What is Data Scrubbing?
Data Cleansing: Ensuring Data Accuracy and Reliability for Informed Decisions
Imagine planning a large family reunion with an inaccurate guest list—wrong contacts, duplicates, misspelled names. A poorly prepared list could ruin the event. Similarly, businesses rely on clean, accurate data for effective operations and strategic decision-making. The process of cleaning and correcting data—ensuring accuracy, removing duplicates, and updating information—is known as data scrubbing or data cleansing. Just as meticulous planning ensures a successful reunion, data scrubbing improves business performance and decision-making.
Key Aspects of Data Cleansing:
- Understanding the critical role of data cleansing.
- Exploring effective data cleansing techniques and tools.
- Identifying common data quality problems and their solutions.
- Implementing data cleansing strategies within your organization.
- Addressing and mitigating potential challenges in the data cleansing process.
Table of Contents:
- Introduction
- What is Data Cleansing?
- The Data Cleansing Process: A Step-by-Step Guide
- Techniques and Tools for Data Cleansing
- The Importance of Data Cleansing
- Addressing Common Data Quality Issues
- Best Practices for Data Cleansing
- Challenges in Data Cleansing
- Conclusion
- Frequently Asked Questions
What is Data Cleansing?
Data cleansing is a crucial data management process that identifies and rectifies data errors, inconsistencies, and inaccuracies. These issues can arise from various sources, including incorrect data entry, database problems, and merging data from multiple sources. Clean data is essential for accurate analysis, reporting, and effective decision-making.
The Data Cleansing Process: A Step-by-Step Guide
Data cleansing is an iterative process involving several key steps:
- Data Validation: Verifying data accuracy and consistency against predefined rules and formats (e.g., ensuring dates are in YYYY-MM-DD format).
- Duplicate Detection and Removal: Identifying and eliminating duplicate entries resulting from data entry errors or system issues.
- Data Standardization: Converting data into a consistent format across different sources (e.g., standardizing currency or date formats).
- Data Correction: Rectifying errors such as typos, incorrect entries, and outdated information.
- Data Enrichment: Supplementing existing data with missing information from external sources or updating records with current details.
- Data Transformation: Converting data into a format suitable for analysis and reporting (e.g., aggregating data or creating calculated fields).
- Data Integration: Combining data from multiple sources into a unified and consistent format.
- Data Auditing: Regularly reviewing data quality and the effectiveness of the cleansing process to ensure ongoing data integrity.
Techniques and Tools for Data Cleansing
Effective data cleansing relies on a combination of techniques and tools:
Techniques:
- Data Validation: Verifying data against predefined rules.
- Data Parsing: Breaking down data into smaller units for error detection.
- Data Standardization: Ensuring consistent data formats.
- Duplicate Removal: Identifying and removing duplicate records.
- Error Correction: Manually or automatically fixing identified errors.
- Data Enrichment: Adding missing or enhancing existing data.
Tools:
- OpenRefine: A powerful open-source tool for data cleaning and transformation.
- Trifacta: An AI-powered data preparation platform.
- Talend: An ETL (Extract, Transform, Load) tool with data cleansing capabilities.
- Data Ladder: A data matching and deduplication tool.
- Pandas (Python Library): A versatile Python library for data manipulation and cleaning.
The Importance of Data Cleansing
Data cleansing offers numerous benefits:
- Improved Decision-Making: Accurate data leads to better informed and more effective decisions.
- Increased Efficiency: Clean data streamlines processes, reducing time spent on error correction.
- Enhanced Customer Relations: Accurate customer data improves customer service and loyalty.
- Regulatory Compliance: Ensures adherence to data privacy and accuracy regulations.
- Cost Savings: Prevents wasted resources due to inaccurate or incomplete data.
- Better Data Integration: Facilitates seamless integration of data from various sources.
- More Accurate Analytics and Reporting: Clean data ensures reliable insights from analytics and reporting.
Addressing Common Data Quality Issues
Common data quality issues and their solutions:
- Missing Values: Imputation (estimating missing values) or removal of incomplete records.
- Inconsistent Data Formats: Standardization of formats (dates, addresses, etc.).
- Duplicate Records: Algorithms to identify and merge or remove duplicates.
- Outliers: Investigation to determine if they are errors or valid data points.
- Incorrect Data: Validation against trusted sources or automated correction.
Best Practices for Data Cleansing
- Establish Data Quality Standards: Define clear criteria for data accuracy and consistency.
- Automate Where Possible: Utilize data cleaning tools and scripts to automate the process.
- Regularly Review and Update Data: Data cleansing is an ongoing process.
- Involve Data Owners: Collaborate with individuals familiar with the data.
- Document Your Process: Maintain detailed records of cleansing activities and decisions.
Challenges in Data Cleansing
- Large Data Volumes: Processing massive datasets can be computationally intensive.
- Data Complexity: Handling various data types and structures.
- Lack of Standardization: Inconsistent data standards across different sources.
- Resource Intensity: Requires significant human and technical resources.
- Continuous Process: Maintaining data quality requires ongoing effort.
Conclusion
Data cleansing is critical for ensuring data accuracy and reliability, leading to better decision-making and improved business outcomes. While challenges exist, the benefits of implementing effective data cleansing strategies far outweigh the effort involved. Investing in data cleansing is an investment in the quality and value of your data.
Frequently Asked Questions
Q1. What is data cleansing? A. Data cleansing is the process of identifying and correcting or removing inaccurate, incomplete, irrelevant, duplicated, or improperly formatted data.
Q2. Why is data cleansing important? A. Data cleansing ensures data accuracy, consistency, and reliability, crucial for informed decision-making, efficient operations, and regulatory compliance.
Q3. What are some common data quality issues? A. Common issues include missing values, inconsistent formats, duplicates, outliers, and incorrect data.
Q4. What tools can be used for data cleansing? A. Tools like OpenRefine, Trifacta, Talend, and Pandas are commonly used.
Q5. What are the challenges in data cleansing? A. Challenges include data volume, complexity, lack of standardization, resource requirements, and the ongoing nature of the process.
The above is the detailed content of What is Data Scrubbing?. For more information, please follow other related articles on the PHP Chinese website!

Hot AI Tools

Undresser.AI Undress
AI-powered app for creating realistic nude photos

AI Clothes Remover
Online AI tool for removing clothes from photos.

Undress AI Tool
Undress images for free

Clothoff.io
AI clothes remover

Video Face Swap
Swap faces in any video effortlessly with our completely free AI face swap tool!

Hot Article

Hot Tools

Notepad++7.3.1
Easy-to-use and free code editor

SublimeText3 Chinese version
Chinese version, very easy to use

Zend Studio 13.0.1
Powerful PHP integrated development environment

Dreamweaver CS6
Visual web development tools

SublimeText3 Mac version
God-level code editing software (SublimeText3)

Hot Topics










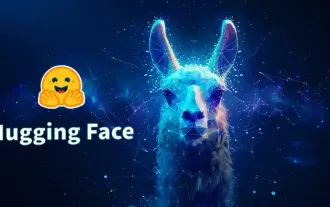
Meta's Llama 3.2: A Leap Forward in Multimodal and Mobile AI Meta recently unveiled Llama 3.2, a significant advancement in AI featuring powerful vision capabilities and lightweight text models optimized for mobile devices. Building on the success o
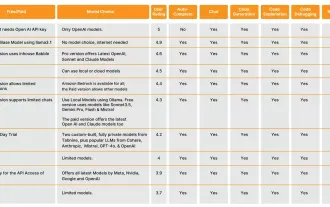
Hey there, Coding ninja! What coding-related tasks do you have planned for the day? Before you dive further into this blog, I want you to think about all your coding-related woes—better list those down. Done? – Let’
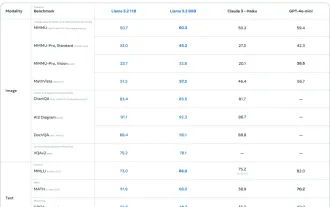
This week's AI landscape: A whirlwind of advancements, ethical considerations, and regulatory debates. Major players like OpenAI, Google, Meta, and Microsoft have unleashed a torrent of updates, from groundbreaking new models to crucial shifts in le
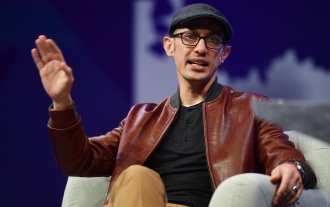
Shopify CEO Tobi Lütke's recent memo boldly declares AI proficiency a fundamental expectation for every employee, marking a significant cultural shift within the company. This isn't a fleeting trend; it's a new operational paradigm integrated into p
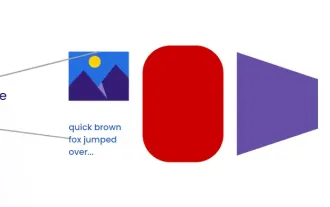
Introduction Imagine walking through an art gallery, surrounded by vivid paintings and sculptures. Now, what if you could ask each piece a question and get a meaningful answer? You might ask, “What story are you telling?
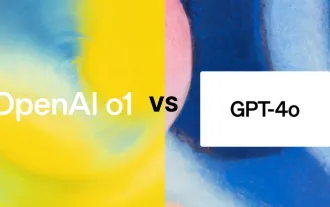
Introduction OpenAI has released its new model based on the much-anticipated “strawberry” architecture. This innovative model, known as o1, enhances reasoning capabilities, allowing it to think through problems mor
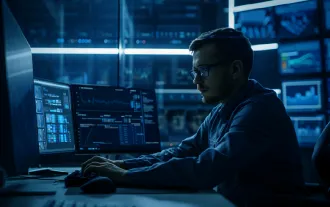
SQL's ALTER TABLE Statement: Dynamically Adding Columns to Your Database In data management, SQL's adaptability is crucial. Need to adjust your database structure on the fly? The ALTER TABLE statement is your solution. This guide details adding colu
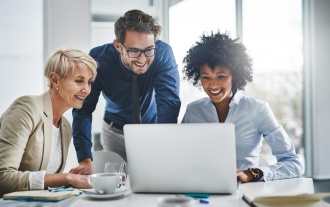
For those of you who might be new to my column, I broadly explore the latest advances in AI across the board, including topics such as embodied AI, AI reasoning, high-tech breakthroughs in AI, prompt engineering, training of AI, fielding of AI, AI re
