This Research Paper Won the ICML 2024 Best Paper Award
A Groundbreaking Paper on Dataset Diversity in Machine Learning
The machine learning (ML) community is abuzz over a recent ICML 2024 Best Paper Award winner that challenges the often-unsubstantiated claims of "diversity" in datasets. Researchers Dora Zhao, Jerone T. A. Andrews, Orestis Papakyriakopoulos, and Alice Xiang's work, "Measure Dataset Diversity, Don't Just Claim It," provides a much-needed framework for rigorously assessing dataset diversity.
This isn't just another paper on dataset diversity; it's a call to action. The authors critique the loose use of terms like "diversity," "quality," and "bias" without proper validation. Their solution? A structured approach using measurement theory principles to define, measure, and evaluate diversity in ML datasets.
The paper's framework involves three crucial steps:
- Conceptualization: Defining "diversity" within the specific context of the dataset.
- Operationalization: Developing concrete methods to quantify the defined aspects of diversity.
- Evaluation: Assessing the reliability and validity of the diversity measurements.
Key findings from their analysis of 135 image and text datasets reveal significant shortcomings: a lack of clear definitions of diversity, insufficient documentation of data collection, reliability concerns, and challenges in validating diversity claims. The researchers offer practical recommendations to address these issues, including using inter-annotator agreement and employing techniques from construct validity.
A case study of the Segment Anything dataset (SA-1B) highlights the framework's practical application, identifying both strengths and areas for improvement in its diversity considerations.
The implications are far-reaching: the paper challenges the assumption that larger datasets automatically equate to greater diversity, emphasizing the need for intentional curation. It also acknowledges the increased documentation burden but advocates for systemic changes in how data work is valued within the ML research community. Furthermore, it highlights the importance of considering how diversity constructs evolve over time.
Read the full paper: Position: Measure Dataset Diversity, Don't Just Claim It
The conclusion emphasizes the need for more rigorous, transparent, and reproducible research in ML. The authors' framework provides essential tools for ensuring that claims of dataset diversity are not merely rhetoric but demonstrably meaningful contributions to fairer and more robust AI systems. This work serves as a critical step toward improving dataset curation and documentation, ultimately leading to more reliable and equitable machine learning models.
While the increased rigor may seem demanding, the authors convincingly argue that building AI on shaky foundations is unacceptable. This paper isn't just about better datasets; it's about a more trustworthy and accountable field of machine learning.
Frequently Asked Questions:
- Q1: Why is measuring dataset diversity important? A1: It ensures diverse representation, reduces bias, improves model generalizability, and promotes fairness in AI.
- Q2: How does dataset diversity impact ML model performance? A2: It improves robustness and accuracy by reducing overfitting and enhancing performance across different populations and conditions.
- Q3: What are common challenges in measuring dataset diversity? A3: Defining diversity, operationalizing definitions, validating claims, and ensuring transparent and reproducible documentation.
- Q4: What are practical steps for improving dataset diversity? A4: Clearly defining diversity goals, collecting data from diverse sources, using standardized measurement methods, continuous evaluation, and implementing robust validation.
The above is the detailed content of This Research Paper Won the ICML 2024 Best Paper Award. For more information, please follow other related articles on the PHP Chinese website!

Hot AI Tools

Undresser.AI Undress
AI-powered app for creating realistic nude photos

AI Clothes Remover
Online AI tool for removing clothes from photos.

Undress AI Tool
Undress images for free

Clothoff.io
AI clothes remover

Video Face Swap
Swap faces in any video effortlessly with our completely free AI face swap tool!

Hot Article

Hot Tools

Notepad++7.3.1
Easy-to-use and free code editor

SublimeText3 Chinese version
Chinese version, very easy to use

Zend Studio 13.0.1
Powerful PHP integrated development environment

Dreamweaver CS6
Visual web development tools

SublimeText3 Mac version
God-level code editing software (SublimeText3)

Hot Topics
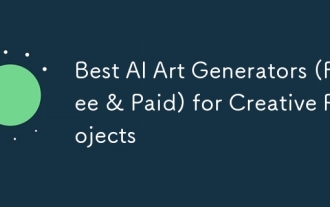
The article reviews top AI art generators, discussing their features, suitability for creative projects, and value. It highlights Midjourney as the best value for professionals and recommends DALL-E 2 for high-quality, customizable art.
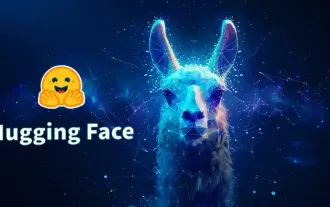
Meta's Llama 3.2: A Leap Forward in Multimodal and Mobile AI Meta recently unveiled Llama 3.2, a significant advancement in AI featuring powerful vision capabilities and lightweight text models optimized for mobile devices. Building on the success o
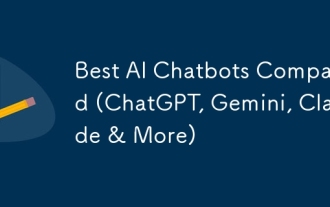
The article compares top AI chatbots like ChatGPT, Gemini, and Claude, focusing on their unique features, customization options, and performance in natural language processing and reliability.
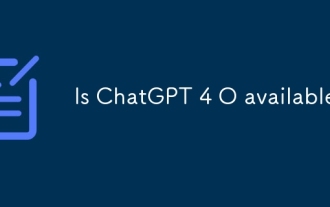
ChatGPT 4 is currently available and widely used, demonstrating significant improvements in understanding context and generating coherent responses compared to its predecessors like ChatGPT 3.5. Future developments may include more personalized interactions and real-time data processing capabilities, further enhancing its potential for various applications.
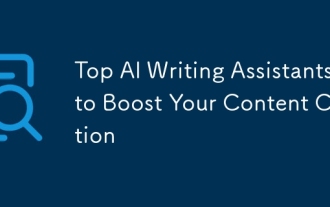
The article discusses top AI writing assistants like Grammarly, Jasper, Copy.ai, Writesonic, and Rytr, focusing on their unique features for content creation. It argues that Jasper excels in SEO optimization, while AI tools help maintain tone consist
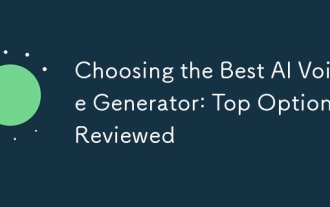
The article reviews top AI voice generators like Google Cloud, Amazon Polly, Microsoft Azure, IBM Watson, and Descript, focusing on their features, voice quality, and suitability for different needs.
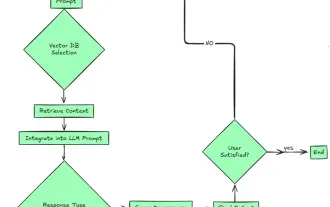
2024 witnessed a shift from simply using LLMs for content generation to understanding their inner workings. This exploration led to the discovery of AI Agents – autonomous systems handling tasks and decisions with minimal human intervention. Buildin
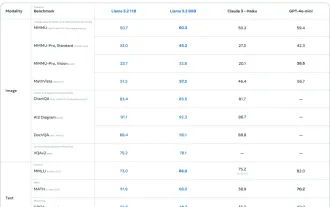
This week's AI landscape: A whirlwind of advancements, ethical considerations, and regulatory debates. Major players like OpenAI, Google, Meta, and Microsoft have unleashed a torrent of updates, from groundbreaking new models to crucial shifts in le
