Vector Streaming: Memory-efficient Indexing with Rust
Introduction
Vector streaming in EmbedAnything is being introduced, a feature designed to optimize large-scale document embedding. Enabling asynchronous chunking and embedding using Rust’s concurrency reduces memory usage and speeds up the process. Today, I will show how to integrate it with the Weaviate Vector Database for seamless image embedding and search.
In my previous article, Supercharge Your Embeddings Pipeline with EmbedAnything, I discussed the idea behind EmbedAnything and how it makes creating embeddings from multiple modalities easy. In this article, I want to introduce a new feature of EmbedAnything called vector streaming and see how it works with Weaviate Vector Database.
Overview
- Vector streaming in EmbedAnything optimizes embedding large-scale documents using asynchronous chunking with Rust’s concurrency.
- It solves memory and efficiency issues in traditional embedding methods by processing chunks in parallel.
- Integration with Weaviate enables seamless embedding and searching in a vector database.
- Implementing vector streaming involves creating a database adapter, initiating an embedding model, and embedding data.
- This approach offers a more efficient, scalable, and flexible solution for large-scale document embedding.
Table of contents
- What is the problem?
- Our Solution to the Problem
- Example Use Case with EmbedAnything
- Step 1: Create the Adapter
- Step 2: Create the Embedding Model
- Step 3: Embed the Directory
- Step 4: Query the Vector Database
- Step 5: Query the Vector Database
- Output
- Frequently Asked Questions
What is the problem?
First, examine the current problem with creating embeddings, especially in large-scale documents. The current embedding frameworks operate on a two-step process: chunking and embedding. First, the text is extracted from all the files, and chunks/nodes are created. Then, these chunks are fed to an embedding model with a specific batch size to process the embeddings. While this is done, the chunks and the embeddings stay on the system memory.
This is not a problem when the files and embedding dimensions are small. But this becomes a problem when there are many files, and you are working with large models and, even worse, multi-vector embeddings. Thus, to work with this, a high RAM is required to process the embeddings. Also, if this is done synchronously, a lot of time is wasted while the chunks are being created, as chunking is not a compute-heavy operation. As the chunks are being made, passing them to the embedding model would be efficient.
Our Solution to the Problem
The solution is to create an asynchronous chunking and embedding task. We can effectively spawn threads to handle this task using Rust’s concurrency patterns and thread safety. This is done using Rust’s MPSC (Multi-producer Single Consumer) module, which passes messages between threads. Thus, this creates a stream of chunks passed into the embedding thread with a buffer. Once the buffer is complete, it embeds the chunks and sends the embeddings back to the main thread, which then sends them to the vector database. This ensures no time is wasted on a single operation and no bottlenecks. Moreover, the system stores only the chunks and embeddings in the buffer, erasing them from memory once they are moved to the vector database.
Example Use Case with EmbedAnything
Now, let’s see this feature in action:
With EmbedAnything, streaming the vectors from a directory of files to the vector database is a simple three-step process.
- Create an adapter for your vector database: This is a wrapper around the database’s functions that allows you to create an index, convert metadata from EmbedAnything’s format to the format required by the database, and the function to insert the embeddings in the index. Adapters for the prominent databases have already been created and are present here.
- Initiate an embedding model of your choice: You can choose from different local models or even cloud models. The configuration can also be determined by setting the chunk size and buffer size for how many embeddings need to be streamed at once. Ideally, this should be as high as possible, but the system RAM limits this.
- Call the embedding function from EmbedAnything: Just pass the directory path to be embedded, the embedding model, the adapter, and the configuration.
In this example, we will embed a directory of images and send it to the vector databases.
Step 1: Create the Adapter
In EmbedAnything, the adapters are created outside so as not to make the library heavy and you get to choose which database you want to work with. Here is a simple adapter for Weaviate:
1 2 3 4 5 6 7 8 9 10 11 12 13 14 15 16 17 18 19 20 21 22 23 24 25 26 27 28 29 30 31 32 33 34 35 36 37 38 39 40 41 42 43 44 45 46 47 48 49 50 51 52 53 54 55 56 57 58 59 60 61 62 63 64 65 66 67 68 69 70 71 72 73 74 75 |
|
Step 2: Create the Embedding Model
Here, since we are embedding images, we can use the clip model
1 2 3 4 5 6 7 |
|
Step 3: Embed the Directory
1 2 3 4 5 6 7 8 9 10 11 |
|
Step 4: Query the Vector Database
1 |
|
Step 5: Query the Vector Database
1 2 3 4 5 6 7 8 9 10 11 |
|
Output
Using the Clip model, we vectorized the whole directory with pictures of cats, dogs, and monkeys. With the simple query “images of cats, ” we were able to search all the files for images of cats.
Check out the notebook for the code here on colab.
Conclusion
I think vector streaming is one of the features that will empower many engineers to opt for a more optimized and no-tech debt solution. Instead of using bulky frameworks on the cloud, you can use a lightweight streaming option.
Check out the GitHub repo over here: EmbedAnything Repo.
Frequently Asked Questions
Q1. What is vector streaming in EmbedAnything?Ans. Vector streaming is a feature that optimizes large-scale document embedding by using Rust’s concurrency for asynchronous chunking and embedding, reducing memory usage and speeding up the process.
Q2. What problem does vector streaming solve?Ans. It addresses high memory usage and inefficiency in traditional embedding methods by processing chunks asynchronously, reducing bottlenecks and optimizing resource use.
Q3. How does vector streaming work with Weaviate?Ans. It uses an adapter to connect EmbedAnything with the Weaviate Vector Database, allowing seamless embedding and querying of data.
Q4. What are the steps for using vector streaming?Ans. Here are steps:
1. Create a database adapter.
2. Initiate an embedding model.
3. Embed the directory.
4. Query the vector database.
Ans. It offers better efficiency, reduced memory usage, scalability, and flexibility compared to traditional embedding methods.
The above is the detailed content of Vector Streaming: Memory-efficient Indexing with Rust. For more information, please follow other related articles on the PHP Chinese website!

Hot AI Tools

Undresser.AI Undress
AI-powered app for creating realistic nude photos

AI Clothes Remover
Online AI tool for removing clothes from photos.

Undress AI Tool
Undress images for free

Clothoff.io
AI clothes remover

Video Face Swap
Swap faces in any video effortlessly with our completely free AI face swap tool!

Hot Article

Hot Tools

Notepad++7.3.1
Easy-to-use and free code editor

SublimeText3 Chinese version
Chinese version, very easy to use

Zend Studio 13.0.1
Powerful PHP integrated development environment

Dreamweaver CS6
Visual web development tools

SublimeText3 Mac version
God-level code editing software (SublimeText3)

Hot Topics










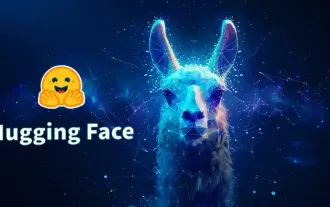
Meta's Llama 3.2: A Leap Forward in Multimodal and Mobile AI Meta recently unveiled Llama 3.2, a significant advancement in AI featuring powerful vision capabilities and lightweight text models optimized for mobile devices. Building on the success o
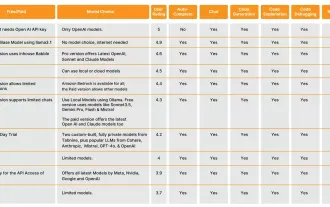
Hey there, Coding ninja! What coding-related tasks do you have planned for the day? Before you dive further into this blog, I want you to think about all your coding-related woes—better list those down. Done? – Let’
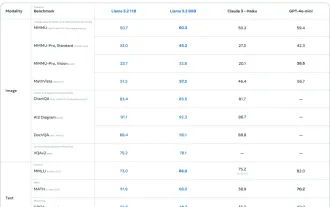
This week's AI landscape: A whirlwind of advancements, ethical considerations, and regulatory debates. Major players like OpenAI, Google, Meta, and Microsoft have unleashed a torrent of updates, from groundbreaking new models to crucial shifts in le
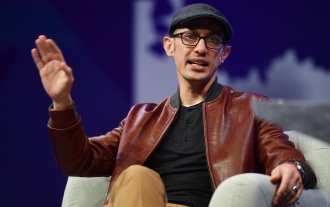
Shopify CEO Tobi Lütke's recent memo boldly declares AI proficiency a fundamental expectation for every employee, marking a significant cultural shift within the company. This isn't a fleeting trend; it's a new operational paradigm integrated into p
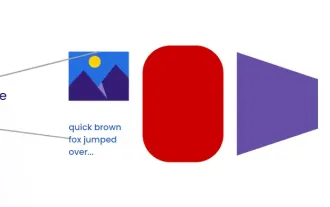
Introduction Imagine walking through an art gallery, surrounded by vivid paintings and sculptures. Now, what if you could ask each piece a question and get a meaningful answer? You might ask, “What story are you telling?
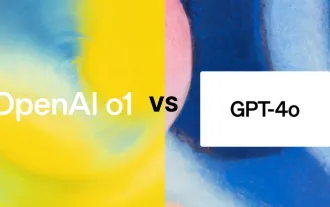
Introduction OpenAI has released its new model based on the much-anticipated “strawberry” architecture. This innovative model, known as o1, enhances reasoning capabilities, allowing it to think through problems mor
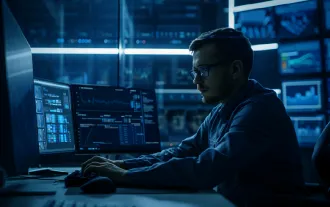
SQL's ALTER TABLE Statement: Dynamically Adding Columns to Your Database In data management, SQL's adaptability is crucial. Need to adjust your database structure on the fly? The ALTER TABLE statement is your solution. This guide details adding colu
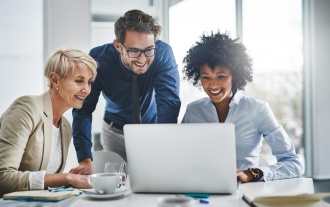
For those of you who might be new to my column, I broadly explore the latest advances in AI across the board, including topics such as embodied AI, AI reasoning, high-tech breakthroughs in AI, prompt engineering, training of AI, fielding of AI, AI re
