Uvicorn is a lightweight web server based on ASGI. One of its core functions is to listen for HTTP requests and proceed...
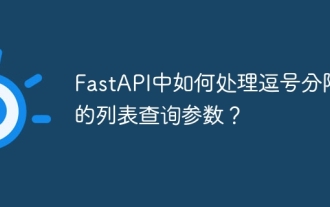
Fastapi ...
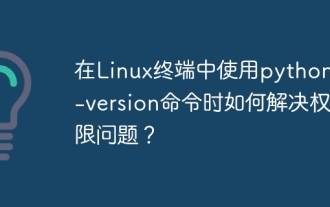
Using python in Linux terminal...
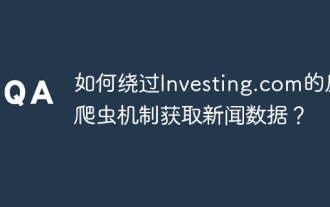
Understanding the anti-crawling strategy of Investing.com Many people often try to crawl news data from Investing.com (https://cn.investing.com/news/latest-news)...
