


Practical application cases of optimism and pessimistic locks in business
The choice of optimistic locks and pessimistic locks depends on business scenarios and data consistency requirements. 1. Pessimistic locks assume data conflicts, and locks ensure data consistency, but low efficiency under high concurrency, such as bank transfers; 2. Optimistic locks assume data conflict probability is low, and no locks are added, check whether the data is modified before update, with high efficiency but data inconsistency, such as e-commerce inventory management and forum comments; 3. In high concurrency scenarios, you can consider combining optimistic locks and pessimistic locks, first pre-processing of optimistic locks, and finally confirming pessimistic locks, taking into account efficiency and data consistency. The final choice requires the trade-off between efficiency and data consistency.
Optimistic lock and pessimistic lock: Trade-offs and choices in business practices
Optimistic lock and pessimistic lock, these two concepts sound mysterious, but in fact they are two completely different strategies when dealing with concurrent access to databases. Simply put, optimistic locks believe that "data generally does not conflict", while pessimistic locks believe that "data is likely to conflict". This article will not give you a boring definition, but will take you into the business scenarios to see how they play and how to choose the right solution based on actual conditions. After reading it, you can control these two lock mechanisms like an old driver based on business needs.
Let’s start with the basics. Pessimistic lock, as the name suggests, always assumes the worst case – concurrent modification. In order to avoid data conflicts, it will directly lock the data when accessing data. Typical examples are the transaction isolation level of the database and the mutex mechanism provided by some programming languages. Imagine bank account transfers. Pessimistic locks are like a strict security guard. Only one user can enter the operation at a time, and others can only wait in line. This ensures the consistency of the data, but efficiency... You know, especially when the concurrency is large, the waiting time will be long.
Optimistic locks are completely different. It believes that the probability of data conflict is very low, so it will not actively add locks. It will check whether the data has been modified before updating it. If it has not been modified, it will be updated; if it has been modified, it will prompt a conflict and let the user re-operate. This is like a flexible administrator, which allows multiple users to view and modify data simultaneously, and only perform verification when submitting modifications. This is much more efficient, but there are risks, that is, "dirty writing" may occur and needs to be handled with caution.
Let’s look at some actual cases.
Case 1: E-commerce product inventory management
Commodity inventory is a typical concurrent scenario. If you use pessimistic locks, you need to add a lock every time the user visits the product page or even just checks the inventory, which will lead to a serious performance bottleneck. And optimistic locking is very suitable. We can use the version number mechanism to achieve optimistic locking: each product has a version number, and each time the inventory is updated, check whether the version number is consistent. If it is inconsistent, it means that the data has been modified and update is refused. It's like a label posted on the product inventory, recording the number of modifications, and only when the label has not changed can it be modified.
<code class="python">class Product:</code><pre class='brush:php;toolbar:false;'> def __init__(self, id, name, stock, version): self.id = id self.name = name self.stock = stock self.version = version def update_stock(self, new_stock, current_version): if self.version == current_version: self.stock = new_stock self.version = 1 return True # Update successful else: return False # Update failed, data has been changed
Simulate concurrent updates
product = Product(1, "iPhone", 100, 1)
thread1 = threading.Thread(target=lambda: product.update_stock(90, 1))
thread2 = threading.Thread(target=lambda: product.update_stock(80, 1))
thread1.start()
thread2.start()
thread1.join()
thread2.join()
print(f"final inventory: {product.stock}") #The result may not be 80 or 90, depending on the order of thread execution, showing the possible problems with optimistic locks
This code uses Python to simulate the implementation of optimistic locks. Note that this is just a simplified version, and issues such as the atomicity of database transactions need to be considered in actual applications. Have you seen it? Although optimistic locking is efficient, it may lead to data inconsistency and requires appropriate mechanisms to deal with conflicts.
Case 2: Forum post comments
Forum post comments, the concurrency volume is also very large. If you use pessimistic locks, every comment needs to be locked, which is too inefficient. Optimistic locks apply here too. We can use a mechanism similar to the version number, or use a timestamp to determine whether the data has been modified.
Case 3: Bank Transfer (Another emphasized)
As mentioned above, pessimistic locking seems to be a safer choice because it can ensure the consistency of data. However, if the concurrency volume is extremely high, the performance bottleneck of pessimistic locking will be very obvious. At this time, we can consider combining optimistic locks and pessimistic locks. For example, using optimistic locks for preprocessing in high concurrency scenarios, and using pessimistic locks for final confirmation only when the last commit is submitted. This can ensure both efficiency and data consistency. This requires more complex strategies and design.
In short, there is no absolute good or bad for optimistic locks and pessimistic locks. Which strategy to choose depends on the specific business scenario and the requirements for data consistency. In high concurrency scenarios, optimistic locks are usually more efficient, but data conflicts need to be handled with caution; while in scenarios with extremely high requirements for data consistency, pessimistic locks are more secure, but performance may become a bottleneck. When making a choice, you need to weigh efficiency and data consistency, and choose the appropriate solution according to the actual situation, or even use it in combination. Remember, there is no silver bullet, only suitable solutions. I wish you become a lock mechanism master!
The above is the detailed content of Practical application cases of optimism and pessimistic locks in business. For more information, please follow other related articles on the PHP Chinese website!

Hot AI Tools

Undresser.AI Undress
AI-powered app for creating realistic nude photos

AI Clothes Remover
Online AI tool for removing clothes from photos.

Undress AI Tool
Undress images for free

Clothoff.io
AI clothes remover

AI Hentai Generator
Generate AI Hentai for free.

Hot Article

Hot Tools

Notepad++7.3.1
Easy-to-use and free code editor

SublimeText3 Chinese version
Chinese version, very easy to use

Zend Studio 13.0.1
Powerful PHP integrated development environment

Dreamweaver CS6
Visual web development tools

SublimeText3 Mac version
God-level code editing software (SublimeText3)

Hot Topics


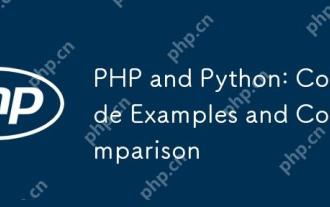
PHP and Python have their own advantages and disadvantages, and the choice depends on project needs and personal preferences. 1.PHP is suitable for rapid development and maintenance of large-scale web applications. 2. Python dominates the field of data science and machine learning.
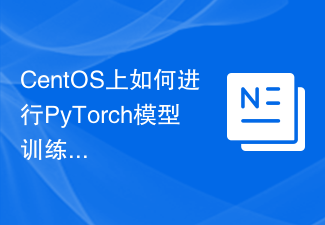
Efficient training of PyTorch models on CentOS systems requires steps, and this article will provide detailed guides. 1. Environment preparation: Python and dependency installation: CentOS system usually preinstalls Python, but the version may be older. It is recommended to use yum or dnf to install Python 3 and upgrade pip: sudoyumupdatepython3 (or sudodnfupdatepython3), pip3install--upgradepip. CUDA and cuDNN (GPU acceleration): If you use NVIDIAGPU, you need to install CUDATool
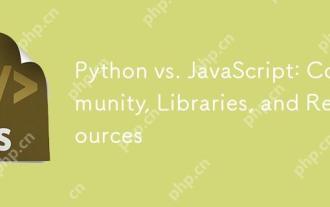
Python and JavaScript have their own advantages and disadvantages in terms of community, libraries and resources. 1) The Python community is friendly and suitable for beginners, but the front-end development resources are not as rich as JavaScript. 2) Python is powerful in data science and machine learning libraries, while JavaScript is better in front-end development libraries and frameworks. 3) Both have rich learning resources, but Python is suitable for starting with official documents, while JavaScript is better with MDNWebDocs. The choice should be based on project needs and personal interests.
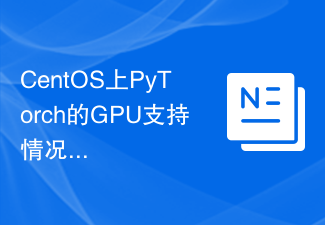
Enable PyTorch GPU acceleration on CentOS system requires the installation of CUDA, cuDNN and GPU versions of PyTorch. The following steps will guide you through the process: CUDA and cuDNN installation determine CUDA version compatibility: Use the nvidia-smi command to view the CUDA version supported by your NVIDIA graphics card. For example, your MX450 graphics card may support CUDA11.1 or higher. Download and install CUDAToolkit: Visit the official website of NVIDIACUDAToolkit and download and install the corresponding version according to the highest CUDA version supported by your graphics card. Install cuDNN library:
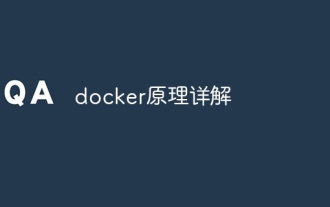
Docker uses Linux kernel features to provide an efficient and isolated application running environment. Its working principle is as follows: 1. The mirror is used as a read-only template, which contains everything you need to run the application; 2. The Union File System (UnionFS) stacks multiple file systems, only storing the differences, saving space and speeding up; 3. The daemon manages the mirrors and containers, and the client uses them for interaction; 4. Namespaces and cgroups implement container isolation and resource limitations; 5. Multiple network modes support container interconnection. Only by understanding these core concepts can you better utilize Docker.
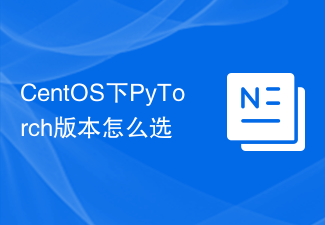
When selecting a PyTorch version under CentOS, the following key factors need to be considered: 1. CUDA version compatibility GPU support: If you have NVIDIA GPU and want to utilize GPU acceleration, you need to choose PyTorch that supports the corresponding CUDA version. You can view the CUDA version supported by running the nvidia-smi command. CPU version: If you don't have a GPU or don't want to use a GPU, you can choose a CPU version of PyTorch. 2. Python version PyTorch
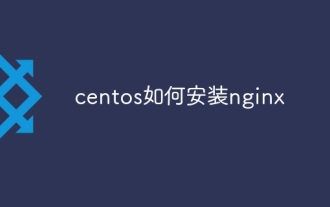
CentOS Installing Nginx requires following the following steps: Installing dependencies such as development tools, pcre-devel, and openssl-devel. Download the Nginx source code package, unzip it and compile and install it, and specify the installation path as /usr/local/nginx. Create Nginx users and user groups and set permissions. Modify the configuration file nginx.conf, and configure the listening port and domain name/IP address. Start the Nginx service. Common errors need to be paid attention to, such as dependency issues, port conflicts, and configuration file errors. Performance optimization needs to be adjusted according to the specific situation, such as turning on cache and adjusting the number of worker processes.
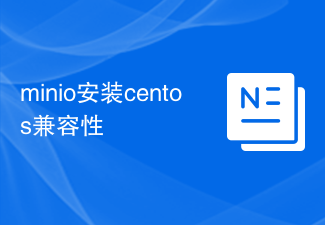
MinIO Object Storage: High-performance deployment under CentOS system MinIO is a high-performance, distributed object storage system developed based on the Go language, compatible with AmazonS3. It supports a variety of client languages, including Java, Python, JavaScript, and Go. This article will briefly introduce the installation and compatibility of MinIO on CentOS systems. CentOS version compatibility MinIO has been verified on multiple CentOS versions, including but not limited to: CentOS7.9: Provides a complete installation guide covering cluster configuration, environment preparation, configuration file settings, disk partitioning, and MinI
