R-CNN vs R-CNN Fast vs R-CNN Faster vs YOLO - Analytics Vidhya
Object Detection: From R-CNN to YOLO – A Journey Through Computer Vision
Imagine a computer not just seeing, but understanding images. This is the essence of object detection, a pivotal area in computer vision revolutionizing machine-world interaction. From self-driving cars navigating busy streets to security systems identifying threats, object detection quietly ensures smooth, accurate operation.
But how does a computer transform pixels into identified objects? This article explores the evolution of object detection algorithms, charting the progress from R-CNN to YOLO, highlighting the crucial speed-accuracy trade-offs that have pushed machine vision beyond human capabilities in some areas.
Key Areas Covered:
- Introduction to object detection and its significance in computer vision.
- The evolution of object detection algorithms: R-CNN to YOLO.
- Detailed explanation of R-CNN, Fast R-CNN, Faster R-CNN, and YOLO: their mechanisms, strengths, and weaknesses.
- Real-world applications of each algorithm.
Table of Contents:
- The R-CNN Family: A History of Innovation
- R-CNN: The Groundbreaker
- Fast R-CNN: Speed and Accuracy Combined
- Faster R-CNN: Rapid Region Proposals
- YOLO: A Single Glance
- Algorithm Comparison: Evolution of Object Detection
- The Future of Object Detection: Pushing Boundaries
- Your Turn to Detect
- Frequently Asked Questions
The R-CNN Family: A History of Innovation
R-CNN (Regions with CNN features): The Pioneer
Introduced in 2014, R-CNN revolutionized object detection. Its process:
- Generates region proposals (around 2000) using selective search.
- Extracts CNN features from each region.
- Classifies regions using SVM classifiers.
Advantages | Limitations |
---|---|
Significantly higher accuracy than prior methods | Extremely slow (47 seconds per image) |
Utilized the power of CNNs for feature extraction | Multi-stage pipeline, hindering end-to-end training |
Real-world application: Imagine using R-CNN to identify fruits in a bowl. It would propose numerous regions, analyze each individually, and pinpoint the location of each apple and orange.
Fast R-CNN: Speed and Accuracy Combined
Fast R-CNN addressed R-CNN's speed issues without sacrificing accuracy:
- Processes the entire image through a CNN once.
- Uses RoI pooling to extract features for each region proposal.
- Employs a softmax layer for classification and bounding box regression.
Advantages | Limitations |
---|---|
Substantially faster than R-CNN (2 seconds per image) | Relies on external region proposals, a bottleneck |
Single-stage training | |
Improved detection accuracy |
Real-world application: In retail, Fast R-CNN rapidly identifies and locates products on shelves, streamlining inventory management.
Faster R-CNN: Rapid Region Proposals
Faster R-CNN introduced the Region Proposal Network (RPN), enabling end-to-end training:
- Uses a fully convolutional network to generate region proposals.
- Shares full-image convolutional features with the detection network.
- Trains the RPN and Fast R-CNN concurrently.
Advantages | Limitations |
---|---|
Near real-time performance (5 fps) | Not fast enough for real-time applications on all hardware |
Higher accuracy due to improved region proposals | |
Fully end-to-end trainable |
Real-world application: In autonomous driving, Faster R-CNN detects and classifies vehicles, pedestrians, and road signs in near real-time, vital for quick decision-making.
YOLO: A Single Glance
YOLO (You Only Look Once) revolutionized object detection by treating it as a single regression problem:
- Divides the image into a grid.
- Predicts bounding boxes and class probabilities for each grid cell.
- Performs a single forward pass on the entire image.
Advantages | Limitations |
---|---|
Extremely fast (45155 fps) | Struggles with small objects or unusual aspect ratios |
Processes streaming video in real-time | |
Learns generalizable object representations |
Real-world application: YOLO excels in sports analytics, tracking multiple players and the ball in real-time for immediate game analysis.
Algorithm Comparison: Evolution of Object Detection
The Future of Object Detection: Pushing Boundaries
The journey from R-CNN to YOLO showcases remarkable progress. However, research continues, focusing on:
- Anchor-free detectors for simplified detection.
- Attention mechanisms for enhanced feature extraction.
- 3D object detection for applications like autonomous driving.
- Lightweight models for edge devices and IoT applications.
Your Turn to Detect
Object detection is no longer confined to research labs. Its accessibility empowers developers, students, and enthusiasts to create innovative applications.
Frequently Asked Questions
Q1. What is object detection? A: Object detection identifies and categorizes visual objects within images or videos.
Q2. How does R-CNN work? A: R-CNN uses region proposals, CNN feature extraction, and SVM classification.
Q3. What is the key improvement in Fast R-CNN? A: Fast R-CNN processes the entire image once using RoI pooling, significantly increasing speed while maintaining accuracy.
Q4. How does Faster R-CNN differ? A: Faster R-CNN introduces the RPN, enabling end-to-end training and near real-time performance.
Q5. What makes YOLO unique? A: YOLO treats object detection as a single regression problem, achieving extreme speed through a single forward pass.
The above is the detailed content of R-CNN vs R-CNN Fast vs R-CNN Faster vs YOLO - Analytics Vidhya. For more information, please follow other related articles on the PHP Chinese website!

Hot AI Tools

Undresser.AI Undress
AI-powered app for creating realistic nude photos

AI Clothes Remover
Online AI tool for removing clothes from photos.

Undress AI Tool
Undress images for free

Clothoff.io
AI clothes remover

Video Face Swap
Swap faces in any video effortlessly with our completely free AI face swap tool!

Hot Article

Hot Tools

Notepad++7.3.1
Easy-to-use and free code editor

SublimeText3 Chinese version
Chinese version, very easy to use

Zend Studio 13.0.1
Powerful PHP integrated development environment

Dreamweaver CS6
Visual web development tools

SublimeText3 Mac version
God-level code editing software (SublimeText3)

Hot Topics
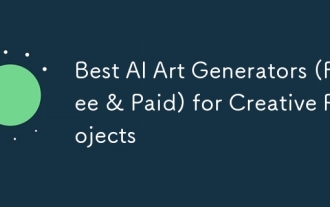
The article reviews top AI art generators, discussing their features, suitability for creative projects, and value. It highlights Midjourney as the best value for professionals and recommends DALL-E 2 for high-quality, customizable art.
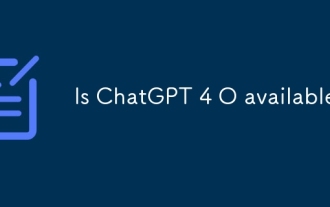
ChatGPT 4 is currently available and widely used, demonstrating significant improvements in understanding context and generating coherent responses compared to its predecessors like ChatGPT 3.5. Future developments may include more personalized interactions and real-time data processing capabilities, further enhancing its potential for various applications.
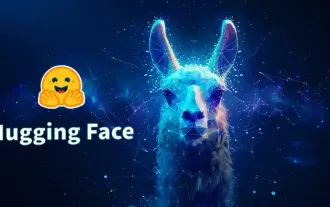
Meta's Llama 3.2: A Leap Forward in Multimodal and Mobile AI Meta recently unveiled Llama 3.2, a significant advancement in AI featuring powerful vision capabilities and lightweight text models optimized for mobile devices. Building on the success o
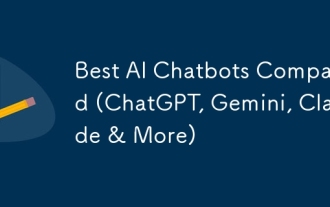
The article compares top AI chatbots like ChatGPT, Gemini, and Claude, focusing on their unique features, customization options, and performance in natural language processing and reliability.
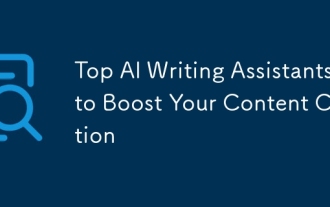
The article discusses top AI writing assistants like Grammarly, Jasper, Copy.ai, Writesonic, and Rytr, focusing on their unique features for content creation. It argues that Jasper excels in SEO optimization, while AI tools help maintain tone consist
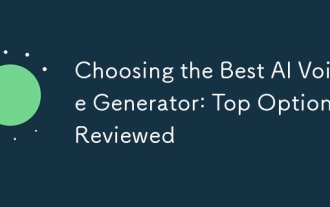
The article reviews top AI voice generators like Google Cloud, Amazon Polly, Microsoft Azure, IBM Watson, and Descript, focusing on their features, voice quality, and suitability for different needs.
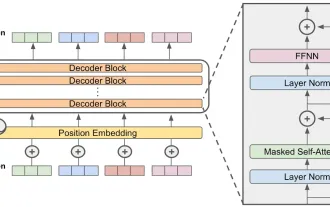
Falcon 3: A Revolutionary Open-Source Large Language Model Falcon 3, the latest iteration in the acclaimed Falcon series of LLMs, represents a significant advancement in AI technology. Developed by the Technology Innovation Institute (TII), this open
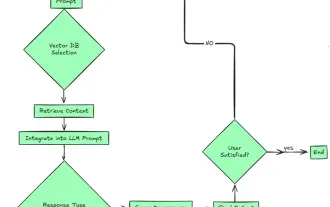
2024 witnessed a shift from simply using LLMs for content generation to understanding their inner workings. This exploration led to the discovery of AI Agents – autonomous systems handling tasks and decisions with minimal human intervention. Buildin
