Can mysql handle big data
MySQL can handle big data, but requires skills and strategies. Splitting databases and tables is the key, splitting large databases or large tables into smaller units. The application logic needs to be adjusted to access the data correctly, and routing can be achieved through a consistent hash or a database proxy. After the database is divided into different tables, transaction processing and data consistency will become complicated, and the routing logic and data distribution need to be carefully examined during debugging. Performance optimization includes selecting the right hardware, using database connection pools, optimizing SQL statements, and adding caches.
Can MySQL handle big data? This question is so good, there is no standard answer, just like asking "how far a bicycle can go", it depends on many factors. Simply saying "can" or "can't" is too arbitrary.
Let’s first talk about the word “big data”. For a small e-commerce website, million-level data may be a tough one, but for a large Internet company, million-level data may not even be considered a fraction of it. Therefore, the definition of big data is relative and depends on your application scenario and hardware resources.
So can MySQL deal with big data? The answer is: Yes, but skills and strategies are required . Don't expect MySQL to easily process Pega-level data like Hadoop or Spark, but after reasonable design and optimization, it is not impossible to process TB-level data.
To put it bluntly, MySQL's own architecture determines that it is more suitable for processing structured data and is good at online transaction processing (OLTP). It is not a natural big data processing tool, but we can use some means to improve its processing power.
Basic knowledge review: You have to first understand the difference between MySQL's storage engines, such as InnoDB and MyISAM. InnoDB supports transactions and line locks, which is more suitable for OLTP scenarios, but it will sacrifice some performance; MyISAM does not support transactions, but reads and writes faster, which is suitable for data that is read only or written once. In addition, the use of indexes is also key. A good index can significantly improve query efficiency.
Core concept: Distribution of databases and tables This is the key to dealing with big data. Splitting a huge database into multiple small databases, or splitting a huge table into multiple small tables is the most commonly used strategy. You can divide the library into tables according to different business logic or data characteristics, such as divide the library into tables by user ID, divide the library into tables by region, etc. This requires careful design, otherwise it will cause many problems.
Working principle: After dividing databases and tables, your application logic needs to be adjusted accordingly in order to correctly access the data. You need a routing layer to decide which request should access which database or table. Commonly used methods include: consistency hashing, database proxy, etc. Which method to choose depends on your specific needs and technology stack.
Example of usage: Suppose you have a user table with a data volume of tens of millions. You can divide the table by the hash value of the user ID, such as moduloing the user ID to 10 and dividing it into 10 tables. In this way, the amount of data in each table is reduced by ten times. Of course, this is just the simplest example, and more complex strategies may be required in practical applications.
My code examples would be more "alternative" because I don't like the same-sized code. I will write a simple routing logic in Python. Of course, in actual applications you will use a more mature solution:
<code class="python">def get_table_name(user_id): # 简单的哈希路由,实际应用中需要更复杂的逻辑return f"user_table_{user_id % 10}" # 模拟数据库操作def query_user(user_id, db_conn): table_name = get_table_name(user_id) # 这里应该使用数据库连接池,避免频繁创建连接cursor = db_conn.cursor() cursor.execute(f"SELECT * FROM {table_name} WHERE id = {user_id}") return cursor.fetchone()</code>
Common errors and debugging techniques: After dividing libraries and tables, transaction processing will become complicated. Cross-library transactions require special processing methods, such as two-stage commits. In addition, data consistency is also a key issue. When debugging, you need to carefully check your routing logic and data distribution.
Performance optimization and best practices: Selecting the right hardware, using database connection pools, optimizing SQL statements, using caches, etc. These are common ways to improve performance. Remember, the readability and maintainability of the code are also important. Don't write difficult code to understand in order to pursue the ultimate performance.
In short, it is not impossible for MySQL to process big data, but it requires you to put in more effort and thinking. It is not a silver bullet, you need to choose the right tools and strategies based on the actual situation. Don’t be intimidated by the word “big data”. You can always find a solution when you take it step by step.
The above is the detailed content of Can mysql handle big data. For more information, please follow other related articles on the PHP Chinese website!

Hot AI Tools

Undresser.AI Undress
AI-powered app for creating realistic nude photos

AI Clothes Remover
Online AI tool for removing clothes from photos.

Undress AI Tool
Undress images for free

Clothoff.io
AI clothes remover

Video Face Swap
Swap faces in any video effortlessly with our completely free AI face swap tool!

Hot Article

Hot Tools

Notepad++7.3.1
Easy-to-use and free code editor

SublimeText3 Chinese version
Chinese version, very easy to use

Zend Studio 13.0.1
Powerful PHP integrated development environment

Dreamweaver CS6
Visual web development tools

SublimeText3 Mac version
God-level code editing software (SublimeText3)

Hot Topics
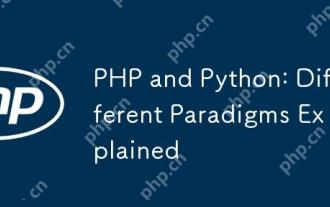
PHP is mainly procedural programming, but also supports object-oriented programming (OOP); Python supports a variety of paradigms, including OOP, functional and procedural programming. PHP is suitable for web development, and Python is suitable for a variety of applications such as data analysis and machine learning.
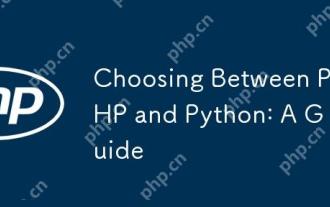
PHP is suitable for web development and rapid prototyping, and Python is suitable for data science and machine learning. 1.PHP is used for dynamic web development, with simple syntax and suitable for rapid development. 2. Python has concise syntax, is suitable for multiple fields, and has a strong library ecosystem.
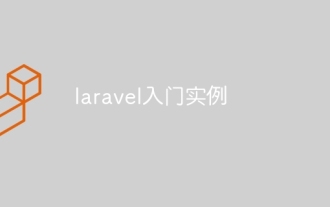
Laravel is a PHP framework for easy building of web applications. It provides a range of powerful features including: Installation: Install the Laravel CLI globally with Composer and create applications in the project directory. Routing: Define the relationship between the URL and the handler in routes/web.php. View: Create a view in resources/views to render the application's interface. Database Integration: Provides out-of-the-box integration with databases such as MySQL and uses migration to create and modify tables. Model and Controller: The model represents the database entity and the controller processes HTTP requests.
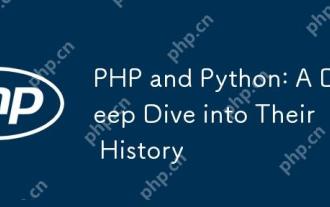
PHP originated in 1994 and was developed by RasmusLerdorf. It was originally used to track website visitors and gradually evolved into a server-side scripting language and was widely used in web development. Python was developed by Guidovan Rossum in the late 1980s and was first released in 1991. It emphasizes code readability and simplicity, and is suitable for scientific computing, data analysis and other fields.
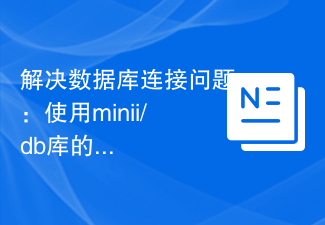
I encountered a tricky problem when developing a small application: the need to quickly integrate a lightweight database operation library. After trying multiple libraries, I found that they either have too much functionality or are not very compatible. Eventually, I found minii/db, a simplified version based on Yii2 that solved my problem perfectly.
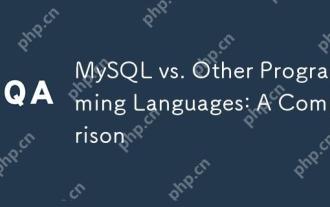
Compared with other programming languages, MySQL is mainly used to store and manage data, while other languages such as Python, Java, and C are used for logical processing and application development. MySQL is known for its high performance, scalability and cross-platform support, suitable for data management needs, while other languages have advantages in their respective fields such as data analytics, enterprise applications, and system programming.
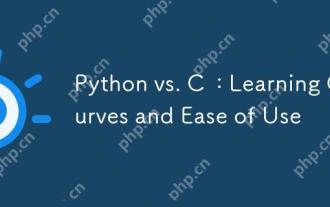
Python is easier to learn and use, while C is more powerful but complex. 1. Python syntax is concise and suitable for beginners. Dynamic typing and automatic memory management make it easy to use, but may cause runtime errors. 2.C provides low-level control and advanced features, suitable for high-performance applications, but has a high learning threshold and requires manual memory and type safety management.
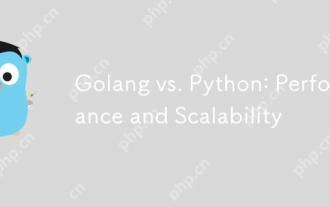
Golang is better than Python in terms of performance and scalability. 1) Golang's compilation-type characteristics and efficient concurrency model make it perform well in high concurrency scenarios. 2) Python, as an interpreted language, executes slowly, but can optimize performance through tools such as Cython.
