Building an Earnings Report Agent with Swarm Framework
Unlock the Power of Automated Earnings Report Analysis with OpenAI's Swarm Framework
Imagine automating the painstaking process of analyzing earnings reports, extracting key insights, and generating informed recommendations—all effortlessly. This article demonstrates how to build a multi-agent system using OpenAI's Swarm framework to achieve just that. We'll construct three specialized agents: a summarizer, a sentiment analyzer, and a recommendation engine. This scalable, modular solution streamlines financial analysis and extends beyond earnings reports to various applications.
Key Learning Objectives:
- Grasp the fundamentals of OpenAI's Swarm framework for multi-agent systems.
- Develop agents for summarizing, sentiment analysis, and generating recommendations.
- Utilize modular agents for efficient earnings report analysis.
- Securely manage API keys using a .env file.
- Implement a multi-agent system to automate earnings report processing.
- Understand real-world applications of multi-agent systems in finance.
- Set up and execute a multi-agent workflow using OpenAI's Swarm.
This article is part of the Data Science Blogathon.
Table of Contents:
- Key Learning Objectives
- Introducing OpenAI's Swarm
- Multi-Agent System Applications and Advantages
- Step 1: Project Setup
- Step 2: Secure API Key Storage
- Step 3: Agent Implementation
- Step 4: File Loading Helper Function
- Step 5: Integrating Components in
main.py
- Step 6: Sample Earnings Report Creation
- Step 7: Program Execution
- Conclusion
- Frequently Asked Questions
Introducing OpenAI's Swarm:
Swarm, OpenAI's lightweight, experimental framework, excels at multi-agent orchestration. It facilitates the coordination of multiple agents, each specializing in a particular task (e.g., summarization, sentiment analysis, recommendation generation). Our system will comprise three agents:
- Summary Agent: Provides a concise summary of the earnings report.
- Sentiment Agent: Analyzes the sentiment expressed in the report.
- Recommendation Agent: Offers actionable recommendations based on sentiment analysis.
Multi-Agent System Applications and Advantages:
The multi-agent system presented here is adaptable to various use cases:
- Portfolio Management: Automate the monitoring of multiple company reports and suggest portfolio adjustments based on sentiment trends.
- Financial News Summarization: Integrate real-time news feeds to proactively identify potential market shifts.
- Sentiment Tracking: Predict stock or crypto movements based on positive/negative market news sentiment.
The modularity of agents enables component reuse across projects, enhancing flexibility and scalability.
Step 1: Project Setup:
Begin by creating the project structure and installing necessary dependencies:
1 2 3 4 5 |
|
Step 2: Secure API Key Storage:
Securely store your OpenAI API key in a .env
file:
1 |
|
This prevents API key exposure within your code.
Step 3: Agent Implementation:
Let's create the three agents:
Summary Agent (agents/summary_agent.py
):
1 2 3 4 5 6 7 8 9 10 11 |
|
Sentiment Agent (agents/sentiment_agent.py
):
1 2 3 4 5 6 7 8 9 10 11 12 |
|
Recommendation Agent (agents/recommendation_agent.py
):
1 2 3 4 5 6 7 8 9 10 11 12 |
|
Step 4: File Loading Helper Function:
Create a helper function (utils/helpers.py
) for efficient file loading:
1 2 3 |
|
Step 5: Integrating Components in main.py
:
Orchestrate the agents in your main script:
1 2 3 4 5 6 7 8 9 10 |
|
Step 6: Sample Earnings Report Creation:
Create sample_earnings.txt
with sample data:
1 2 |
|
Step 7: Program Execution:
Run the program:
1 |
|
(Expected output similar to the image provided in the original article.)
Conclusion:
This tutorial demonstrates a multi-agent system using OpenAI's Swarm for automated earnings report analysis. Its modular design and adaptability make it a powerful tool for financial analysis and beyond. Further enhancements could include integrating real-time financial APIs for dynamic analysis.
Frequently Asked Questions: (Similar to the original FAQ section, but potentially rephrased for better clarity and flow)
The media shown in this article is not owned by [Platform Name] and is used at the Author’s discretion.
The above is the detailed content of Building an Earnings Report Agent with Swarm Framework. For more information, please follow other related articles on the PHP Chinese website!

Hot AI Tools

Undresser.AI Undress
AI-powered app for creating realistic nude photos

AI Clothes Remover
Online AI tool for removing clothes from photos.

Undress AI Tool
Undress images for free

Clothoff.io
AI clothes remover

Video Face Swap
Swap faces in any video effortlessly with our completely free AI face swap tool!

Hot Article

Hot Tools

Notepad++7.3.1
Easy-to-use and free code editor

SublimeText3 Chinese version
Chinese version, very easy to use

Zend Studio 13.0.1
Powerful PHP integrated development environment

Dreamweaver CS6
Visual web development tools

SublimeText3 Mac version
God-level code editing software (SublimeText3)

Hot Topics










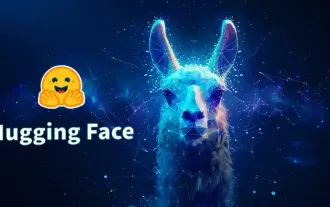
Meta's Llama 3.2: A Leap Forward in Multimodal and Mobile AI Meta recently unveiled Llama 3.2, a significant advancement in AI featuring powerful vision capabilities and lightweight text models optimized for mobile devices. Building on the success o
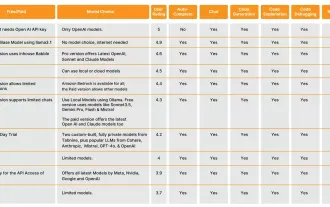
Hey there, Coding ninja! What coding-related tasks do you have planned for the day? Before you dive further into this blog, I want you to think about all your coding-related woes—better list those down. Done? – Let’
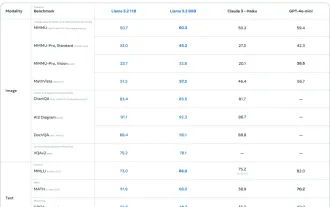
This week's AI landscape: A whirlwind of advancements, ethical considerations, and regulatory debates. Major players like OpenAI, Google, Meta, and Microsoft have unleashed a torrent of updates, from groundbreaking new models to crucial shifts in le
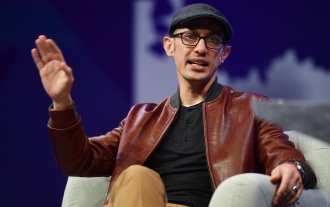
Shopify CEO Tobi Lütke's recent memo boldly declares AI proficiency a fundamental expectation for every employee, marking a significant cultural shift within the company. This isn't a fleeting trend; it's a new operational paradigm integrated into p
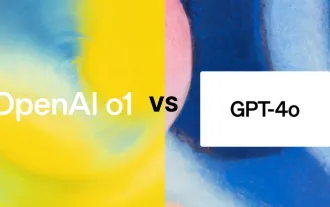
Introduction OpenAI has released its new model based on the much-anticipated “strawberry” architecture. This innovative model, known as o1, enhances reasoning capabilities, allowing it to think through problems mor
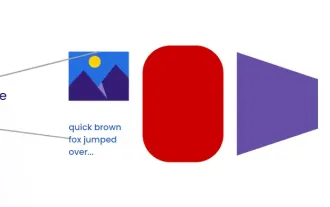
Introduction Imagine walking through an art gallery, surrounded by vivid paintings and sculptures. Now, what if you could ask each piece a question and get a meaningful answer? You might ask, “What story are you telling?
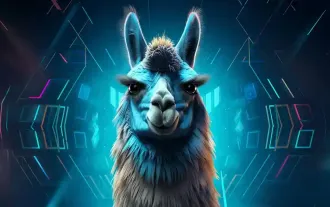
Meta's Llama 3.2: A Multimodal AI Powerhouse Meta's latest multimodal model, Llama 3.2, represents a significant advancement in AI, boasting enhanced language comprehension, improved accuracy, and superior text generation capabilities. Its ability t
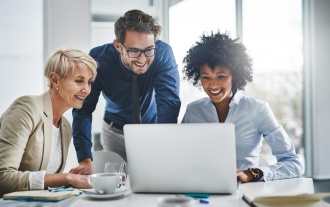
For those of you who might be new to my column, I broadly explore the latest advances in AI across the board, including topics such as embodied AI, AI reasoning, high-tech breakthroughs in AI, prompt engineering, training of AI, fielding of AI, AI re
