Redis as Cache vs Datastore: Trade-offs.
Article discusses trade-offs of using Redis as a cache vs. datastore, focusing on performance, data persistence, and scalability implications.
Redis as Cache vs Datastore: Trade-offs
When considering whether to use Redis as a cache or a datastore, several trade-offs need to be evaluated. Redis is an in-memory data structure store that can function effectively as both a cache and a persistent datastore, but each use case has different implications.
Using Redis as a cache: Redis is primarily used as a cache to store frequently accessed data temporarily, thereby reducing the load on the primary database and improving application response times. In this role, Redis typically holds a subset of the data that is most often read or computed, and it does not need to store all the data an application might require.
Using Redis as a datastore: In contrast, when Redis is used as a datastore, it serves as the primary data storage system. In this scenario, Redis stores all necessary data, and data persistence becomes a crucial factor. This use case leverages Redis's ability to support various data structures like strings, lists, sets, and hashes, making it versatile for different data storage needs.
Trade-offs:
- Data Persistence: When used as a cache, Redis does not prioritize data persistence, as cached data can be recalculated or fetched from the primary database. As a datastore, Redis must ensure data persistence, often through mechanisms like RDB snapshots and AOF (Append Only File) logs.
- Performance: Redis excels at providing low-latency data access when used as a cache. However, when used as a datastore, performance may be slightly impacted due to the overhead of ensuring data persistence.
- Scalability: Using Redis as a cache typically involves simpler scaling strategies since it's expected that some data can be lost and recalculated. When used as a datastore, scaling becomes more complex due to the need to maintain data integrity and consistency across nodes.
- Complexity and Cost: Managing Redis as a cache generally requires less overhead and can be less costly than maintaining it as a full-fledged datastore, where additional resources for persistence and backup are necessary.
By understanding these trade-offs, developers can better decide how to leverage Redis to meet their specific application needs, balancing performance, data integrity, and operational complexity.
What performance benefits can I expect from using Redis as a cache rather than a datastore?
Using Redis as a cache provides several performance benefits over using it as a datastore:
- Reduced Latency: Redis operates in-memory, meaning data retrieval is extremely fast. When used as a cache, Redis can serve frequently accessed data much quicker than a traditional disk-based database, significantly reducing application latency.
- Load Reduction on Primary Database: By caching frequently accessed data in Redis, the primary database experiences reduced load, as fewer queries are directed at it. This not only improves response times for the database but also extends its lifespan by reducing wear and tear.
- High Throughput: Redis, as a cache, can handle a high volume of read requests efficiently. Caching commonly accessed data reduces the number of read operations on the primary database, allowing for higher overall throughput.
- Efficient Data Retrieval: Caching mechanisms like TTL (Time To Live) enable automatic expiration of data, ensuring that the cache contains fresh data. This avoids unnecessary data staleness and maintains the efficiency of data retrieval.
- Simplified Scaling: Scaling Redis as a cache is typically more straightforward than scaling it as a datastore. As a cache, Redis can handle data loss to some extent, making it easier to scale horizontally using clustering techniques.
In summary, using Redis as a cache optimizes performance by leveraging its in-memory capabilities to accelerate data access and reduce the load on the primary database, which results in a more responsive application.
How does data persistence differ when using Redis as a cache versus a datastore?
Data persistence in Redis differs significantly between its use as a cache and as a datastore:
Redis as a Cache:
- Volatility: When used as a cache, Redis is typically configured to be volatile, meaning data can be lost upon server restart or failures. This is acceptable because cached data can be recalculated or fetched from the primary database.
- No Persistence Mechanisms: Although Redis supports persistence mechanisms like RDB snapshots and AOF logs, they are often disabled or minimized when Redis is used purely as a cache to reduce overhead.
- Data Expiration: Cached data often has a TTL set, allowing for automatic data expiration, further emphasizing the transient nature of cached data.
Redis as a Datastore:
- Persistence: As a datastore, Redis needs to ensure data durability and persistence. This is achieved through RDB snapshots, which periodically save the dataset to disk, and AOF logs, which record every write operation.
- Data Integrity: Ensuring data integrity becomes critical, and Redis may employ both RDB and AOF concurrently to balance between performance and data safety. RDB provides a point-in-time backup, while AOF maintains a continuous log.
-
Configuration: Redis configurations such as
appendonly yes
andsave
commands in the configuration file are actively used to manage how and when data is persisted.
In essence, while Redis as a cache can afford to be non-persistent and volatile, Redis as a datastore must prioritize data persistence and integrity, employing mechanisms like RDB and AOF to achieve this.
What are the scalability implications of choosing Redis as a cache over a datastore?
The scalability implications of choosing Redis as a cache versus a datastore are significant and should be considered carefully:
Scalability of Redis as a Cache:
- Simpler Horizontal Scaling: Scaling Redis as a cache is often easier because it can tolerate data loss to some extent. Redis Cluster or Redis Sentinel can be used to distribute the load across multiple nodes, focusing on high availability and redundancy of cached data.
- Load Balancing: Since Redis as a cache can handle read-heavy workloads, load balancers can efficiently distribute read requests across multiple Redis instances, improving scalability without significantly increasing complexity.
- Lower Overhead: Managing Redis as a cache typically requires less overhead, as persistence and data integrity concerns are less critical. This makes it easier to add or remove nodes based on traffic demands.
Scalability of Redis as a Datastore:
- Complex Data Distribution: When Redis is used as a datastore, scaling becomes more complex due to the need to maintain data integrity and consistency across nodes. Redis Cluster can be used, but ensuring all data is replicated and consistently available increases the complexity of deployment.
- Data Sharding: To scale effectively as a datastore, data sharding (partitioning) becomes necessary. This involves careful planning of how data is distributed across nodes, ensuring even load distribution and minimizing cross-node operations.
- Persistence Overhead: The need for data persistence adds additional overhead when scaling Redis as a datastore. Ensuring that RDB snapshots and AOF logs are handled correctly across multiple nodes adds to the management complexity.
- Higher Cost: Scalability efforts for Redis as a datastore may incur higher costs due to the need for more robust hardware to handle both the in-memory and persistence requirements.
In conclusion, while both configurations can be scaled, using Redis as a cache generally offers simpler and more cost-effective scalability compared to using it as a datastore, where maintaining data persistence and integrity complicates the scaling process.
The above is the detailed content of Redis as Cache vs Datastore: Trade-offs.. For more information, please follow other related articles on the PHP Chinese website!

Hot AI Tools

Undresser.AI Undress
AI-powered app for creating realistic nude photos

AI Clothes Remover
Online AI tool for removing clothes from photos.

Undress AI Tool
Undress images for free

Clothoff.io
AI clothes remover

Video Face Swap
Swap faces in any video effortlessly with our completely free AI face swap tool!

Hot Article

Hot Tools

Notepad++7.3.1
Easy-to-use and free code editor

SublimeText3 Chinese version
Chinese version, very easy to use

Zend Studio 13.0.1
Powerful PHP integrated development environment

Dreamweaver CS6
Visual web development tools

SublimeText3 Mac version
God-level code editing software (SublimeText3)

Hot Topics










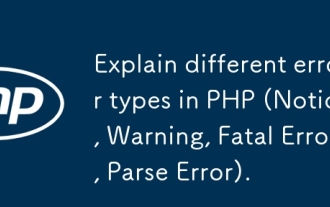
There are four main error types in PHP: 1.Notice: the slightest, will not interrupt the program, such as accessing undefined variables; 2. Warning: serious than Notice, will not terminate the program, such as containing no files; 3. FatalError: the most serious, will terminate the program, such as calling no function; 4. ParseError: syntax error, will prevent the program from being executed, such as forgetting to add the end tag.
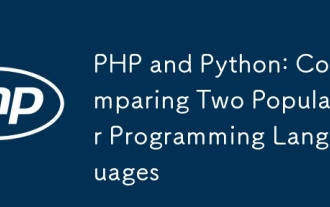
PHP and Python each have their own advantages, and choose according to project requirements. 1.PHP is suitable for web development, especially for rapid development and maintenance of websites. 2. Python is suitable for data science, machine learning and artificial intelligence, with concise syntax and suitable for beginners.
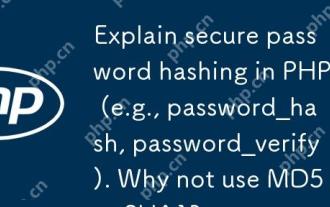
In PHP, password_hash and password_verify functions should be used to implement secure password hashing, and MD5 or SHA1 should not be used. 1) password_hash generates a hash containing salt values to enhance security. 2) Password_verify verify password and ensure security by comparing hash values. 3) MD5 and SHA1 are vulnerable and lack salt values, and are not suitable for modern password security.
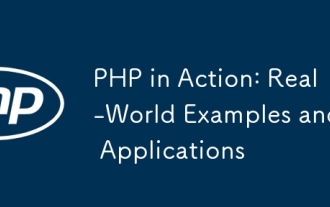
PHP is widely used in e-commerce, content management systems and API development. 1) E-commerce: used for shopping cart function and payment processing. 2) Content management system: used for dynamic content generation and user management. 3) API development: used for RESTful API development and API security. Through performance optimization and best practices, the efficiency and maintainability of PHP applications are improved.
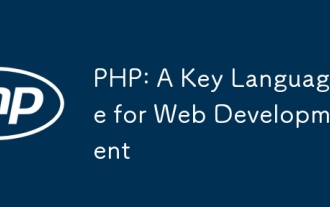
PHP is a scripting language widely used on the server side, especially suitable for web development. 1.PHP can embed HTML, process HTTP requests and responses, and supports a variety of databases. 2.PHP is used to generate dynamic web content, process form data, access databases, etc., with strong community support and open source resources. 3. PHP is an interpreted language, and the execution process includes lexical analysis, grammatical analysis, compilation and execution. 4.PHP can be combined with MySQL for advanced applications such as user registration systems. 5. When debugging PHP, you can use functions such as error_reporting() and var_dump(). 6. Optimize PHP code to use caching mechanisms, optimize database queries and use built-in functions. 7
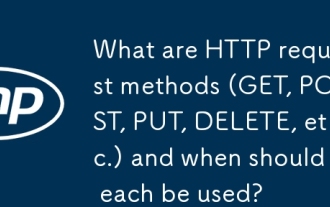
HTTP request methods include GET, POST, PUT and DELETE, which are used to obtain, submit, update and delete resources respectively. 1. The GET method is used to obtain resources and is suitable for read operations. 2. The POST method is used to submit data and is often used to create new resources. 3. The PUT method is used to update resources and is suitable for complete updates. 4. The DELETE method is used to delete resources and is suitable for deletion operations.
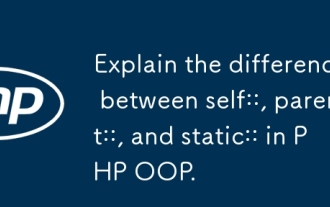
In PHPOOP, self:: refers to the current class, parent:: refers to the parent class, static:: is used for late static binding. 1.self:: is used for static method and constant calls, but does not support late static binding. 2.parent:: is used for subclasses to call parent class methods, and private methods cannot be accessed. 3.static:: supports late static binding, suitable for inheritance and polymorphism, but may affect the readability of the code.
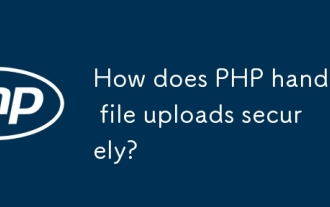
PHP handles file uploads through the $\_FILES variable. The methods to ensure security include: 1. Check upload errors, 2. Verify file type and size, 3. Prevent file overwriting, 4. Move files to a permanent storage location.
