


Explain different data serialization formats (e.g., JSON, Pickle, Protocol Buffers). When would you use each?
Explain different data serialization formats (e.g., JSON, Pickle, Protocol Buffers). When would you use each?
JSON (JavaScript Object Notation):
JSON is a lightweight, text-based data interchange format that is easy for humans to read and write and easy for machines to parse and generate. It is language-independent and widely used in web applications for data exchange between a server and a client.
- When to use JSON: JSON is ideal for web APIs due to its simplicity and wide support in various programming languages. It is also commonly used in configuration files, web services, and NoSQL databases. JSON's human-readable format makes it suitable for scenarios where data may be manually inspected or edited.
Pickle:
Pickle is a Python-specific binary serialization format that can serialize Python objects, including custom classes and complex data structures. It is designed for use within the Python ecosystem.
- When to use Pickle: Pickle is best used for serializing Python objects when data needs to be stored or transferred between Python applications. It is efficient for serializing complex Python data structures. However, because Pickle is specific to Python, it should not be used for cross-language data exchange or when security is a concern.
Protocol Buffers:
Protocol Buffers (protobuf) is a binary serialization format developed by Google, designed to be fast, small, and platform-independent. It requires a schema definition and generates code for the serialization and deserialization of structured data.
- When to use Protocol Buffers: Protocol Buffers are excellent for high-performance scenarios where efficiency and speed are critical, such as in microservices and large-scale systems. They are also suitable for applications that require backward and forward compatibility. Protobuf's use of a schema helps ensure data integrity and can reduce the size of the serialized data.
What are the key differences between JSON, Pickle, and Protocol Buffers in terms of performance and compatibility?
Performance:
- JSON: JSON is relatively slow in terms of serialization and deserialization because it is a text-based format. It is less compact compared to binary formats like Pickle and Protocol Buffers.
- Pickle: Pickle is generally faster than JSON due to its binary nature, optimized for Python. However, it may not be as fast as Protocol Buffers in some scenarios.
- Protocol Buffers: Protocol Buffers offer the best performance in terms of speed and size, as they are designed to be highly efficient and optimized for both serialization and deserialization processes.
Compatibility:
- JSON: JSON is widely compatible with virtually all programming languages and platforms, making it an excellent choice for cross-platform communication.
- Pickle: Pickle is specific to Python and is not compatible with other programming languages. It is also version-specific, meaning data serialized with one version of Python may not be deserializable with another version.
- Protocol Buffers: Protocol Buffers are platform-independent and have excellent backward and forward compatibility, allowing you to add new fields to your data structure without breaking existing applications.
Which data serialization format is best suited for web APIs and why?
JSON is the best-suited format for web APIs due to several reasons:
- Universal Compatibility: JSON is supported by all major programming languages and platforms, making it ideal for web applications where clients and servers may use different technologies.
- Human-Readable: JSON's text-based format is easy to read and debug, which is beneficial for API developers and testers.
- Built-in Browser Support: Modern web browsers natively support JSON, simplifying the integration of web APIs with client-side scripts.
- Lightweight: Although not as compact as binary formats, JSON is still relatively lightweight and adequate for most web API use cases.
- RESTful Services: JSON is the de facto standard for RESTful services, providing a consistent and expected data format for API consumers.
How does the choice of serialization format impact data security and integrity?
Security:
- JSON: JSON is generally secure as it is text-based and easier to inspect for malicious content. However, care must be taken when deserializing JSON data to prevent injection attacks.
- Pickle: Pickle can pose significant security risks because it can execute arbitrary code during deserialization. It should never be used with untrusted data, as it can lead to code injection vulnerabilities.
- Protocol Buffers: Protocol Buffers are considered secure because they rely on a predefined schema, which helps prevent arbitrary code execution. However, the security depends on the proper implementation and use of the schema.
Integrity:
- JSON: JSON's human-readable nature makes it easier to verify data integrity manually. However, it lacks built-in mechanisms for data validation, which could affect data integrity if not handled properly.
- Pickle: Pickle preserves the integrity of Python objects and can include custom validation logic. However, its Python-specific nature limits its use for ensuring cross-platform data integrity.
- Protocol Buffers: Protocol Buffers provide excellent data integrity through the use of schemas. The schema definition helps ensure that the data adheres to a specific structure, reducing the likelihood of data corruption or invalid data being deserialized. Additionally, Protocol Buffers support optional fields, which allow for backward and forward compatibility, further enhancing data integrity.
The above is the detailed content of Explain different data serialization formats (e.g., JSON, Pickle, Protocol Buffers). When would you use each?. For more information, please follow other related articles on the PHP Chinese website!

Hot AI Tools

Undresser.AI Undress
AI-powered app for creating realistic nude photos

AI Clothes Remover
Online AI tool for removing clothes from photos.

Undress AI Tool
Undress images for free

Clothoff.io
AI clothes remover

AI Hentai Generator
Generate AI Hentai for free.

Hot Article

Hot Tools

Notepad++7.3.1
Easy-to-use and free code editor

SublimeText3 Chinese version
Chinese version, very easy to use

Zend Studio 13.0.1
Powerful PHP integrated development environment

Dreamweaver CS6
Visual web development tools

SublimeText3 Mac version
God-level code editing software (SublimeText3)

Hot Topics


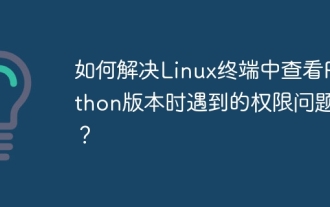
Solution to permission issues when viewing Python version in Linux terminal When you try to view Python version in Linux terminal, enter python...
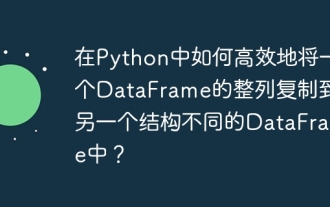
When using Python's pandas library, how to copy whole columns between two DataFrames with different structures is a common problem. Suppose we have two Dats...
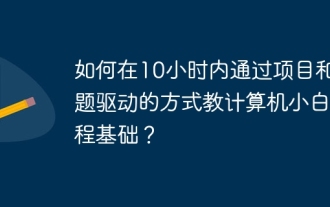
How to teach computer novice programming basics within 10 hours? If you only have 10 hours to teach computer novice some programming knowledge, what would you choose to teach...
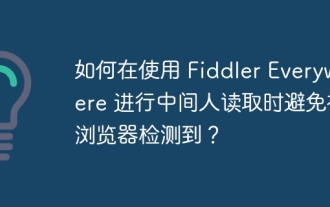
How to avoid being detected when using FiddlerEverywhere for man-in-the-middle readings When you use FiddlerEverywhere...
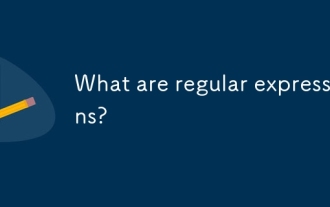
Regular expressions are powerful tools for pattern matching and text manipulation in programming, enhancing efficiency in text processing across various applications.
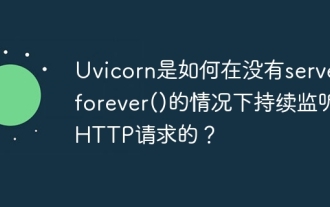
How does Uvicorn continuously listen for HTTP requests? Uvicorn is a lightweight web server based on ASGI. One of its core functions is to listen for HTTP requests and proceed...
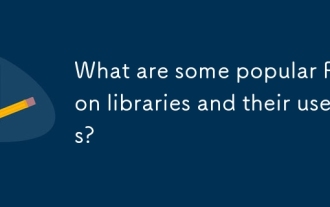
The article discusses popular Python libraries like NumPy, Pandas, Matplotlib, Scikit-learn, TensorFlow, Django, Flask, and Requests, detailing their uses in scientific computing, data analysis, visualization, machine learning, web development, and H
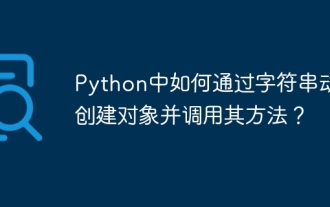
In Python, how to dynamically create an object through a string and call its methods? This is a common programming requirement, especially if it needs to be configured or run...
