


7 Ways to Split Data Using LangChain Text Splitters - Analytics Vidhya
LangChain Text Splitters: Optimizing LLM Input for Efficiency and Accuracy
Our previous article covered LangChain's document loaders. However, LLMs have context window size limitations (measured in tokens). Exceeding this limit truncates data, compromising accuracy and increasing costs. The solution? Send only relevant data to the LLM, requiring data splitting. Enter LangChain's Text Splitters.
Key Concepts:
- The Crucial Role of Text Splitters: Understand why efficient text splitting is vital for optimizing LLM applications, balancing context window size and cost.
- Diverse Text Splitting Techniques: Explore various methods, including character counts, token counts, recursive splitting, and techniques tailored to HTML, code, and JSON structures.
- LangChain Text Splitter Implementation: Learn practical application, including installation, code examples for text splitting, and handling diverse data formats.
- Semantic Splitting for Enhanced Relevance: Discover how sentence embeddings and cosine similarity create semantically coherent chunks, maximizing relevance.
Table of Contents:
- What are Text Splitters?
- Data Splitting Methods
- Character Count-Based Splitting
- Recursive Splitting
- Token Count-Based Splitting
- Handling HTML
- Code-Specific Splitting
- JSON Data Handling
- Semantic Chunking
- Frequently Asked Questions
What are Text Splitters?
Text splitters divide large text into smaller, manageable chunks for improved LLM query relevance. They work directly on raw text or LangChain document objects. Multiple methods cater to different content types and use cases.
Data Splitting Methods
LangChain Text Splitters are crucial for efficient large document processing. They improve performance, contextual understanding, enable parallel processing, and facilitate better data management. Let's examine several methods:
Prerequisites: Install the package using pip install langchain_text_splitters
Character Count-Based Splitting
This method splits text based on character count, using a specified separator.
from langchain_community.document_loaders import UnstructuredPDFLoader from langchain_text_splitters import CharacterTextSplitter # Load data (replace with your PDF path) loader = UnstructuredPDFLoader('how-to-formulate-successful-business-strategy.pdf', mode='single') data = loader.load() text_splitter = CharacterTextSplitter(separator="\n", chunk_size=500, chunk_overlap=0, is_separator_regex=False) texts = text_splitter.split_documents(data) len(texts) # Output: Number of chunks
This example splits text into 500-character chunks, using newline characters as separators.
Recursive Splitting
This uses multiple separators sequentially until chunks are below chunk_size
. Useful for sentence-level splitting.
from langchain_text_splitters import RecursiveCharacterTextSplitter recursive_splitter = RecursiveCharacterTextSplitter(separators=["\n\n", "\n", r"(?>> 293 # ... (rest of the code remains similar)
Token Count-Based Splitting
LLMs use tokens; splitting by token count is more accurate. This example uses the o200k_base
encoding (check the GitHub link for model/encoding mappings).
from langchain_text_splitters import TokenTextSplitter text_splitter = TokenTextSplitter(encoding_name='o200k_base', chunk_size=50, chunk_overlap=0) texts = text_splitter.split_documents(data) len(texts) # Output: Number of chunks
Recursive splitting can also be combined with token counting.
For plain text, recursive splitting with character or token counting is generally preferred.
Handling HTML
For structured data like HTML, splitting should respect the structure. This example splits based on HTML headers.
from langchain_text_splitters import HTMLHeaderTextSplitter headers_to_split_on = [("h1", "Header 1"), ("h2", "Header 2"), ("h3", "Header 3")] html_splitter = HTMLHeaderTextSplitter(headers_to_split_on, return_each_element=True) html_header_splits = html_splitter.split_text_from_url('https://diataxis.fr/') len(html_header_splits) # Output: Number of chunks
HTMLSectionSplitter
allows splitting based on other sections.
Code-Specific Splitting
Programming languages have unique structures. This example uses syntax-aware splitting for Python code.
from langchain_text_splitters import RecursiveCharacterTextSplitter, Language # ... (Python code example) ... python_splitter = RecursiveCharacterTextSplitter.from_language(language=Language.PYTHON, chunk_size=100, chunk_overlap=0) python_docs = python_splitter.create_documents([PYTHON_CODE])
JSON Data Handling
Nested JSON objects can be split while preserving key relationships.
from langchain_text_splitters import RecursiveJsonSplitter # ... (JSON data example) ... splitter = RecursiveJsonSplitter(max_chunk_size=200, min_chunk_size=20) chunks = splitter.split_text(json_data, convert_lists=True)
Semantic Chunking
This method uses sentence embeddings and cosine similarity to group semantically related sentences.
from langchain_experimental.text_splitter import SemanticChunker from langchain_openai.embeddings import OpenAIEmbeddings # Requires OpenAI API key # ... (code using OpenAIEmbeddings and SemanticChunker) ...
Conclusion
LangChain offers various text splitting methods, each suited for different data types. Choosing the right method optimizes LLM input, improving accuracy and reducing costs.
Frequently Asked Questions
(Q&A section remains largely the same, with minor wording adjustments for clarity and flow.)
The above is the detailed content of 7 Ways to Split Data Using LangChain Text Splitters - Analytics Vidhya. For more information, please follow other related articles on the PHP Chinese website!

Hot AI Tools

Undresser.AI Undress
AI-powered app for creating realistic nude photos

AI Clothes Remover
Online AI tool for removing clothes from photos.

Undress AI Tool
Undress images for free

Clothoff.io
AI clothes remover

Video Face Swap
Swap faces in any video effortlessly with our completely free AI face swap tool!

Hot Article

Hot Tools

Notepad++7.3.1
Easy-to-use and free code editor

SublimeText3 Chinese version
Chinese version, very easy to use

Zend Studio 13.0.1
Powerful PHP integrated development environment

Dreamweaver CS6
Visual web development tools

SublimeText3 Mac version
God-level code editing software (SublimeText3)

Hot Topics
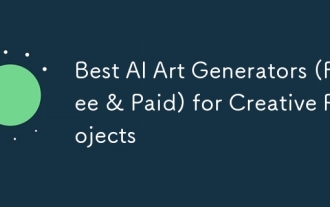
The article reviews top AI art generators, discussing their features, suitability for creative projects, and value. It highlights Midjourney as the best value for professionals and recommends DALL-E 2 for high-quality, customizable art.
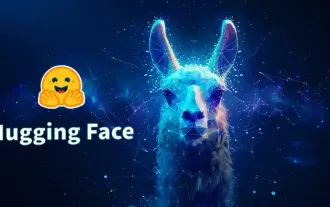
Meta's Llama 3.2: A Leap Forward in Multimodal and Mobile AI Meta recently unveiled Llama 3.2, a significant advancement in AI featuring powerful vision capabilities and lightweight text models optimized for mobile devices. Building on the success o
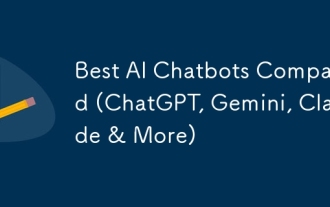
The article compares top AI chatbots like ChatGPT, Gemini, and Claude, focusing on their unique features, customization options, and performance in natural language processing and reliability.
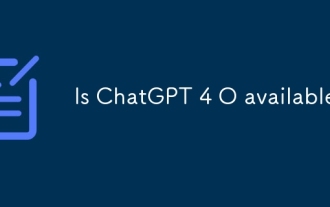
ChatGPT 4 is currently available and widely used, demonstrating significant improvements in understanding context and generating coherent responses compared to its predecessors like ChatGPT 3.5. Future developments may include more personalized interactions and real-time data processing capabilities, further enhancing its potential for various applications.
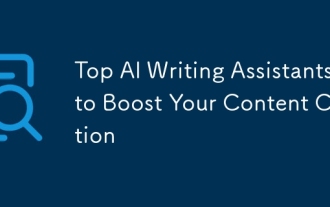
The article discusses top AI writing assistants like Grammarly, Jasper, Copy.ai, Writesonic, and Rytr, focusing on their unique features for content creation. It argues that Jasper excels in SEO optimization, while AI tools help maintain tone consist
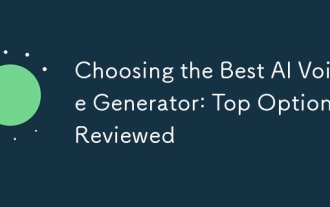
The article reviews top AI voice generators like Google Cloud, Amazon Polly, Microsoft Azure, IBM Watson, and Descript, focusing on their features, voice quality, and suitability for different needs.
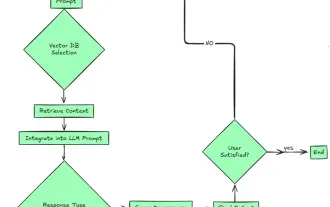
2024 witnessed a shift from simply using LLMs for content generation to understanding their inner workings. This exploration led to the discovery of AI Agents – autonomous systems handling tasks and decisions with minimal human intervention. Buildin
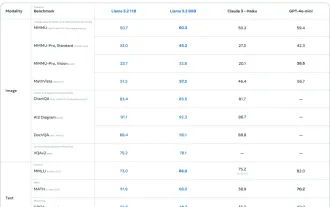
This week's AI landscape: A whirlwind of advancements, ethical considerations, and regulatory debates. Major players like OpenAI, Google, Meta, and Microsoft have unleashed a torrent of updates, from groundbreaking new models to crucial shifts in le
