Python: Automation, Scripting, and Task Management
Python excels in automation, scripting, and task management. 1) Automation: implement file backup through standard libraries such as os and shutil. 2) Scripting: Use the psutil library to monitor system resources. 3) Task management: Use the schedule library to schedule tasks. Python's ease of use and rich library support makes it the preferred tool in these areas.
introduction
What do you think of when we talk about Python? Is it its concise syntax or a powerful library ecosystem? Today we are going to explore in-depth the application of Python in automation, scripting and task management. Through this article, you will learn how Python can be the best in these fields and master some practical tips and best practices.
Review of basic knowledge
Python shines in automation and scripting mainly because of its ease of use and rich library support. Let's briefly review the relevant basics:
- Automation : refers to the automatic execution of repetitive tasks through programming to reduce manual intervention.
- Scripting : Write small programs to complete specific tasks, often used for system management or data processing.
- Task management : involves scheduling tasks, monitoring task status and processing task results.
Python's standard libraries such as os
, sys
and subprocess
provide powerful system operation capabilities, while third-party libraries such as schedule
and apscheduler
make task scheduling a breeze.
Core concept or function analysis
Python application in automation
Automation is a major strength of Python. Whether it is file processing, data collection or system management, Python can easily deal with it. Let's look at a simple automation example:
import os import shutil # Automatic file backup def backup_files(source_dir, backup_dir): if not os.path.exists(backup_dir): os.makedirs(backup_dir) for filename in os.listdir(source_dir): source_path = os.path.join(source_dir, filename) backup_path = os.path.join(backup_dir, filename) shutil.copy2(source_path, backup_path) # Use example source_directory = '/path/to/source' backup_directory = '/path/to/backup' backup_files(source_directory, backup_directory)
This simple script shows how Python automates file backups through standard libraries. It works by iterating over files in the source directory and copying them into the backup directory.
Python application in scripting
Scripting is another important application scenario in Python. Let's look at a simple script example for monitoring system resources:
import psutil def monitor_system(): cpu_percent = psutil.cpu_percent(interval=1) memory = psutil.virtual_memory() disk = psutil.disk_usage('/') print(f"CPU Usage: {cpu_percent}%") print(f"Memory Usage: {memory.percent}%") print(f"Disk Usage: {disk.percent}%") if __name__ == "__main__": monitor_system()
This script uses the psutil
library to get CPU, memory, and disk usage. It works by calling psutil
's API to get real-time data of system resources.
Python in task management
Task management is a natural extension of Python in automation and scripting. Let's look at a simple task scheduling example:
import schedule import time def job(): print("I'm working...") schedule.every(10).minutes.do(job) # Execute while True every 10 minutes: schedule.run_pending() time.sleep(1)
This script uses schedule
library to schedule tasks and executes job
functions every 10 minutes. It works by setting the execution frequency of tasks through schedule
library and constantly checking in the main loop whether there are tasks to be executed.
Example of usage
Basic usage
Let's look at a more complex automation example for batch processing of images:
from PIL import Image import os def resize_images(source_dir, target_dir, size): if not os.path.exists(target_dir): os.makedirs(target_dir) for filename in os.listdir(source_dir): if filename.endswith(('.png', '.jpg', '.jpeg')): with Image.open(os.path.join(source_dir, filename)) as img: img = img.resize(size, Image.LANCZOS) img.save(os.path.join(target_dir, filename)) # Use example source_directory = '/path/to/source' target_directory = '/path/to/target' resize_images(source_directory, target_directory, (300, 300))
This script uses the PIL
library to resize images in batches. It iterates over image files in the source directory, resizes them to the specified size, and saves them to the target directory.
Advanced Usage
Let's look at a more complex script example to monitor the availability of a website:
import requests from time import sleep import smtplib from email.mime.text import MIMEText def check_website(url): try: response = requests.get(url) response.raise_for_status() return True except requests.RequestException: return False def send_alert(email, subject, body): msg = MIMEText(body) msg['Subject'] = subject msg['From'] = 'alert@example.com' msg['To'] = email with smtplib.SMTP('smtp.example.com', 587) as server: server.starttls() server.login('username', 'password') server.send_message(msg) def monitor_website(url, email): While True: If not check_website(url): send_alert(email, 'Website Down', f'The website {url} is currently down.') sleep(60) # Check once a minute# Use example website_url = 'https://example.com' alert_email = 'user@example.com' monitor_website(website_url, alert_email)
This script uses the requests
library to check the availability of the website and uses the smtplib
library to send alert emails when the website is unavailable. It checks the availability of the website every minute through an infinite loop and sends an alert immediately when a problem is detected.
Common Errors and Debugging Tips
There are some common problems you may encounter when using Python for automation, scripting, and task management:
- Permissions Issue : Make sure your script has sufficient permissions to access and operate the file system.
- Dependency Issue : Make sure that all required libraries are installed correctly, it is recommended to use a virtual environment to manage dependencies.
- Network problem : When processing network requests, pay attention to handling timeouts and connection errors.
Debugging Tips:
- Logging : Use the
logging
module to record the script execution process to help locate problems. - Exception handling : Use
try-except
block to catch and handle possible exceptions to avoid script crashes. - Debugging tools : Use
pdb
or IDE's own debugging tools to execute code step by step and view variable status.
Performance optimization and best practices
In practical applications, how to optimize Python code to improve the efficiency of automation, scripting and task management?
- Using asynchronous programming : For I/O-intensive tasks, using the
asyncio
library can significantly improve performance. For example, when monitoring multiple websites, requests can be sent in parallel:
import asyncio import aiohttp async def check_website(session, url): try: async with session.get(url) as response: response.raise_for_status() return True except aiohttp.ClientError: return False async def monitor_websites(urls): async with aiohttp.ClientSession() as session: tasks = [check_website(session, url) for url in urls] results = await asyncio.gather(*tasks) for url, result in zip(urls, results): If not result: print(f'{url} is down') # Use example urls = ['https://example1.com', 'https://example2.com'] asyncio.run(monitor_websites(urls))
Code readability : Write clear and detailed code to improve the maintainability of the code. For example, add comments to explain complex logic using meaningful variable names and function names.
Modular design : divide the code into multiple modules or functions to improve the reusability and testability of the code. For example, encapsulate different task logic into independent functions for easy testing and maintenance.
Performance testing : Use
timeit
module or other performance testing tools to evaluate the execution efficiency of the code, identify bottlenecks and optimize. For example, compare the performance differences between different algorithm implementations:
import timeit def method1(): result = 0 for i in range(1000000): result = i return result def method2(): Return sum(range(1000000)) print("Method 1:", timeit.timeit(method1, number=10)) print("Method 2:", timeit.timeit(method2, number=10))
With these tips and best practices, you can better leverage Python to enable automation, scripting, and task management, improving productivity and code quality.
In practical applications, I have encountered a project that requires regular collection of data from multiple data sources and processing it. Due to the large amount of data and the high acquisition frequency, I used asynchronous programming to process data acquisition tasks in parallel, which greatly improved efficiency. At the same time, I also used logging and exception handling to ensure the stability and maintainability of the system.
Hopefully this article will provide you with some useful insights and practical experience to help you achieve greater success in Python automation, scripting and task management.
The above is the detailed content of Python: Automation, Scripting, and Task Management. For more information, please follow other related articles on the PHP Chinese website!

Hot AI Tools

Undresser.AI Undress
AI-powered app for creating realistic nude photos

AI Clothes Remover
Online AI tool for removing clothes from photos.

Undress AI Tool
Undress images for free

Clothoff.io
AI clothes remover

AI Hentai Generator
Generate AI Hentai for free.

Hot Article

Hot Tools

Notepad++7.3.1
Easy-to-use and free code editor

SublimeText3 Chinese version
Chinese version, very easy to use

Zend Studio 13.0.1
Powerful PHP integrated development environment

Dreamweaver CS6
Visual web development tools

SublimeText3 Mac version
God-level code editing software (SublimeText3)

Hot Topics



HTMLisnotaprogramminglanguage;itisamarkuplanguage.1)HTMLstructuresandformatswebcontentusingtags.2)ItworkswithCSSforstylingandJavaScriptforinteractivity,enhancingwebdevelopment.
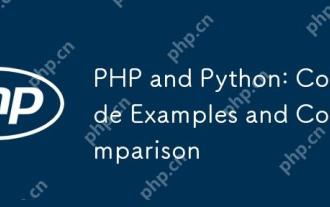
PHP and Python have their own advantages and disadvantages, and the choice depends on project needs and personal preferences. 1.PHP is suitable for rapid development and maintenance of large-scale web applications. 2. Python dominates the field of data science and machine learning.
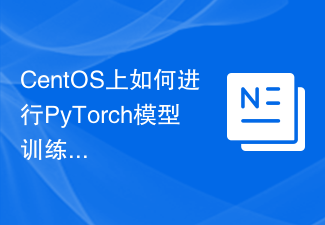
Efficient training of PyTorch models on CentOS systems requires steps, and this article will provide detailed guides. 1. Environment preparation: Python and dependency installation: CentOS system usually preinstalls Python, but the version may be older. It is recommended to use yum or dnf to install Python 3 and upgrade pip: sudoyumupdatepython3 (or sudodnfupdatepython3), pip3install--upgradepip. CUDA and cuDNN (GPU acceleration): If you use NVIDIAGPU, you need to install CUDATool
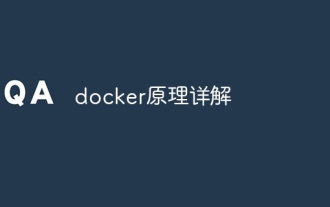
Docker uses Linux kernel features to provide an efficient and isolated application running environment. Its working principle is as follows: 1. The mirror is used as a read-only template, which contains everything you need to run the application; 2. The Union File System (UnionFS) stacks multiple file systems, only storing the differences, saving space and speeding up; 3. The daemon manages the mirrors and containers, and the client uses them for interaction; 4. Namespaces and cgroups implement container isolation and resource limitations; 5. Multiple network modes support container interconnection. Only by understanding these core concepts can you better utilize Docker.
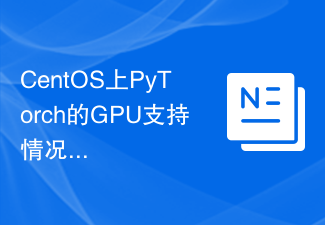
Enable PyTorch GPU acceleration on CentOS system requires the installation of CUDA, cuDNN and GPU versions of PyTorch. The following steps will guide you through the process: CUDA and cuDNN installation determine CUDA version compatibility: Use the nvidia-smi command to view the CUDA version supported by your NVIDIA graphics card. For example, your MX450 graphics card may support CUDA11.1 or higher. Download and install CUDAToolkit: Visit the official website of NVIDIACUDAToolkit and download and install the corresponding version according to the highest CUDA version supported by your graphics card. Install cuDNN library:
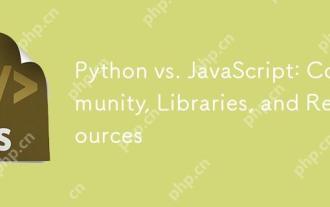
Python and JavaScript have their own advantages and disadvantages in terms of community, libraries and resources. 1) The Python community is friendly and suitable for beginners, but the front-end development resources are not as rich as JavaScript. 2) Python is powerful in data science and machine learning libraries, while JavaScript is better in front-end development libraries and frameworks. 3) Both have rich learning resources, but Python is suitable for starting with official documents, while JavaScript is better with MDNWebDocs. The choice should be based on project needs and personal interests.
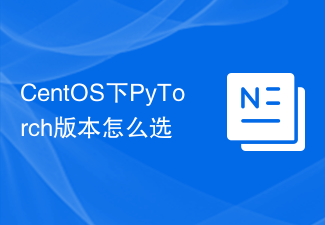
When selecting a PyTorch version under CentOS, the following key factors need to be considered: 1. CUDA version compatibility GPU support: If you have NVIDIA GPU and want to utilize GPU acceleration, you need to choose PyTorch that supports the corresponding CUDA version. You can view the CUDA version supported by running the nvidia-smi command. CPU version: If you don't have a GPU or don't want to use a GPU, you can choose a CPU version of PyTorch. 2. Python version PyTorch
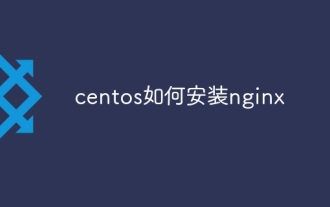
CentOS Installing Nginx requires following the following steps: Installing dependencies such as development tools, pcre-devel, and openssl-devel. Download the Nginx source code package, unzip it and compile and install it, and specify the installation path as /usr/local/nginx. Create Nginx users and user groups and set permissions. Modify the configuration file nginx.conf, and configure the listening port and domain name/IP address. Start the Nginx service. Common errors need to be paid attention to, such as dependency issues, port conflicts, and configuration file errors. Performance optimization needs to be adjusted according to the specific situation, such as turning on cache and adjusting the number of worker processes.
