How do I use MongoDB's query operators for advanced filtering?
This article explains MongoDB's advanced query operators, enabling complex data filtering beyond simple equality checks. It details operators like $eq, $ne, $gt, $in, $regex, and their combinations for efficient data retrieval, aggregation, and vali
How to Use MongoDB's Query Operators for Advanced Filtering?
MongoDB offers a rich set of query operators that go beyond simple equality checks, enabling powerful and flexible data filtering. These operators allow you to specify complex criteria for selecting documents from your collections. Here's a breakdown of how to use them:
1. Understanding the Basic Syntax: MongoDB queries use a JSON-like structure. The core element is a query document that contains key-value pairs. The keys represent the fields you want to filter, and the values specify the conditions.
2. Essential Operators:
-
$eq
(Equality): Matches documents where the field value is equal to the specified value. e.g.,{"age": {"$eq": 30}}
-
$ne
(Not Equal): Matches documents where the field value is not equal to the specified value. e.g.,{"city": {"$ne": "New York"}}
-
$gt
,$gte
,$lt
,$lte
(Comparison Operators): Greater than, greater than or equal to, less than, less than or equal to. e.g.,{"price": {"$gt": 100}}
-
$in
,$nin
(Inclusion/Exclusion): Matches documents where the field value is in (or not in) an array of values. e.g.,{"status": {"$in": ["active", "pending"]}}
-
$regex
(Regular Expressions): Matches documents where the field value matches a regular expression pattern. e.g.,{"name": {"$regex": /^John/}}
(matches names starting with "John") -
$exists
: Checks if a field exists in a document. e.g.,{"address": {"$exists": true}}
-
$type
: Matches documents based on the BSON type of a field. Useful for data validation.
3. Combining Operators: You can combine multiple operators within a single query document to create complex filtering logic. MongoDB will apply these conditions conjunctively (using AND). For OR conditions, use the $or
operator:
db.collection.find( { $or: [ { age: { $gt: 30 } }, { city: "London" } ] } )
4. Using the MongoDB Shell or Driver: These operators are used within the find()
method of your chosen MongoDB driver (e.g., pymongo for Python, the MongoDB shell).
What Are Some Common Use Cases for MongoDB's Advanced Query Operators?
MongoDB's advanced query operators are crucial for a wide variety of data filtering and manipulation tasks. Here are some common use cases:
- Targeted Data Retrieval: Quickly find specific documents based on complex criteria, such as finding all users with an age between 25 and 35 who live in a particular city and have a specific subscription status. This avoids retrieving and processing the entire dataset.
- Data Aggregation and Analysis: Advanced operators are essential for building aggregation pipelines. For instance, you can use
$match
(to filter data) with$group
(to group documents) and$sum
(to perform calculations) to analyze sales data by region or product. - Implementing Business Rules: Enforce business logic within your queries. For example, you might use
$regex
to validate email addresses or$type
to ensure data integrity. - Real-time Filtering and Search: In applications with dynamic filtering needs, like e-commerce sites or search engines, advanced operators provide the flexibility to refine search results based on user input.
- Data Validation and Cleaning: Identify and correct inconsistent or erroneous data within your collection. For example, you can use
$exists
to find documents missing critical fields.
Can I Use MongoDB's Query Operators to Filter Data Based on Nested Documents?
Yes, MongoDB's query operators work seamlessly with nested documents. To filter based on fields within nested documents, you use dot notation to specify the path to the nested field.
For example, consider documents with the structure:
{ "user": { "name": "Alice", "address": { "city": "New York", "zip": "10001" } } }
To find all documents where the city is "New York," you would use:
db.collection.find( { "user.address.city": "New York" } )
For more complex nested filtering, you can combine dot notation with other operators:
db.collection.find( { "user.address.zip": { $regex: /^100/ } } ) // Find documents where zip code starts with "100"
You can also use the $elemMatch
operator to filter arrays of embedded documents. This allows you to specify conditions that must be met by at least one element within the array.
How Do I Optimize My MongoDB Queries Using Advanced Operators for Better Performance?
Optimizing MongoDB queries using advanced operators involves several strategies:
-
Indexing: Proper indexing is paramount. Create indexes on fields frequently used in
$match
stages of aggregations orfind()
queries. Compound indexes can speed up queries involving multiple fields. -
Selective Field Retrieval: Use the
projection
parameter in yourfind()
queries to retrieve only the necessary fields. This reduces the amount of data transferred from the database, improving performance. -
Avoid
$or
with Unindexed Fields: Queries using$or
can be slow if the fields involved are not indexed. Consider alternative approaches, such as multiple queries or creating separate indexes. -
Limit Data Returned: Use the
limit()
method to restrict the number of documents returned. This is particularly important for large datasets. -
Efficient Operator Usage: Choose the most appropriate operator for the task. For example, using
$in
with a small array is generally more efficient than multiple$or
conditions. -
Analyze Query Execution Plans: Use
explain()
to analyze the execution plan of your queries. This helps identify bottlenecks and areas for optimization. Theexplain()
output shows the index used (or lack thereof), the number of documents examined, and other performance metrics. -
Aggregation Pipeline Optimization: When using aggregation pipelines, try to minimize the number of stages and ensure that each stage efficiently processes the data. Consider using
$lookup
for joins instead of multiple stages when possible.
By carefully selecting and using advanced operators, and by optimizing your queries through indexing and efficient data retrieval, you can significantly improve the performance of your MongoDB applications.
The above is the detailed content of How do I use MongoDB's query operators for advanced filtering?. For more information, please follow other related articles on the PHP Chinese website!

Hot AI Tools

Undresser.AI Undress
AI-powered app for creating realistic nude photos

AI Clothes Remover
Online AI tool for removing clothes from photos.

Undress AI Tool
Undress images for free

Clothoff.io
AI clothes remover

AI Hentai Generator
Generate AI Hentai for free.

Hot Article

Hot Tools

Notepad++7.3.1
Easy-to-use and free code editor

SublimeText3 Chinese version
Chinese version, very easy to use

Zend Studio 13.0.1
Powerful PHP integrated development environment

Dreamweaver CS6
Visual web development tools

SublimeText3 Mac version
God-level code editing software (SublimeText3)

Hot Topics


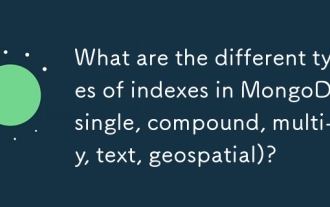
The article discusses various MongoDB index types (single, compound, multi-key, text, geospatial) and their impact on query performance. It also covers considerations for choosing the right index based on data structure and query needs.
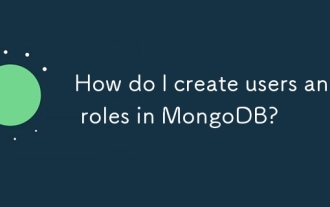
The article discusses creating users and roles in MongoDB, managing permissions, ensuring security, and automating these processes. It emphasizes best practices like least privilege and role-based access control.
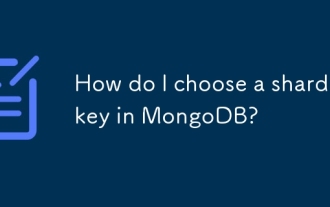
The article discusses selecting a shard key in MongoDB, emphasizing its impact on performance and scalability. Key considerations include high cardinality, query patterns, and avoiding monotonic growth.
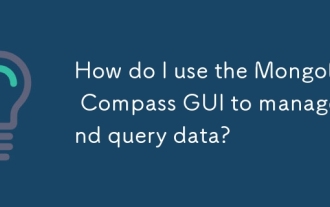
This article explains how to use MongoDB Compass, a GUI for managing and querying MongoDB databases. It covers connecting, navigating databases, querying with a visual builder, data manipulation, and import/export. While efficient for smaller datas
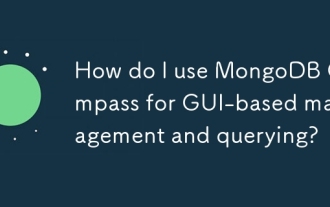
MongoDB Compass is a GUI tool for managing and querying MongoDB databases. It offers features for data exploration, complex query execution, and data visualization.
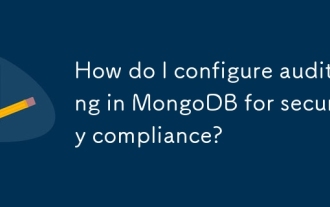
The article discusses configuring MongoDB auditing for security compliance, detailing steps to enable auditing, set up audit filters, and ensure logs meet regulatory standards. Main issue: proper configuration and analysis of audit logs for security
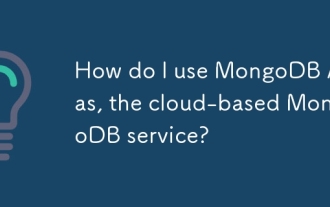
This article guides users through MongoDB Atlas, a cloud-based NoSQL database. It covers setup, cluster management, data handling, scaling, security, and optimization strategies, highlighting key differences from self-hosted MongoDB and emphasizing
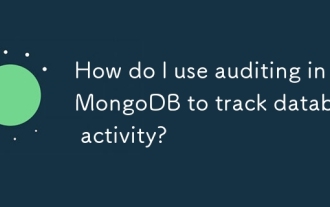
This article details how to implement auditing in MongoDB using change streams, aggregation pipelines, and various storage options (other MongoDB collections, external databases, message queues). It emphasizes performance optimization (filtering, as
