Understanding SciPy Library in Python
Introduction
Imagine you're a scientist or engineer tackling complex problems – differential equations, optimization challenges, or Fourier analysis. Python's ease of use and graphics capabilities are appealing, but these tasks demand powerful tools. Enter SciPy, an open-source Python library for scientific and numerical computation. SciPy simplifies data processing, equation solving, Fourier transforms, and much more, making scientific computing efficient and accessible.
Key Learning Points
This guide will cover:
- SciPy's role in scientific computing.
- Installation and importing SciPy into your Python environment.
- Exploring SciPy's core modules and capabilities.
- Practical examples of SciPy applications.
- Understanding SciPy's advantages in various scientific and engineering fields.
Table of Contents
- What is SciPy?
- SciPy's Applications
- SciPy vs. Other Libraries
- Installing SciPy
- Core SciPy Modules
- Real-World SciPy Examples
- Frequently Asked Questions
What is SciPy?
SciPy (pronounced "Sigh Pie") stands for Scientific Python. It's an open-source Python library designed for scientific and technical computation. Built as an extension to NumPy, it provides high-level tools for scientific and engineering applications.
Why Choose SciPy?
SciPy enhances Python's capabilities for numerical computation, offering a robust and efficient toolkit. Its key benefits include:
- Extensive Functionality: SciPy offers modules for optimization, integration, interpolation, eigenvalue problems, equation solving, signal processing, and much more. It provides solutions that would otherwise require significant development effort.
- Performance and Efficiency: SciPy's functions are optimized for speed and efficiency, especially when handling large datasets. Many routines leverage established, high-performance algorithms.
- User-Friendliness: SciPy's functions are designed for ease of use, particularly when combined with NumPy. Its intuitive interface makes it accessible to users of all programming skill levels.
- Open Source and Community Support: As an open-source project, SciPy benefits from a large and active community of developers and researchers, ensuring ongoing development and support.
Where and How Can We Use SciPy?
SciPy finds applications in numerous fields requiring scientific and technical computation:
-
Data Analysis:
scipy.stats
provides statistical functions for probability calculations and hypothesis testing, along with tools for managing and analyzing large datasets. - Engineering: SciPy is used for signal processing, solving differential equations, and modeling engineering systems.
-
Optimization: The
scipy.optimize
module offers methods for finding function extrema, crucial for machine learning, economics, and operations research. - Physics and Astronomy: SciPy aids in simulating physical processes, solving partial differential equations, and modeling celestial mechanics.
- Finance: Applications include portfolio optimization, option pricing (Black-Scholes model), and time series analysis.
- Machine Learning: While dedicated machine learning libraries exist, SciPy provides fundamental functions for optimization, linear algebra, and statistical distributions, supporting model creation and evaluation.
How is SciPy Different from Other Libraries?
SciPy distinguishes itself in several ways:
- NumPy Foundation: SciPy extends NumPy's array capabilities, adding advanced scientific computing tools. NumPy focuses on array operations, while SciPy incorporates algorithms and models.
- Broad Scope: Unlike specialized libraries like Pandas (data manipulation) or Matplotlib (visualization), SciPy offers comprehensive coverage across multiple scientific computing domains.
- Community-Driven Development: SciPy's community-driven development ensures responsiveness to the evolving needs of the scientific community.
- Seamless Integration: SciPy integrates well with other Python libraries, enabling complex workflows combining multiple tools (e.g., SciPy with Matplotlib for visualization or Pandas for data manipulation).
How to Install SciPy?
Installing SciPy is straightforward. This guide outlines the process, verification steps, and troubleshooting tips.
Prerequisites
Before installing SciPy, ensure you have Python 3.7 or later and NumPy installed. Most Python distributions include pip
, the package manager used for installation. Check your installations using:
python --version pip --version
If Python or pip
is missing, download them from python.org and follow the installation instructions.
Installing with pip
The easiest way to install SciPy is using pip
:
Step 1: Open your terminal or command prompt.
Step 2: Run the installation command:
pip install scipy
pip
automatically installs SciPy and its dependencies, including NumPy if needed.
Step 3: Verify the installation:
Open a Python shell and import SciPy:
import scipy print(scipy.__version__)
A successful installation displays the SciPy version number.
Core Modules in SciPy
SciPy's modular structure provides specialized functions for various computations. Here's a summary of core modules and their uses:
-
scipy.cluster
: Clustering algorithms (hierarchical, k-means). -
scipy.constants
: Physical and mathematical constants and units. -
scipy.fft
: Fast Fourier transforms (FFT). -
scipy.integrate
: Integration and ordinary differential equation (ODE) solvers. -
scipy.interpolate
: Interpolation methods. -
scipy.io
: Input/output functions for various file formats (MATLAB, WAV, etc.). -
scipy.linalg
: Linear algebra routines (matrix decompositions, solving linear systems). -
scipy.ndimage
: Multi-dimensional image processing. -
scipy.optimize
: Optimization and root-finding algorithms. -
scipy.signal
: Signal processing tools (filtering, Fourier transforms, system analysis). -
scipy.sparse
: Sparse matrix operations. -
scipy.spatial
: Spatial data structures and algorithms. -
scipy.special
: Special functions (Bessel, gamma, error functions, etc.). -
scipy.stats
: Statistical functions, hypothesis testing, probability distributions.
Applications of SciPy
Let's explore some practical SciPy applications:
Optimization
SciPy's optimize
module solves optimization problems using methods like minimize
, curve_fit
, and least_squares
.
Example:
from scipy.optimize import minimize def objective_function(x): return x**2 2*x 1 result = minimize(objective_function, 0) print(result)
Integration
The integrate
module provides integration techniques (quad
, dblquad
, tplquad
for single, double, and triple integrals).
Example:
from scipy.integrate import quad result, error = quad(lambda x: x**2, 0, 1) print(result)
Signal Processing
The signal
module offers tools for filtering, convolution, and Fourier transforms.
Example: (Illustrative - requires data)
from scipy import signal # ... (load signal data) ... filtered_signal = signal.medfilt(signal_data, kernel_size=5)
Linear Algebra
The linalg
module handles linear algebra problems (matrix inversion, decomposition, solving linear systems).
Example:
from scipy.linalg import lu import numpy as np A = np.array([[1, 2, 3], [4, 5, 6], [7, 8, 10]]) P, L, U = lu(A) print(L)
Statistics
The stats
module provides statistical analysis tools (probability calculations, hypothesis testing, working with distributions).
Example:
from scipy.stats import norm mean, std_dev = 0, 1 prob = norm.cdf(1, loc=mean, scale=std_dev) print(prob)
Conclusion
SciPy is an indispensable tool for modern scientific computing. It extends Python's capabilities, providing solutions for a wide range of problems, from optimization to signal processing. Whether for academic research or industrial projects, SciPy streamlines computation, allowing you to focus on the science, not the code.
Frequently Asked Questions
Q1: NumPy vs. SciPy? NumPy provides array support and basic math; SciPy builds upon NumPy, adding advanced scientific computation modules.
Q2: Can I use SciPy without NumPy? No, SciPy depends on NumPy.
Q3: Is SciPy suitable for large-scale data analysis? SciPy is well-suited for moderate-scale analysis. For very large datasets, consider integrating it with Pandas or Dask.
Q4: How does SciPy handle optimization? The optimize
module offers various algorithms for minimization, curve fitting, and root finding.
Q5: Is SciPy good for machine learning? SciPy offers some useful tools, but dedicated machine learning libraries (like Scikit-learn) are generally preferred.
The above is the detailed content of Understanding SciPy Library in Python. For more information, please follow other related articles on the PHP Chinese website!

Hot AI Tools

Undresser.AI Undress
AI-powered app for creating realistic nude photos

AI Clothes Remover
Online AI tool for removing clothes from photos.

Undress AI Tool
Undress images for free

Clothoff.io
AI clothes remover

Video Face Swap
Swap faces in any video effortlessly with our completely free AI face swap tool!

Hot Article

Hot Tools

Notepad++7.3.1
Easy-to-use and free code editor

SublimeText3 Chinese version
Chinese version, very easy to use

Zend Studio 13.0.1
Powerful PHP integrated development environment

Dreamweaver CS6
Visual web development tools

SublimeText3 Mac version
God-level code editing software (SublimeText3)

Hot Topics




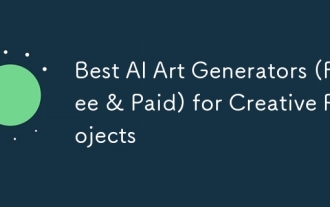
The article reviews top AI art generators, discussing their features, suitability for creative projects, and value. It highlights Midjourney as the best value for professionals and recommends DALL-E 2 for high-quality, customizable art.
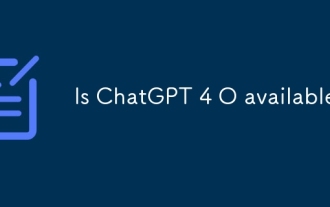
ChatGPT 4 is currently available and widely used, demonstrating significant improvements in understanding context and generating coherent responses compared to its predecessors like ChatGPT 3.5. Future developments may include more personalized interactions and real-time data processing capabilities, further enhancing its potential for various applications.
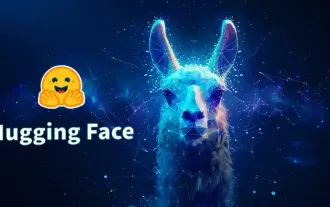
Meta's Llama 3.2: A Leap Forward in Multimodal and Mobile AI Meta recently unveiled Llama 3.2, a significant advancement in AI featuring powerful vision capabilities and lightweight text models optimized for mobile devices. Building on the success o
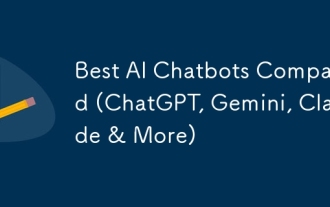
The article compares top AI chatbots like ChatGPT, Gemini, and Claude, focusing on their unique features, customization options, and performance in natural language processing and reliability.
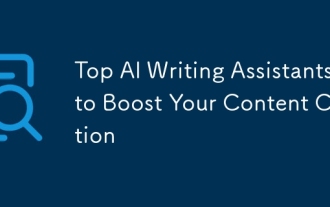
The article discusses top AI writing assistants like Grammarly, Jasper, Copy.ai, Writesonic, and Rytr, focusing on their unique features for content creation. It argues that Jasper excels in SEO optimization, while AI tools help maintain tone consist
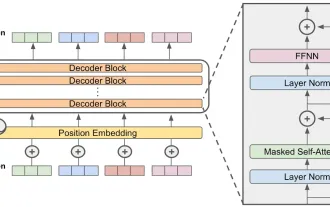
Falcon 3: A Revolutionary Open-Source Large Language Model Falcon 3, the latest iteration in the acclaimed Falcon series of LLMs, represents a significant advancement in AI technology. Developed by the Technology Innovation Institute (TII), this open
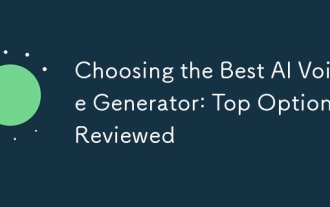
The article reviews top AI voice generators like Google Cloud, Amazon Polly, Microsoft Azure, IBM Watson, and Descript, focusing on their features, voice quality, and suitability for different needs.
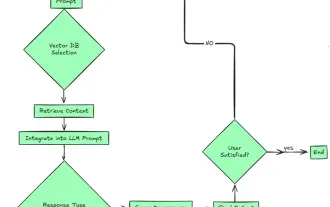
2024 witnessed a shift from simply using LLMs for content generation to understanding their inner workings. This exploration led to the discovery of AI Agents – autonomous systems handling tasks and decisions with minimal human intervention. Buildin
