What are Distilled Models?
DeepSeek's distilled models, also seen on Ollama and Groq Cloud, are smaller, more efficient versions of original LLMs, designed to match larger models' performance while using fewer resources. This "distillation" process, a form of model compression, was introduced by Geoffrey Hinton in 2015.
Table of Contents:
- Benefits of Distilled Models
- Origin of Distilled Models
- Implementing LLM Distillation
- Understanding Model Distillation
- Challenges and Limitations
- Future of Model Distillation
- Real-World Applications
- Conclusion
Benefits of Distilled Models:
- Lower memory usage and computational needs
- Reduced energy consumption during training and inference
- Faster processing speeds
Related: Building a RAG System for AI Reasoning with DeepSeek R1 Distilled Model
Origin of Distilled Models:
Hinton's 2015 paper, "Distilling the Knowledge in a Neural Network," explored compressing large neural networks into smaller, knowledge-preserving versions. A larger "teacher" model trains a smaller "student" model, aiming for the student to replicate the teacher's key learned weights.
The student learns by minimizing errors against two targets: the ground truth (hard target) and the teacher's predictions (soft target).
Dual Loss Components:
- Hard Loss: Error against true labels.
- Soft Loss: Error against teacher's predictions. This provides nuanced information about class probabilities.
The total loss is a weighted sum of these losses, controlled by parameter λ (lambda). The softmax function, modified with a temperature parameter (T), softens the probability distribution, improving learning. The soft loss is multiplied by T² to compensate for this.
DistilBERT and DistillGPT2:
DistilBERT uses Hinton's method with a cosine embedding loss. It's significantly smaller than BERT-base but with a slight accuracy reduction. DistillGPT2, while faster than GPT-2, shows higher perplexity (lower performance) on large text datasets.
Implementing LLM Distillation:
This involves data preparation, teacher model selection, and a distillation process using frameworks like Hugging Face Transformers, TensorFlow Model Optimization, PyTorch Distiller, or DeepSpeed. Evaluation metrics include accuracy, inference speed, model size, and resource utilization.
Understanding Model Distillation:
The student model can be a simplified teacher model or have a different architecture. The distillation process trains the student to mimic the teacher's behavior by minimizing the difference between their predictions.
Challenges and Limitations:
- Potential accuracy loss compared to the original model.
- Complexity in configuring the distillation process and hyperparameters.
- Variable effectiveness depending on the domain or task.
Future Directions in Model Distillation:
- Improved distillation techniques to reduce performance gaps.
- Automated distillation processes for easier implementation.
- Wider applications across different machine learning areas.
Real-World Applications:
- Mobile and edge computing.
- Energy-efficient cloud services.
- Faster prototyping for startups and researchers.
Conclusion:
Distilled models offer a valuable balance between performance and efficiency. While they may not surpass the original model, their reduced resource requirements make them highly beneficial in various applications. The choice between a distilled model and the original depends on the acceptable performance trade-off and available computational resources.
The above is the detailed content of What are Distilled Models?. For more information, please follow other related articles on the PHP Chinese website!

Hot AI Tools

Undresser.AI Undress
AI-powered app for creating realistic nude photos

AI Clothes Remover
Online AI tool for removing clothes from photos.

Undress AI Tool
Undress images for free

Clothoff.io
AI clothes remover

Video Face Swap
Swap faces in any video effortlessly with our completely free AI face swap tool!

Hot Article

Hot Tools

Notepad++7.3.1
Easy-to-use and free code editor

SublimeText3 Chinese version
Chinese version, very easy to use

Zend Studio 13.0.1
Powerful PHP integrated development environment

Dreamweaver CS6
Visual web development tools

SublimeText3 Mac version
God-level code editing software (SublimeText3)

Hot Topics
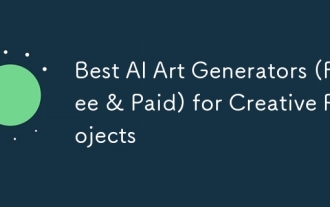
The article reviews top AI art generators, discussing their features, suitability for creative projects, and value. It highlights Midjourney as the best value for professionals and recommends DALL-E 2 for high-quality, customizable art.
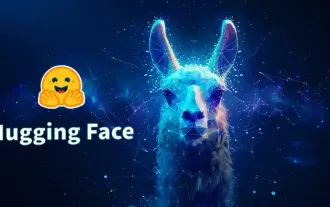
Meta's Llama 3.2: A Leap Forward in Multimodal and Mobile AI Meta recently unveiled Llama 3.2, a significant advancement in AI featuring powerful vision capabilities and lightweight text models optimized for mobile devices. Building on the success o
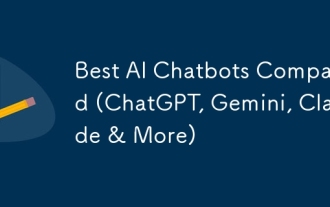
The article compares top AI chatbots like ChatGPT, Gemini, and Claude, focusing on their unique features, customization options, and performance in natural language processing and reliability.
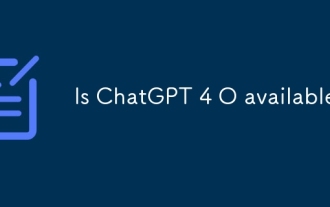
ChatGPT 4 is currently available and widely used, demonstrating significant improvements in understanding context and generating coherent responses compared to its predecessors like ChatGPT 3.5. Future developments may include more personalized interactions and real-time data processing capabilities, further enhancing its potential for various applications.
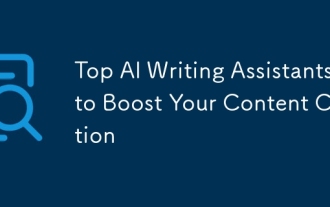
The article discusses top AI writing assistants like Grammarly, Jasper, Copy.ai, Writesonic, and Rytr, focusing on their unique features for content creation. It argues that Jasper excels in SEO optimization, while AI tools help maintain tone consist
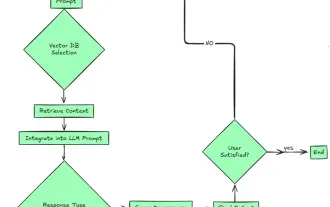
2024 witnessed a shift from simply using LLMs for content generation to understanding their inner workings. This exploration led to the discovery of AI Agents – autonomous systems handling tasks and decisions with minimal human intervention. Buildin
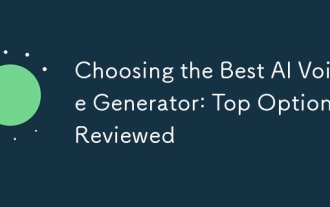
The article reviews top AI voice generators like Google Cloud, Amazon Polly, Microsoft Azure, IBM Watson, and Descript, focusing on their features, voice quality, and suitability for different needs.
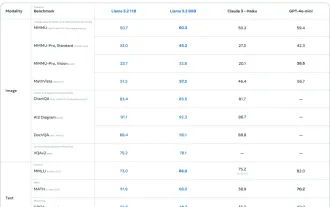
This week's AI landscape: A whirlwind of advancements, ethical considerations, and regulatory debates. Major players like OpenAI, Google, Meta, and Microsoft have unleashed a torrent of updates, from groundbreaking new models to crucial shifts in le
