


How do I use the aggregation framework in MongoDB for complex data transformations?
This article explains MongoDB's aggregation framework, a pipeline-based tool for complex data transformations. It details using stages like $group, $sort, $match, and $lookup for tasks such as calculating totals, filtering, joining collections, and
How to Use the MongoDB Aggregation Framework for Complex Data Transformations
The MongoDB aggregation framework is a powerful tool for performing complex data transformations directly within the database. It uses a pipeline-based approach, where data passes through a series of stages, each performing a specific operation. These stages can include filtering, grouping, sorting, projecting, and more. Let's illustrate with an example. Imagine you have a collection called sales
with documents like this:
{ "_id" : ObjectId("5f9f16c75474444444444444"), "item" : "ABC", "price" : 10, "quantity" : 2, "date" : ISODate("2024-01-15T00:00:00Z") } { "_id" : ObjectId("5f9f16c75474444444444445"), "item" : "XYZ", "price" : 20, "quantity" : 1, "date" : ISODate("2024-01-15T00:00:00Z") } { "_id" : ObjectId("5f9f16c75474444444444446"), "item" : "ABC", "price" : 10, "quantity" : 3, "date" : ISODate("2024-01-16T00:00:00Z") }
To calculate the total revenue for each item, you would use the following aggregation pipeline:
db.sales.aggregate([ { $group: { _id: "$item", totalRevenue: { $sum: { $multiply: ["$price", "$quantity"] } } } }, { $sort: { totalRevenue: -1 } } ])
This pipeline first groups the documents by the item
field using $group
. Then, for each group, it calculates the totalRevenue
using $sum
and $multiply
to multiply price and quantity. Finally, it sorts the results in descending order of totalRevenue
using $sort
. This demonstrates how multiple stages can be chained together for complex transformations. Other common stages include $match
(filtering), $project
(selecting and renaming fields), $unwind
(deconstructing arrays), and $lookup
(joining with other collections – discussed later).
What Are Some Common Use Cases for MongoDB's Aggregation Framework Beyond Simple Queries?
Beyond simple queries like finding documents matching specific criteria, the aggregation framework excels in scenarios requiring data manipulation and analysis. Here are some common use cases:
- Real-time analytics: Aggregations can process streaming data to provide immediate insights into trends and patterns. For example, tracking website traffic in real-time or monitoring sensor data.
- Data enrichment: Adding calculated fields or derived data to existing documents. This might involve calculating totals, averages, or ratios based on other fields.
- Reporting and dashboards: Generating summarized data for reports and visualizations. Aggregations can group data, calculate aggregates, and format the results for easy consumption.
- Data cleaning and transformation: Transforming data into a more usable format, such as converting data types or restructuring documents.
- Complex filtering and sorting: Performing intricate filtering and sorting operations that are difficult or impossible to achieve with simple query operators.
- Building complex analytical queries: Performing operations like calculating moving averages, percentiles, or other statistical measures.
How Can I Optimize MongoDB Aggregation Pipelines for Performance with Large Datasets?
Optimizing aggregation pipelines for large datasets is crucial for performance. Here are some key strategies:
- Indexing: Ensure appropriate indexes are created on fields used in
$match
,$sort
,$group
, and$lookup
stages. Indexes significantly speed up data retrieval. - Filtering early: Use
$match
stages early in the pipeline to filter out unwanted documents as soon as possible. This reduces the amount of data processed by subsequent stages. - Limit the number of stages: Excessive stages can slow down processing. Try to consolidate operations where possible.
- Use appropriate aggregation operators: Choose the most efficient operators for the task. For example,
$sum
is generally faster than$reduce
for summing values. - Avoid unnecessary field projections: Only project the necessary fields in
$project
stages to reduce the data volume processed. - Optimize
$lookup
joins: When joining collections, ensure the joined collection has an appropriate index on the join field. Consider using$lookup
withlet
andpipeline
for complex join conditions. - Shard your data: For extremely large datasets, sharding distributes the data across multiple servers, improving scalability and performance.
- Use
explain()
: Use thedb.collection.aggregate(...).explain()
method to analyze the execution plan and identify potential bottlenecks.
Can I Use the MongoDB Aggregation Framework to Perform Joins or Lookups from Other Collections?
Yes, the MongoDB aggregation framework supports joins and lookups from other collections using the $lookup
stage. $lookup
performs a left outer join, bringing in data from another collection based on a specified join condition.
For example, consider two collections: customers
and orders
.
// customers collection { "_id" : 1, "name" : "John Doe" } { "_id" : 2, "name" : "Jane Smith" } // orders collection { "_id" : 101, "customer_id" : 1, "amount" : 100 } { "_id" : 102, "customer_id" : 1, "amount" : 200 } { "_id" : 103, "customer_id" : 2, "amount" : 50 }
To retrieve customer information along with their orders, you'd use the following aggregation pipeline:
db.customers.aggregate([ { $lookup: { from: "orders", localField: "_id", foreignField: "customer_id", as: "orders" } } ])
This pipeline joins customers
and orders
collections based on the _id
field in customers
and customer_id
field in orders
. The result will include the customer's information and an array of their associated orders within the orders
field. Remember to create indexes on the _id
field in customers
and customer_id
field in orders
for optimal performance. More complex join conditions can be achieved using the let
and pipeline
options within the $lookup
stage.
The above is the detailed content of How do I use the aggregation framework in MongoDB for complex data transformations?. For more information, please follow other related articles on the PHP Chinese website!

Hot AI Tools

Undresser.AI Undress
AI-powered app for creating realistic nude photos

AI Clothes Remover
Online AI tool for removing clothes from photos.

Undress AI Tool
Undress images for free

Clothoff.io
AI clothes remover

Video Face Swap
Swap faces in any video effortlessly with our completely free AI face swap tool!

Hot Article

Hot Tools

Notepad++7.3.1
Easy-to-use and free code editor

SublimeText3 Chinese version
Chinese version, very easy to use

Zend Studio 13.0.1
Powerful PHP integrated development environment

Dreamweaver CS6
Visual web development tools

SublimeText3 Mac version
God-level code editing software (SublimeText3)

Hot Topics
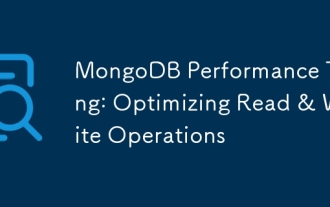
The core strategies of MongoDB performance tuning include: 1) creating and using indexes, 2) optimizing queries, and 3) adjusting hardware configuration. Through these methods, the read and write performance of the database can be significantly improved, response time, and throughput can be improved, thereby optimizing the user experience.
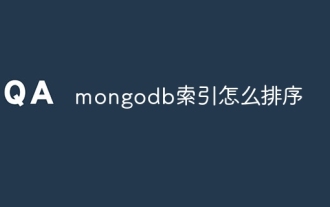
Sorting index is a type of MongoDB index that allows sorting documents in a collection by specific fields. Creating a sort index allows you to quickly sort query results without additional sorting operations. Advantages include quick sorting, override queries, and on-demand sorting. The syntax is db.collection.createIndex({ field: <sort order> }), where <sort order> is 1 (ascending order) or -1 (descending order). You can also create multi-field sorting indexes that sort multiple fields.
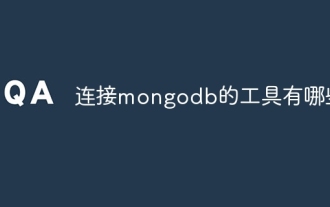
The main tools for connecting to MongoDB are: 1. MongoDB Shell, suitable for quickly viewing data and performing simple operations; 2. Programming language drivers (such as PyMongo, MongoDB Java Driver, MongoDB Node.js Driver), suitable for application development, but you need to master the usage methods; 3. GUI tools (such as Robo 3T, Compass) provide a graphical interface for beginners and quick data viewing. When selecting tools, you need to consider application scenarios and technology stacks, and pay attention to connection string configuration, permission management and performance optimization, such as using connection pools and indexes.
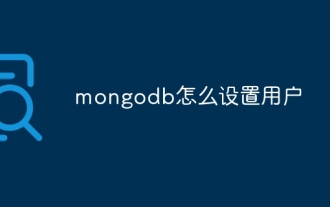
To set up a MongoDB user, follow these steps: 1. Connect to the server and create an administrator user. 2. Create a database to grant users access. 3. Use the createUser command to create a user and specify their role and database access rights. 4. Use the getUsers command to check the created user. 5. Optionally set other permissions or grant users permissions to a specific collection.
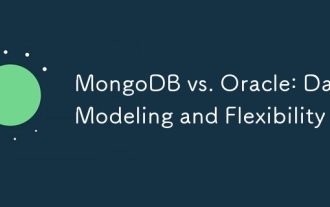
MongoDB is more suitable for processing unstructured data and rapid iteration, while Oracle is more suitable for scenarios that require strict data consistency and complex queries. 1.MongoDB's document model is flexible and suitable for handling complex data structures. 2. Oracle's relationship model is strict to ensure data consistency and complex query performance.
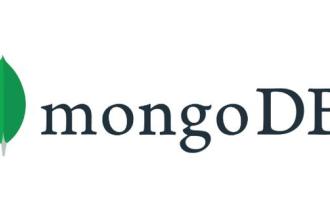
Choosing MongoDB or relational database depends on application requirements. 1. Relational databases (such as MySQL) are suitable for applications that require high data integrity and consistency and fixed data structures, such as banking systems; 2. NoSQL databases such as MongoDB are suitable for processing massive, unstructured or semi-structured data and have low requirements for data consistency, such as social media platforms. The final choice needs to weigh the pros and cons and decide based on the actual situation. There is no perfect database, only the most suitable database.
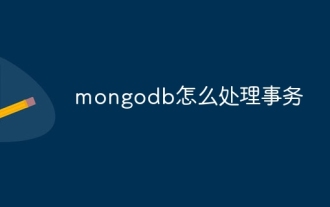
Transaction processing in MongoDB provides solutions such as multi-document transactions, snapshot isolation, and external transaction managers to achieve transaction behavior, ensure multiple operations are executed as one atomic unit, ensuring atomicity and isolation. Suitable for applications that need to ensure data integrity, prevent concurrent operational data corruption, or implement atomic updates in distributed systems. However, its transaction processing capabilities are limited and are only suitable for a single database instance. Multi-document transactions only support read and write operations. Snapshot isolation does not provide atomic guarantees. Integrating external transaction managers may also require additional development work.
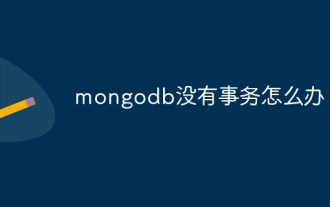
MongoDB lacks transaction mechanisms, which makes it unable to guarantee the atomicity, consistency, isolation and durability of database operations. Alternative solutions include verification and locking mechanisms, distributed transaction coordinators, and transaction engines. When choosing an alternative solution, its complexity, performance, and data consistency requirements should be considered.
