What are Diffusion Models?
Dive into the World of Diffusion Models: A Comprehensive Guide
Imagine watching ink bloom across a page, its color subtly diffusing until a captivating pattern emerges. This natural diffusion process, where particles move from high to low concentration, inspires diffusion models in machine learning. Like the spreading ink, these models add and remove noise from data to generate remarkably high-quality results. This article explores diffusion models, their mechanisms, advantages, and applications.
Table of Contents
- What are Diffusion Models?
- How Diffusion Models Function
- Reverse Diffusion: Noise to Image
- Implementation: A Step-by-Step Guide
- Diffusion Model Techniques
- GANs vs. Diffusion Models: A Comparison
- Applications of Diffusion Models
- Data Denoising Explained
- Anomaly Detection and Data Synthesis
- Advantages of Diffusion Models
- Popular Diffusion Tools
- Challenges and Future Directions
- Frequently Asked Questions
What are Diffusion Models?
Diffusion models mimic the natural dispersal of particles. Think of perfume gradually filling a room. In machine learning, they introduce noise to data and then learn to reverse this process, reconstructing the data or creating realistic variations. This gradual refinement leads to highly detailed and accurate outputs, valuable in diverse fields like medical imaging and realistic image/text generation. Their iterative approach allows for nuanced results by mirroring natural diffusion.
How Diffusion Models Function
Diffusion models operate in two phases: a forward phase where noise is added to data, and a reverse phase where this noise is systematically removed. This involves several key stages:
Data Preparation
Before diffusion, data undergoes cleaning, normalization, and augmentation to ensure quality and consistency. This is crucial for effective learning and realistic output generation.
Forward Diffusion: Images to Noise
The forward process starts with a simple distribution (often Gaussian) and adds structured noise incrementally through reversible steps (a Markov chain). This allows the model to learn the intricate patterns within the target data distribution.
Mathematical Formulation (Forward)
Given initial data x₀, the forward process generates noisy versions x₁, x₂, …, xₜ using:
Reverse Diffusion: Noise to Image
The reverse diffusion process cleverly transforms pure noise into a clean image by iteratively removing noise. Training a diffusion model involves learning this reverse process to reconstruct images from noise. Unlike GANs, which perform this in a single step, diffusion models use multiple steps, making training more efficient.
Mathematical Foundation of Reverse Diffusion
The reverse process leverages Markov chains and Gaussian noise, aiming to reconstruct x₀ from xₜ (the final noisy data). This is modeled by:
where μθ(xₜ, t) is the model-predicted mean and σθ²(t) is the variance.
Implementation: A Step-by-Step Guide
Let's outline the implementation steps:
Step 1: Import Libraries
import torch import torch.nn as nn import torch.optim as optim
Step 2: Define the Diffusion Model
class DiffusionModel(nn.Module): # ... (Model architecture as in the original input)
Step 3: Initialize and Train
# ... (Model initialization, optimizer, loss function, and training loop as in the original input)
Diffusion Model Techniques
Several techniques drive diffusion models:
Denoising Diffusion Probabilistic Models (DDPMs)
DDPMs are prominent, training a model to reverse a noise-addition process.
Score-Based Generative Models (SBGMs)
SBGMs utilize score functions (gradients of log probability density) to guide the denoising process.
Stochastic Differential Equations (SDEs)
SDEs model diffusion as continuous-time stochastic processes.
Noise Conditional Score Networks (NCSN)
NCSNs condition the score network on the noise level.
Variational Diffusion Models (VDMs)
VDMs combine diffusion with variational inference.
Implicit Diffusion Models
Implicit models don't explicitly define forward/reverse processes.
Augmented Diffusion Models
These models enhance standard diffusion models with modifications. (See original for details)
GANs vs. Diffusion Models: A Comparison
(Table comparing GANs and Diffusion Models as in the original input)
Applications of Diffusion Models
Diffusion models find applications in:
Image Generation (with code examples as in the original input)
Image-to-Image Translation (with code examples as in the original input)
Data Denoising Explained
(Code example for image denoising as in the original input)
Anomaly Detection and Data Synthesis
(Code example for anomaly detection as in the original input)
Advantages of Diffusion Models
(List of benefits as in the original input)
Popular Diffusion Tools
(List of popular tools as in the original input)
Challenges and Future Directions
(Discussion of challenges and future directions as in the original input)
Conclusion
Diffusion models offer a powerful approach to generative modeling, mimicking natural diffusion processes to create high-quality outputs. Their iterative nature and robust training make them valuable across various applications.
Frequently Asked Questions
(FAQs as in the original input)
The above is the detailed content of What are Diffusion Models?. For more information, please follow other related articles on the PHP Chinese website!

Hot AI Tools

Undresser.AI Undress
AI-powered app for creating realistic nude photos

AI Clothes Remover
Online AI tool for removing clothes from photos.

Undress AI Tool
Undress images for free

Clothoff.io
AI clothes remover

AI Hentai Generator
Generate AI Hentai for free.

Hot Article

Hot Tools

Notepad++7.3.1
Easy-to-use and free code editor

SublimeText3 Chinese version
Chinese version, very easy to use

Zend Studio 13.0.1
Powerful PHP integrated development environment

Dreamweaver CS6
Visual web development tools

SublimeText3 Mac version
God-level code editing software (SublimeText3)

Hot Topics


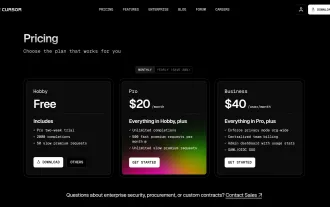
Vibe coding is reshaping the world of software development by letting us create applications using natural language instead of endless lines of code. Inspired by visionaries like Andrej Karpathy, this innovative approach lets dev
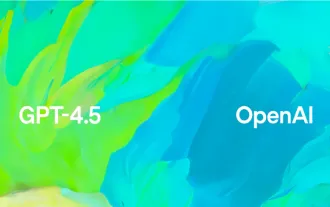
February 2025 has been yet another game-changing month for generative AI, bringing us some of the most anticipated model upgrades and groundbreaking new features. From xAI’s Grok 3 and Anthropic’s Claude 3.7 Sonnet, to OpenAI’s G
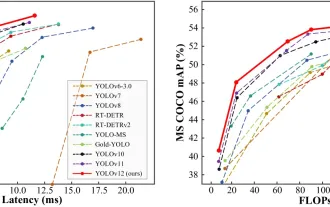
YOLO (You Only Look Once) has been a leading real-time object detection framework, with each iteration improving upon the previous versions. The latest version YOLO v12 introduces advancements that significantly enhance accuracy
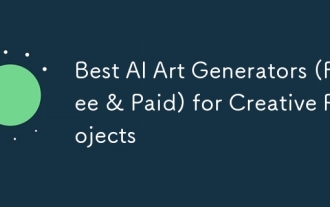
The article reviews top AI art generators, discussing their features, suitability for creative projects, and value. It highlights Midjourney as the best value for professionals and recommends DALL-E 2 for high-quality, customizable art.
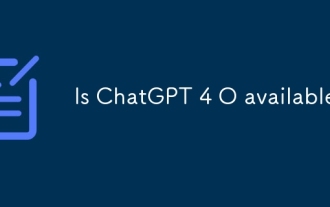
ChatGPT 4 is currently available and widely used, demonstrating significant improvements in understanding context and generating coherent responses compared to its predecessors like ChatGPT 3.5. Future developments may include more personalized interactions and real-time data processing capabilities, further enhancing its potential for various applications.
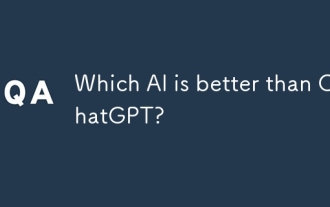
The article discusses AI models surpassing ChatGPT, like LaMDA, LLaMA, and Grok, highlighting their advantages in accuracy, understanding, and industry impact.(159 characters)
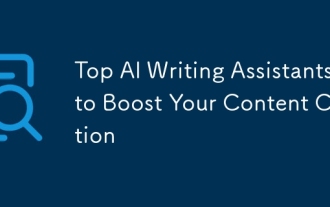
The article discusses top AI writing assistants like Grammarly, Jasper, Copy.ai, Writesonic, and Rytr, focusing on their unique features for content creation. It argues that Jasper excels in SEO optimization, while AI tools help maintain tone consist
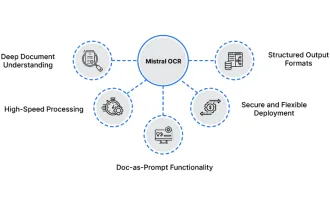
Mistral OCR: Revolutionizing Retrieval-Augmented Generation with Multimodal Document Understanding Retrieval-Augmented Generation (RAG) systems have significantly advanced AI capabilities, enabling access to vast data stores for more informed respons
