


How do I use RedisBloom for probabilistic data structures (Bloom filters, Cuckoo filters)?
How do I use RedisBloom for probabilistic data structures (Bloom filters, Cuckoo filters)?
RedisBloom is a Redis module that provides support for probabilistic data structures such as Bloom filters and Cuckoo filters. Here’s a step-by-step guide on how to use RedisBloom for these structures:
-
Installation: First, ensure that you have RedisBloom installed. You can install it by compiling from source, using a binary release, or using Docker. For example, to install using Docker:
1
docker run -p 6379:6379 --name redis-redisbloom redislabs/rebloom:latest
Copy after login - Connecting to Redis: Connect to your Redis server that has RedisBloom installed. You can use the Redis CLI or any Redis client that supports modules.
Creating and Managing Bloom Filters:
Creating a Bloom Filter: Use the
BF.RESERVE
command to create a Bloom filter. You need to specify a key, an initial size, and an error rate.1
BF.RESERVE myBloomFilter 0.01 1000
Copy after loginThis creates a Bloom filter named
myBloomFilter
with a 1% error rate and an initial capacity for 1000 items.Adding Items: Use
BF.ADD
orBF.MADD
to add items to your Bloom filter.1
2
BF.ADD myBloomFilter item1
BF.MADD myBloomFilter item1 item2 item3
Copy after loginChecking Membership: Use
BF.EXISTS
orBF.MEXISTS
to check if items are in the Bloom filter.1
2
BF.EXISTS myBloomFilter item1
BF.MEXISTS myBloomFilter item1 item2 item3
Copy after login
Creating and Managing Cuckoo Filters:
Creating a Cuckoo Filter: Use the
CF.RESERVE
command to create a Cuckoo filter. You need to specify a key and an initial size.1
CF.RESERVE myCuckooFilter 1000
Copy after loginThis creates a Cuckoo filter named
myCuckooFilter
with an initial capacity for 1000 items.Adding Items: Use
CF.ADD
orCF.ADDNX
to add items to your Cuckoo filter.1
2
CF.ADD myCuckooFilter item1
CF.ADDNX myCuckooFilter item1
Copy after loginChecking and Deleting Items: Use
CF.EXISTS
to check if an item exists,CF.DEL
to delete an item, andCF.COUNT
to count the number of times an item was added.1
2
3
CF.EXISTS myCuckooFilter item1
CF.DEL myCuckooFilter item1
CF.
COUNT
myCuckooFilter item1
Copy after login
What are the best practices for configuring Bloom filters in RedisBloom?
When configuring Bloom filters in RedisBloom, consider the following best practices:
- Choose the Right Error Rate: The error rate (
error_rate
parameter) affects the space efficiency of the Bloom filter. A lower error rate requires more space but reduces the probability of false positives. For most applications, an error rate between 0.001 and 0.01 is a good balance. - Estimate Capacity: Accurately estimate the number of items you expect to add to the filter (
initial_size
parameter). Underestimating this can lead to reduced performance, while overestimating wastes space. It's better to slightly overestimate than underestimate. - Expansion Strategy: If the initial capacity is exceeded, RedisBloom can automatically expand the Bloom filter. Set the
expansion
parameter to control how much the filter should grow when it reaches capacity. A typical value is 1 (double the size). - Non-Scaling Filters: For use cases where you have a fixed number of items, consider setting
nonscaling
totrue
. This can help optimize memory usage but means the filter cannot be expanded after creation. - Monitoring and Adjusting: Regularly monitor the performance of your Bloom filters, especially the false positive rate. Adjust the parameters if needed to maintain optimal performance.
Example configuration:
1 |
|
How can I optimize the performance of Cuckoo filters in RedisBloom?
To optimize the performance of Cuckoo filters in RedisBloom, follow these strategies:
- Initial Capacity Estimation: Accurately estimate the initial capacity (
size
parameter). Cuckoo filters are more space-efficient than Bloom filters but can become slower if they need to be expanded multiple times. - Bucket Size: The
bucketSize
parameter affects the trade-off between space and performance. A larger bucket size can lead to fewer relocations but uses more memory. A typical value is 2, but you can adjust it based on your workload. - Max Iterations: The
maxIterations
parameter controls the maximum number of relocation attempts before an item is rejected. Increasing this value can improve the filter's ability to accept items but can also increase the time needed for insertion. - Expansion Strategy: Similar to Bloom filters, you can use the
expansion
parameter to control how much the Cuckoo filter grows when it reaches capacity. A typical value is 1 (double the size). - Monitoring and Tuning: Monitor the filter's performance, especially the rate of insertions and deletions. Adjust the parameters based on the actual workload to maintain optimal performance.
Example configuration:
1 |
|
What are the common use cases for probabilistic data structures in RedisBloom?
Probabilistic data structures in RedisBloom, such as Bloom filters and Cuckoo filters, are useful in a variety of scenarios where space and time efficiency are critical. Common use cases include:
- Caching and Duplicate Detection: Use Bloom filters to quickly check if an item is in a cache or to detect duplicates in large datasets. This is particularly useful in web crawlers and data pipelines to avoid processing duplicate items.
- Membership Testing: Cuckoo filters are great for testing whether an item is a member of a set with high accuracy and the ability to delete items. This is useful in applications like user session tracking or inventory management systems.
- Network and Security Applications: Bloom filters can be used in network routers to quickly check if an IP address is blacklisted or to filter out known spam emails without needing to store the full list of addresses or emails.
- Recommendation Systems: Probabilistic data structures can help in recommendation systems by quickly determining whether a user has already been recommended a specific item, reducing the computational load.
- Real-time Analytics: In real-time analytics, Bloom filters can be used to quickly aggregate data and identify trends without maintaining large data sets in memory.
- Fraud Detection: Use Cuckoo filters to quickly check if a transaction or user is flagged as potentially fraudulent, improving the efficiency of fraud detection systems.
By leveraging RedisBloom's probabilistic data structures, applications can achieve significant performance improvements in handling large volumes of data with a small memory footprint.
The above is the detailed content of How do I use RedisBloom for probabilistic data structures (Bloom filters, Cuckoo filters)?. For more information, please follow other related articles on the PHP Chinese website!

Hot AI Tools

Undresser.AI Undress
AI-powered app for creating realistic nude photos

AI Clothes Remover
Online AI tool for removing clothes from photos.

Undress AI Tool
Undress images for free

Clothoff.io
AI clothes remover

Video Face Swap
Swap faces in any video effortlessly with our completely free AI face swap tool!

Hot Article

Hot Tools

Notepad++7.3.1
Easy-to-use and free code editor

SublimeText3 Chinese version
Chinese version, very easy to use

Zend Studio 13.0.1
Powerful PHP integrated development environment

Dreamweaver CS6
Visual web development tools

SublimeText3 Mac version
God-level code editing software (SublimeText3)

Hot Topics
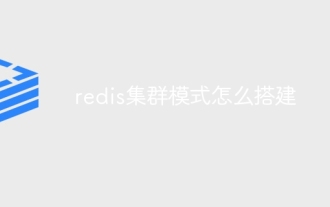
Redis cluster mode deploys Redis instances to multiple servers through sharding, improving scalability and availability. The construction steps are as follows: Create odd Redis instances with different ports; Create 3 sentinel instances, monitor Redis instances and failover; configure sentinel configuration files, add monitoring Redis instance information and failover settings; configure Redis instance configuration files, enable cluster mode and specify the cluster information file path; create nodes.conf file, containing information of each Redis instance; start the cluster, execute the create command to create a cluster and specify the number of replicas; log in to the cluster to execute the CLUSTER INFO command to verify the cluster status; make
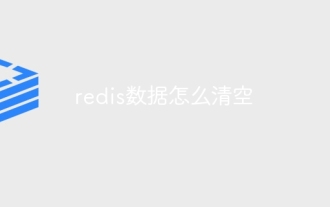
How to clear Redis data: Use the FLUSHALL command to clear all key values. Use the FLUSHDB command to clear the key value of the currently selected database. Use SELECT to switch databases, and then use FLUSHDB to clear multiple databases. Use the DEL command to delete a specific key. Use the redis-cli tool to clear the data.
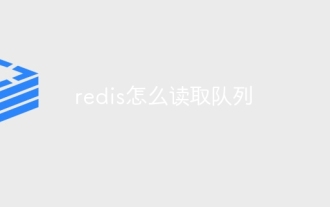
To read a queue from Redis, you need to get the queue name, read the elements using the LPOP command, and process the empty queue. The specific steps are as follows: Get the queue name: name it with the prefix of "queue:" such as "queue:my-queue". Use the LPOP command: Eject the element from the head of the queue and return its value, such as LPOP queue:my-queue. Processing empty queues: If the queue is empty, LPOP returns nil, and you can check whether the queue exists before reading the element.
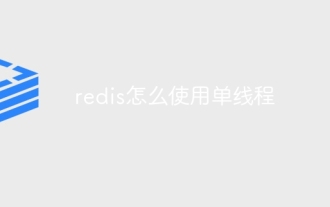
Redis uses a single threaded architecture to provide high performance, simplicity, and consistency. It utilizes I/O multiplexing, event loops, non-blocking I/O, and shared memory to improve concurrency, but with limitations of concurrency limitations, single point of failure, and unsuitable for write-intensive workloads.
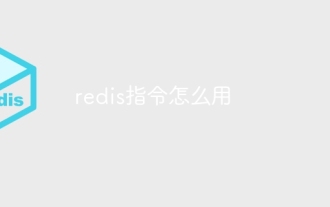
Using the Redis directive requires the following steps: Open the Redis client. Enter the command (verb key value). Provides the required parameters (varies from instruction to instruction). Press Enter to execute the command. Redis returns a response indicating the result of the operation (usually OK or -ERR).
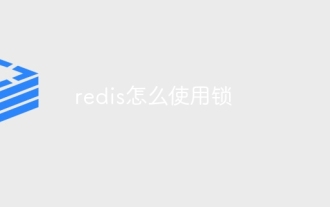
Using Redis to lock operations requires obtaining the lock through the SETNX command, and then using the EXPIRE command to set the expiration time. The specific steps are: (1) Use the SETNX command to try to set a key-value pair; (2) Use the EXPIRE command to set the expiration time for the lock; (3) Use the DEL command to delete the lock when the lock is no longer needed.
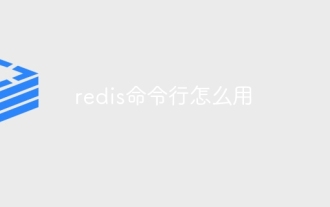
Use the Redis command line tool (redis-cli) to manage and operate Redis through the following steps: Connect to the server, specify the address and port. Send commands to the server using the command name and parameters. Use the HELP command to view help information for a specific command. Use the QUIT command to exit the command line tool.
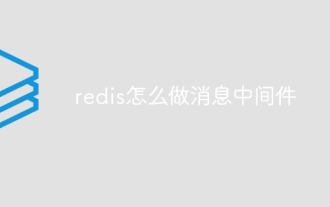
Redis, as a message middleware, supports production-consumption models, can persist messages and ensure reliable delivery. Using Redis as the message middleware enables low latency, reliable and scalable messaging.
