MongoDB Sharding: Scaling Your Database for High Volume Data
MongoDB Sharding is a horizontal scaling technology that improves database performance and capacity by distributing data across multiple servers. 1) Enable Sharding: sh.enableSharding("myDatabase"). 2) Set the shard key: shardCollection("myDatabase.myCollection", { "userId": 1 }). 3) Select the appropriate shard key and block size, optimize query performance and load balancing, and achieve efficient data management and expansion.
introduction
In today's era of data explosion, how to effectively manage and scale databases has become a challenge for every developer and database administrator. MongoDB Sharding is a horizontally scalable solution that allows us to spread data across multiple servers, thereby improving the performance and capacity of the database. This article will explore in-depth the implementation principles, configuration methods and best practices in practical applications of MongoDB Sharding. By reading this article, you will learn how to use Sharding to deal with the challenges of high-capacity data and master some tips to avoid common problems.
Review of basic knowledge
MongoDB is a document-based NoSQL database that supports rich data models and efficient query operations. Sharding is a data sharding technology provided by MongoDB, which achieves horizontal scaling of the database by dispersing data across multiple nodes. Before understanding Sharding, we need to understand the basic architecture of MongoDB, including the concepts of single nodes, replica sets and sharded clusters.
In MongoDB, data is stored in a collection, and the document in the collection is the basic unit of data. Sharding implements distributed storage and querying of data by dispersing documents in a collection onto different shards.
Core concept or function analysis
The definition and function of MongoDB Sharding
MongoDB Sharding is a technology that divides data horizontally and distributes it on multiple servers. Its main function is to improve the scalability and performance of the database. With Sharding, we can disperse data across multiple physical servers, thus avoiding a single server becoming a performance bottleneck.
A simple sharding example:
// Configure the sharding key sh.enableSharding("myDatabase") sh.shardCollection("myDatabase.myCollection", { "userId": 1 })
In this example, we enable Sharding for myDatabase
and set userId
as sharding key for myCollection
collection. The shard key determines how data is distributed among shards.
How it works
The working principle of MongoDB Sharding can be divided into the following steps:
Sharding key selection : Selecting a suitable sharding key is the key to Sharding. The shard key determines how data is distributed among shards, affecting query performance and data balance.
Data sharding : MongoDB divides data into multiple blocks (Chunks) according to the shard key, each block contains a portion of data. The size of the block can be adjusted by configuration, and the default size is 64MB.
Sharding Management : MongoDB uses a configuration server (Config Server) and a router (Mongos) to manage sharding. The server is configured to store shard metadata, and the router is responsible for routing client requests to the correct shard.
Query processing : When the client initiates a query request, Mongos will distribute the request to the relevant shard based on the query conditions and shard keys. Each shard processes the query request independently and returns the result to Mongos, and finally returns the result to the client by Mongos.
The implementation principle of Sharding involves multiple aspects such as data distribution, load balancing and query optimization. Choosing the right sharding key and block size is the key to optimizing Sharding performance, while taking into account data growth and query patterns.
Example of usage
Basic usage
Configuring MongoDB Sharding requires the following steps:
// Enable Sharding sh.enableSharding("myDatabase") // Set shardCollection("myDatabase.myCollection", { "userId": 1 })
In this example, we first enable Sharding for the database myDatabase
, and then set userId
as sharding key for the collection myCollection
. userId
is selected as the shard key because it has high uniqueness and uniform distribution in the data.
Advanced Usage
In practical applications, we may need to select different shard keys and block sizes according to different query modes and data distribution. For example, if we need to query data frequently by time range, we can select the time field as the shard key:
// Use the time field as the shard key sh.shardCollection("myDatabase.logs", { "timestamp": 1 })
In this example, we set timestamp
as shard key for logs
collection, which can better support queries by time range.
Common Errors and Debugging Tips
When using MongoDB Sharding, common errors include improper selection of shard keys, unreasonable block size settings, etc. Here are some debugging tips:
Shard key selection : When selecting shard keys, you need to consider the distribution of data and query mode. Avoid selecting fields with low uniqueness or uneven distribution as shard keys.
Block size adjustment : If the block size is set too large, it may cause uneven data distribution; if the setting is too small, it may increase management overhead. You can view the current block size through
sh.status()
command and adjust it according to the actual situation.Query Performance Optimization : In a Sharding environment, query performance may be affected. You can analyze the query plan through the
explain()
command to optimize query conditions and indexes.
Performance optimization and best practices
In practical applications, the following aspects need to be considered:
Sharding key optimization : Choosing the right sharding key is the key to optimizing Sharding performance. It is necessary to select fields with high uniqueness and uniform distribution as shard keys based on the data distribution and query mode.
Block size adjustment : Adjust the block size in time according to the data growth and query mode. You can manually split blocks through the
sh.splitAt()
command to achieve balanced data distribution.Query Optimization : In a Sharding environment, query performance may be affected. You can analyze the query plan through the
explain()
command to optimize query conditions and indexes. At the same time, you can use thehint()
command to specify the index to improve query performance.Load balancing : MongoDB provides automatic load balancing function, which can achieve balanced data distribution through
balancer
process. The start-stop of the load balancer can be controlled throughsh.startBalancer()
andsh.stopBalancer()
commands.Monitoring and maintenance : Regularly monitor the performance and status of the Sharding cluster to discover and resolve problems in a timely manner. You can view the real-time status of the cluster through
mongotop
andmongostat
commands, and optimize configuration and resource allocation.
Through the above methods, we can effectively optimize the performance of MongoDB Sharding and realize the scaling and management of high-capacity data. In actual applications, Sharding configuration and optimization strategies need to be flexibly adjusted according to specific business needs and data characteristics.
In short, MongoDB Sharding, as a powerful horizontal scaling technology, provides us with solutions to efficiently manage and scale databases. By deeply understanding the principles and best practices of Sharding, we can better address the challenges of high-capacity data and achieve database scalability and high performance.
The above is the detailed content of MongoDB Sharding: Scaling Your Database for High Volume Data. For more information, please follow other related articles on the PHP Chinese website!

Hot AI Tools

Undresser.AI Undress
AI-powered app for creating realistic nude photos

AI Clothes Remover
Online AI tool for removing clothes from photos.

Undress AI Tool
Undress images for free

Clothoff.io
AI clothes remover

Video Face Swap
Swap faces in any video effortlessly with our completely free AI face swap tool!

Hot Article

Hot Tools

Notepad++7.3.1
Easy-to-use and free code editor

SublimeText3 Chinese version
Chinese version, very easy to use

Zend Studio 13.0.1
Powerful PHP integrated development environment

Dreamweaver CS6
Visual web development tools

SublimeText3 Mac version
God-level code editing software (SublimeText3)

Hot Topics
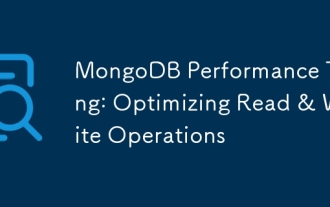
The core strategies of MongoDB performance tuning include: 1) creating and using indexes, 2) optimizing queries, and 3) adjusting hardware configuration. Through these methods, the read and write performance of the database can be significantly improved, response time, and throughput can be improved, thereby optimizing the user experience.
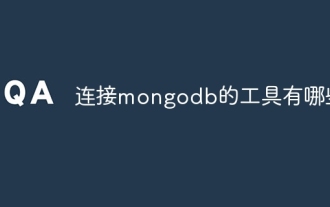
The main tools for connecting to MongoDB are: 1. MongoDB Shell, suitable for quickly viewing data and performing simple operations; 2. Programming language drivers (such as PyMongo, MongoDB Java Driver, MongoDB Node.js Driver), suitable for application development, but you need to master the usage methods; 3. GUI tools (such as Robo 3T, Compass) provide a graphical interface for beginners and quick data viewing. When selecting tools, you need to consider application scenarios and technology stacks, and pay attention to connection string configuration, permission management and performance optimization, such as using connection pools and indexes.
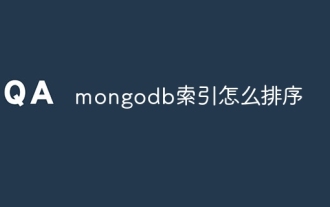
Sorting index is a type of MongoDB index that allows sorting documents in a collection by specific fields. Creating a sort index allows you to quickly sort query results without additional sorting operations. Advantages include quick sorting, override queries, and on-demand sorting. The syntax is db.collection.createIndex({ field: <sort order> }), where <sort order> is 1 (ascending order) or -1 (descending order). You can also create multi-field sorting indexes that sort multiple fields.
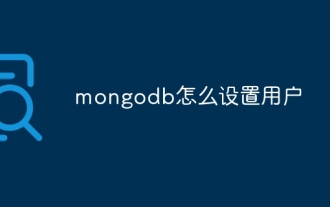
To set up a MongoDB user, follow these steps: 1. Connect to the server and create an administrator user. 2. Create a database to grant users access. 3. Use the createUser command to create a user and specify their role and database access rights. 4. Use the getUsers command to check the created user. 5. Optionally set other permissions or grant users permissions to a specific collection.
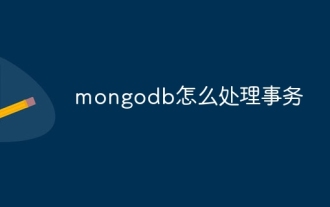
Transaction processing in MongoDB provides solutions such as multi-document transactions, snapshot isolation, and external transaction managers to achieve transaction behavior, ensure multiple operations are executed as one atomic unit, ensuring atomicity and isolation. Suitable for applications that need to ensure data integrity, prevent concurrent operational data corruption, or implement atomic updates in distributed systems. However, its transaction processing capabilities are limited and are only suitable for a single database instance. Multi-document transactions only support read and write operations. Snapshot isolation does not provide atomic guarantees. Integrating external transaction managers may also require additional development work.
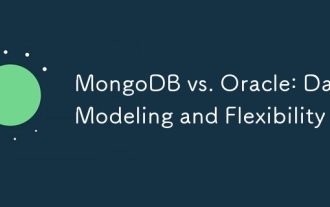
MongoDB is more suitable for processing unstructured data and rapid iteration, while Oracle is more suitable for scenarios that require strict data consistency and complex queries. 1.MongoDB's document model is flexible and suitable for handling complex data structures. 2. Oracle's relationship model is strict to ensure data consistency and complex query performance.
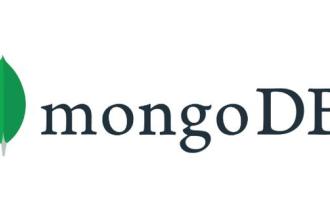
Choosing MongoDB or relational database depends on application requirements. 1. Relational databases (such as MySQL) are suitable for applications that require high data integrity and consistency and fixed data structures, such as banking systems; 2. NoSQL databases such as MongoDB are suitable for processing massive, unstructured or semi-structured data and have low requirements for data consistency, such as social media platforms. The final choice needs to weigh the pros and cons and decide based on the actual situation. There is no perfect database, only the most suitable database.
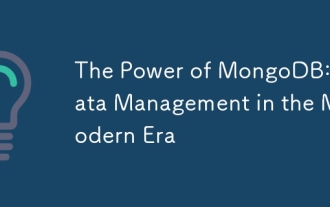
MongoDB is a NoSQL database because of its flexibility and scalability are very important in modern data management. It uses document storage, is suitable for processing large-scale, variable data, and provides powerful query and indexing capabilities.
