How to train PyTorch model on CentOS
Efficient training of PyTorch models on CentOS systems requires steps, and this article will provide detailed guides.
1. Environmental preparation:
Python and dependencies installation: CentOS systems usually preinstall Python, but the version may be older. It is recommended to use
yum
ordnf
to install Python 3 and upgradepip
:sudo yum update python3
(orsudo dnf update python3
),pip3 install --upgrade pip
.CUDA and cuDNN (GPU acceleration): If you use NVIDIA GPU, you need to install the CUDA Toolkit and cuDNN library. Please visit NVIDIA's official website to download the corresponding version of the installation package and strictly follow the official guidelines to install it.
Virtual environment creation (recommended): It is recommended to use
venv
orconda
to create a virtual environment to isolate project dependencies and avoid version conflicts. For example, usevenv
:python3 -m venv myenv
,source myenv/bin/activate
.
2. PyTorch installation:
Visit the PyTorch official website and select the appropriate installation command based on the system configuration (CPU or CUDA version). For example, in the CUDA 11.3 environment:
pip3 install torch torchvision torchaudio --index-url https://download.pytorch.org/whl/cu113
3. Model training process:
Dataset preparation: Prepare the training set and validation set. You can use public data sets or collect data yourself and ensure that the data format is compatible with the model code.
Model code writing: Use PyTorch to write model code, including model architecture, loss functions, and optimizer definitions.
Training model: Run training scripts on CentOS system. Make sure the environment is configured correctly, especially GPU environment variables.
Training process monitoring: Monitor indicators such as loss value and accuracy, and adjust model parameters or training strategies in a timely manner.
Model saving and loading: After training is completed, save model parameters for subsequent loading for inference or continue training.
torch.save(model.state_dict(), 'your_model.pth')
Model Testing: Use the test set to evaluate model performance.
4. Example of PyTorch training loop:
The following is a simplified PyTorch training loop example, which needs to be modified according to actual conditions:
import torch import torch.nn as nn import torch.optim as optim from torch.utils.data import DataLoader from your_dataset import YourDataset # Replace with your dataset class YourModel(nn.Module): def __init__(self): super(YourModel, self).__init__() # ... Model layer definition... def forward(self, x): # ... Forward transmission... Return x train_data = YourDataset(train=True) val_data = YourDataset(train=False) train_loader = DataLoader(train_data, batch_size=32, shuffle=True) val_loader = DataLoader(val_data, batch_size=32, shuffle=False) model = YourModel() criteria = nn.CrossEntropyLoss() optimizer = optim.Adam(model.parameters(), lr=0.001) num_epochs = 10 # training rounds for epoch in range(num_epochs): model.train() for inputs, labels in train_loader: optimizer.zero_grad() outputs = model(inputs) loss = criteria(outputs, labels) loss.backward() optimizer.step() # ... Print training process information... model.eval() with torch.no_grad(): # ... Verify the model, calculate the performance indicators of the verification set... torch.save(model.state_dict(), 'model.pth')
Please modify YourModel
, YourDataset
, loss function, optimizer and training parameters in the code according to your specific model and dataset. Remember to activate the virtual environment before running the code.
The above is the detailed content of How to train PyTorch model on CentOS. For more information, please follow other related articles on the PHP Chinese website!

Hot AI Tools

Undresser.AI Undress
AI-powered app for creating realistic nude photos

AI Clothes Remover
Online AI tool for removing clothes from photos.

Undress AI Tool
Undress images for free

Clothoff.io
AI clothes remover

AI Hentai Generator
Generate AI Hentai for free.

Hot Article

Hot Tools

Notepad++7.3.1
Easy-to-use and free code editor

SublimeText3 Chinese version
Chinese version, very easy to use

Zend Studio 13.0.1
Powerful PHP integrated development environment

Dreamweaver CS6
Visual web development tools

SublimeText3 Mac version
God-level code editing software (SublimeText3)

Hot Topics


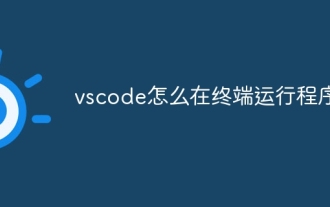
In VS Code, you can run the program in the terminal through the following steps: Prepare the code and open the integrated terminal to ensure that the code directory is consistent with the terminal working directory. Select the run command according to the programming language (such as Python's python your_file_name.py) to check whether it runs successfully and resolve errors. Use the debugger to improve debugging efficiency.
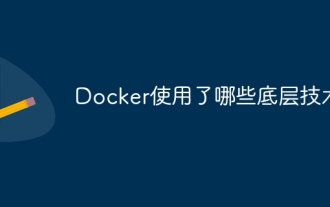
Docker uses container engines, mirror formats, storage drivers, network models, container orchestration tools, operating system virtualization, and container registry to support its containerization capabilities, providing lightweight, portable and automated application deployment and management.
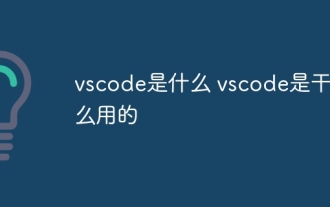
VS Code is the full name Visual Studio Code, which is a free and open source cross-platform code editor and development environment developed by Microsoft. It supports a wide range of programming languages and provides syntax highlighting, code automatic completion, code snippets and smart prompts to improve development efficiency. Through a rich extension ecosystem, users can add extensions to specific needs and languages, such as debuggers, code formatting tools, and Git integrations. VS Code also includes an intuitive debugger that helps quickly find and resolve bugs in your code.
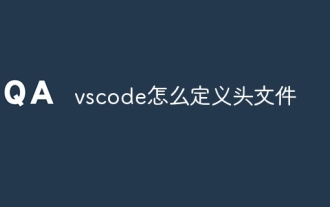
How to define header files using Visual Studio Code? Create a header file and declare symbols in the header file using the .h or .hpp suffix name (such as classes, functions, variables) Compile the program using the #include directive to include the header file in the source file. The header file will be included and the declared symbols are available.
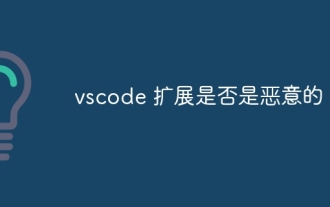
VS Code extensions pose malicious risks, such as hiding malicious code, exploiting vulnerabilities, and masturbating as legitimate extensions. Methods to identify malicious extensions include: checking publishers, reading comments, checking code, and installing with caution. Security measures also include: security awareness, good habits, regular updates and antivirus software.
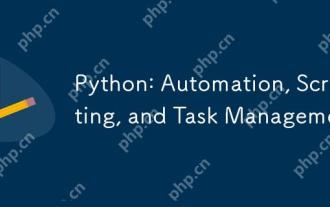
Python excels in automation, scripting, and task management. 1) Automation: File backup is realized through standard libraries such as os and shutil. 2) Script writing: Use the psutil library to monitor system resources. 3) Task management: Use the schedule library to schedule tasks. Python's ease of use and rich library support makes it the preferred tool in these areas.
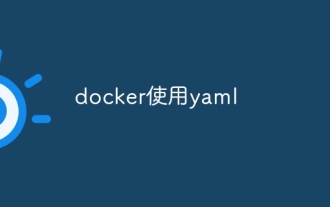
YAML is used to configure containers, images, and services for Docker. To configure: For containers, specify the name, image, port, and environment variables in docker-compose.yml. For images, basic images, build commands, and default commands are provided in Dockerfile. For services, set the name, mirror, port, volume, and environment variables in docker-compose.service.yml.
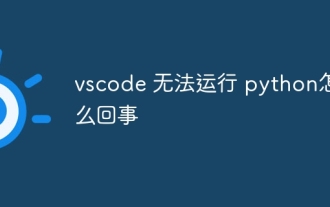
The most common "cannot run Python" problem stems from the misconfiguration of the Python interpreter path. Solutions include: confirming Python installation, configuring VS Code, and using a virtual environment. In addition, there are efficient debugging techniques and best practices such as breakpoint debugging, variable monitoring, log output, and code formatting, such as isolating dependencies using virtual environments, tracking code execution using breakpoints, and tracking variable changes in real time using monitoring expressions, etc., which can greatly improve development efficiency.
