How to Access Data Science Agent in Google Colab? - Analytics Vidhya
Google Colab's Gemini-powered Data Science Agent: Your AI-Driven Data Analysis Assistant
Tired of tedious data analysis? Google Colab's new Data Science Agent, powered by Gemini AI, automates data preprocessing, exploratory data analysis (EDA), model building, and even code generation, letting you focus on insights. This guide explores its capabilities, from automating data manipulation and visualization to building multi-agent systems. Perfect for both beginners and experienced data scientists, it streamlines your workflow and enhances teamwork in cloud notebooks.
Table of Contents
- What is the Data Science Agent?
- Using the Data Science Agent in Google Colab
- Data Science Agent in Action:
- Task 1: Automated Data Analysis & Visualization
- Task 2: Automated Model Evaluation & Optimization
- Task 3: Building a Multi-agent System
- Key Applications
- Future Enhancements
- Frequently Asked Questions
What is a Data Science Agent?
The Data Science Agent is an AI assistant that simplifies data analysis by automating tasks like data cleaning, EDA, feature engineering, and model development. Within Google Colab, it acts as an intelligent helper, automating library imports, data loading, visualization, code generation, and execution. Instead of manual setup, describe your analysis goals in plain language, and the agent generates and executes a Colab notebook, even handling errors. It also provides context-aware suggestions and assists with debugging and code optimization.
Benchmark: The Google Data Science Agent achieved a notable 4th place ranking on the DABStep benchmark for multi-step reasoning on HuggingFace, outperforming several other leading AI agents.
How to Use the Data Science Agent in Google Colab
- New Notebook: Create a new Google Colab notebook.
- Data Upload: Import your dataset (CSV or XLS) using the "Analyze files with Gemini" option in the bottom right corner.
- Define Objectives: In the Gemini side panel, use natural language prompts (e.g., "Visualize trends," "Build a prediction model," "Handle missing values") to specify your analysis goals.
- Automated Execution: The agent generates and executes the necessary code, providing a functional notebook for further refinement.
Gemini Data Science Agent in Action
Let's examine three key tasks: data analysis and visualization, model building, and creating a multi-agent system.
Task 1: Automated Data Analysis – Manipulation & Visualization
This task automates data cleaning, transformation, summarization, and visualization.
Prompt: "Perform data analysis including manipulation and visualization for this dataset."
Response (Initial & Post-Execution):
Analysis: The agent efficiently handled data loading, cleaning (addressing missing values), scaling, and visualization, providing insightful charts and summaries.
Task 2: Automated Model Evaluation and Optimization
This task automates model evaluation and optimization processes.
Prompt: "Use two ML algorithms and evaluate their performance using different metrics."
Response (Initial & Post-Execution):
Analysis: The agent automated data splitting, model training (Logistic Regression and Random Forest), performance evaluation, and hyperparameter tuning, providing a comparison of model performance.
Task 3: Building a Multi-agent System
This task explores building a multi-agent system (using AutoGen or CrewAI).
Prompt: "Build a multi-agent system (using AutoGen or CrewAI) to provide real-time updates on major sports events."
Response:
Analysis: The agent's limitations with real-time data and API interaction were evident here. It provided code snippets but couldn't fully execute the task due to its focus on static datasets.
Key Applications
- Automated data processing (CSV/XLS)
- Sentiment analysis on text data (CSV)
- Deep learning model development (TensorFlow/PyTorch)
- Automated error handling
- Structured workflow for ML projects
Future Implications
Future improvements should include support for unstructured data (TXT, PDF, JSON, images), improved document understanding, and real-time data integration via APIs.
Conclusion
The Data Science Agent is a powerful tool for streamlining data analysis workflows, particularly with structured data. Future enhancements to handle unstructured data and real-time data streams will significantly expand its capabilities.
Frequently Asked Questions
- Q1. What is the Google Colab Data Science Agent? A. An AI assistant automating data analysis tasks in Google Colab.
- Q2. What data formats are supported? A. Primarily CSV and XLS; support for other formats is limited.
- Q3. Can it build deep learning models? A. Yes, it integrates with TensorFlow and PyTorch.
- Q4. Does it automatically fix code errors? A. Yes, it handles some errors during execution.
- Q5. What future improvements are needed? A. Support for unstructured data, document processing, image analysis, and real-time data integration.
The above is the detailed content of How to Access Data Science Agent in Google Colab? - Analytics Vidhya. For more information, please follow other related articles on the PHP Chinese website!

Hot AI Tools

Undresser.AI Undress
AI-powered app for creating realistic nude photos

AI Clothes Remover
Online AI tool for removing clothes from photos.

Undress AI Tool
Undress images for free

Clothoff.io
AI clothes remover

Video Face Swap
Swap faces in any video effortlessly with our completely free AI face swap tool!

Hot Article

Hot Tools

Notepad++7.3.1
Easy-to-use and free code editor

SublimeText3 Chinese version
Chinese version, very easy to use

Zend Studio 13.0.1
Powerful PHP integrated development environment

Dreamweaver CS6
Visual web development tools

SublimeText3 Mac version
God-level code editing software (SublimeText3)

Hot Topics










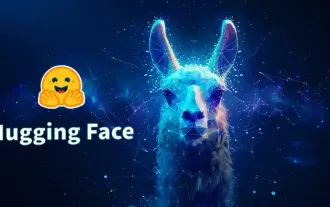
Meta's Llama 3.2: A Leap Forward in Multimodal and Mobile AI Meta recently unveiled Llama 3.2, a significant advancement in AI featuring powerful vision capabilities and lightweight text models optimized for mobile devices. Building on the success o
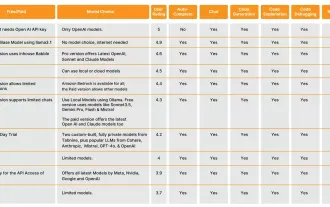
Hey there, Coding ninja! What coding-related tasks do you have planned for the day? Before you dive further into this blog, I want you to think about all your coding-related woes—better list those down. Done? – Let’
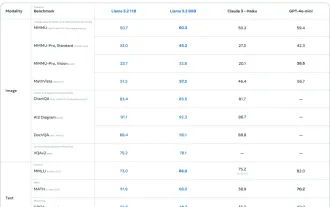
This week's AI landscape: A whirlwind of advancements, ethical considerations, and regulatory debates. Major players like OpenAI, Google, Meta, and Microsoft have unleashed a torrent of updates, from groundbreaking new models to crucial shifts in le
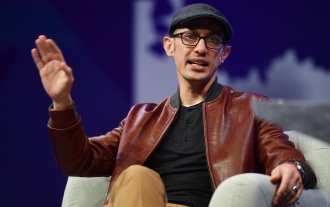
Shopify CEO Tobi Lütke's recent memo boldly declares AI proficiency a fundamental expectation for every employee, marking a significant cultural shift within the company. This isn't a fleeting trend; it's a new operational paradigm integrated into p
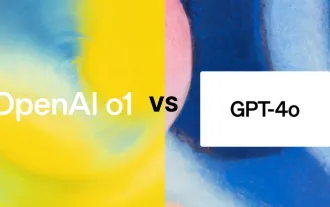
Introduction OpenAI has released its new model based on the much-anticipated “strawberry” architecture. This innovative model, known as o1, enhances reasoning capabilities, allowing it to think through problems mor
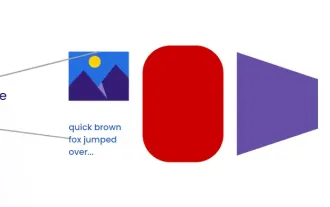
Introduction Imagine walking through an art gallery, surrounded by vivid paintings and sculptures. Now, what if you could ask each piece a question and get a meaningful answer? You might ask, “What story are you telling?
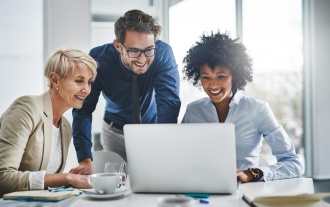
For those of you who might be new to my column, I broadly explore the latest advances in AI across the board, including topics such as embodied AI, AI reasoning, high-tech breakthroughs in AI, prompt engineering, training of AI, fielding of AI, AI re
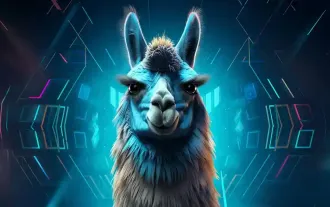
Meta's Llama 3.2: A Multimodal AI Powerhouse Meta's latest multimodal model, Llama 3.2, represents a significant advancement in AI, boasting enhanced language comprehension, improved accuracy, and superior text generation capabilities. Its ability t
