Graph RAG: Enhancing RAG with Graph Structures - Analytics Vidhya
Introduction
Ever wondered how some AI systems seem to effortlessly access and integrate relevant information into their responses, mimicking a conversation with an expert? This is the power of Retrieval-Augmented Generation (RAG). RAG significantly enhances natural language processing by combining the strengths of generative and retrieval models. Upon receiving a query, a RAG system efficiently retrieves pertinent information from a knowledge base and seamlessly incorporates this data into its response, resulting in more accurate and comprehensive answers.
Overview
This article introduces Graph RAG, an advanced evolution of standard RAG. We'll explore the architecture and functionality of both standard RAG and Graph RAG, highlighting Graph RAG's key advantages. We'll delve into its potential applications across various sectors and research areas, and address the challenges and future directions of this innovative technology.
Table of contents
- Understanding Standard RAG Architecture
- Introducing Graph RAG
- Graph RAG's Superiority over Standard RAG
- The Graph RAG Process
- Visualizing Graph RAG: A Flowchart
- Key Differences: Standard RAG vs. Graph RAG
- Applications and Challenges of Graph RAG
- Frequently Asked Questions
Understanding Standard RAG Architecture
A standard RAG system comprises three core components:
- Retrieval Module: This module searches a knowledge base (or extensive document corpus) for relevant information using techniques like similarity search algorithms and dense vector representations of text.
- Generation Module: A large language model (LLM) generates the response, using both the retrieved information and the original query as input.
- Knowledge Base: The database containing the information the retrieval module accesses.
Building a RAG system begins with creating a knowledge base by indexing documents and generating embeddings. This is followed by training or fine-tuning a retriever model for efficient knowledge base searches and implementing a suitable generator model (often a pre-trained LLM). Finally, these components are integrated for seamless operation.
Also Read: Addressing 12 Common RAG Challenges
Introducing Graph RAG
Graph RAG represents a significant enhancement to the RAG approach by incorporating graph-structured data. Unlike standard RAG, which treats the knowledge base as a flat collection of documents, Graph RAG models information as a network of interconnected entities and their relationships.
Graph RAG's Superiority over Standard RAG
Graph RAG offers several key advantages:
- Contextual Understanding: It leverages the relationships between data points for richer context.
- Multi-hop Reasoning: The graph structure allows the system to follow relationship chains, enabling more complex reasoning.
- Structured Knowledge Representation: Graphs naturally represent hierarchical and non-hierarchical relationships, surpassing the limitations of flat document structures.
- Improved Efficiency: Graph structures can optimize certain queries, particularly those involving relationship traversal.
The Graph RAG Process
The Graph RAG process unfolds as follows:
- Query Analysis: The input query is analyzed and transformed into a format suitable for graph querying.
- Graph Exploration: The system traverses the graph, following relevant relationships to identify connected information.
- Subgraph Extraction: Instead of retrieving isolated data points, relevant subgraphs capturing interconnected contexts are extracted.
- Context Synthesis: The retrieved subgraphs are combined and processed to create a coherent context.
- Response Generation: The LLM generates a response using the query and the integrated graph information.
Also Read: Constructing a RAG Pipeline Using LLama Index
Visualizing Graph RAG: A Flowchart
This flowchart visually represents the steps outlined above, illustrating the flow from query input to response generation via graph traversal, subgraph retrieval, and context integration.
Key Differences: Standard RAG vs. Graph RAG
The core differences lie in:
- Knowledge Representation: Standard RAG uses a flat structure; Graph RAG utilizes a graph structure.
- Retrieval Method: Standard RAG often employs vector similarity search; Graph RAG utilizes graph traversal algorithms.
- Contextual Awareness: Graph RAG captures more intricate, multi-step relationships.
- Reasoning Ability: Graph RAG's structure facilitates more sophisticated reasoning across interconnected information.
Applications and Challenges of Graph RAG
Challenges | Applications |
---|---|
Graph Construction: Creating and maintaining accurate, current knowledge graphs is resource-intensive. | Legal Research: Navigating complex legal networks. |
Scalability: Efficient traversal and retrieval become challenging as graphs grow. | Healthcare: Understanding medical knowledge, patient data, and treatment options. |
Query Interpretation: Translating natural language queries into effective graph queries is complex. | Financial Analysis: Analyzing intricate financial networks. |
Integration Complexity: Combining information from multiple subgraphs coherently is difficult. | Social Network Analysis: Exploring complex social structures. |
Knowledge Management: Enhancing corporate knowledge bases. |
Conclusion
Graph RAG represents a substantial advancement in retrieval-augmented generation. Its use of graph structures enables more nuanced and context-rich information retrieval and response generation. While challenges remain, particularly concerning implementation complexity and scalability, its potential across various fields makes it a promising area for ongoing research and development.
To learn more about Graph RAG: Click Here
Frequently Asked Questions
Q1. How does Graph RAG differ from standard RAG? Graph RAG utilizes graph-structured data, enabling more complex relationship modeling and multi-hop reasoning compared to standard RAG's flat document structure.
Q2. What are the key components of a Graph RAG system? A graph-structured knowledge base, a graph traversal mechanism, a subgraph retrieval system, an information integration module, and a response generator.
Q3. Where is Graph RAG particularly useful? In scientific research, healthcare, finance, law, social network analysis, and knowledge management.
Q4. What are the primary challenges in implementing Graph RAG? Graph construction and maintenance, scalability, complex query interpretation, and coherent information integration from multiple subgraphs.
Q5. How does Graph RAG improve upon traditional methods? It offers superior relational context understanding, enables multi-hop reasoning, provides a more natural representation of complex relationships, and can be more efficient for certain relationship-based queries.
The above is the detailed content of Graph RAG: Enhancing RAG with Graph Structures - Analytics Vidhya. For more information, please follow other related articles on the PHP Chinese website!

Hot AI Tools

Undresser.AI Undress
AI-powered app for creating realistic nude photos

AI Clothes Remover
Online AI tool for removing clothes from photos.

Undress AI Tool
Undress images for free

Clothoff.io
AI clothes remover

Video Face Swap
Swap faces in any video effortlessly with our completely free AI face swap tool!

Hot Article

Hot Tools

Notepad++7.3.1
Easy-to-use and free code editor

SublimeText3 Chinese version
Chinese version, very easy to use

Zend Studio 13.0.1
Powerful PHP integrated development environment

Dreamweaver CS6
Visual web development tools

SublimeText3 Mac version
God-level code editing software (SublimeText3)

Hot Topics










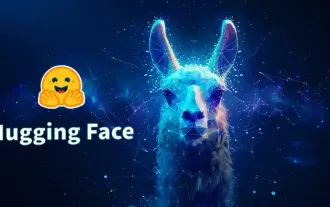
Meta's Llama 3.2: A Leap Forward in Multimodal and Mobile AI Meta recently unveiled Llama 3.2, a significant advancement in AI featuring powerful vision capabilities and lightweight text models optimized for mobile devices. Building on the success o
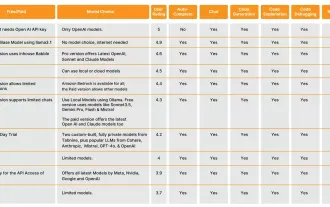
Hey there, Coding ninja! What coding-related tasks do you have planned for the day? Before you dive further into this blog, I want you to think about all your coding-related woes—better list those down. Done? – Let’
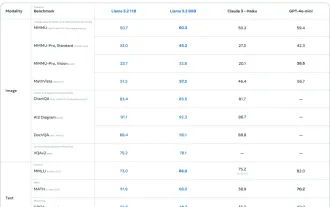
This week's AI landscape: A whirlwind of advancements, ethical considerations, and regulatory debates. Major players like OpenAI, Google, Meta, and Microsoft have unleashed a torrent of updates, from groundbreaking new models to crucial shifts in le
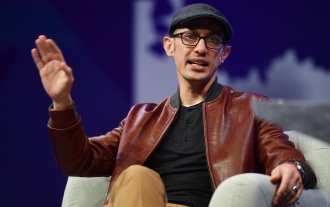
Shopify CEO Tobi Lütke's recent memo boldly declares AI proficiency a fundamental expectation for every employee, marking a significant cultural shift within the company. This isn't a fleeting trend; it's a new operational paradigm integrated into p
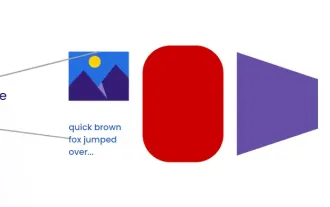
Introduction Imagine walking through an art gallery, surrounded by vivid paintings and sculptures. Now, what if you could ask each piece a question and get a meaningful answer? You might ask, “What story are you telling?
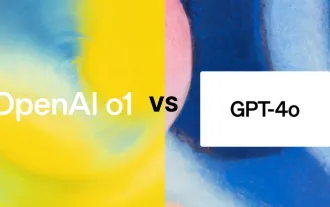
Introduction OpenAI has released its new model based on the much-anticipated “strawberry” architecture. This innovative model, known as o1, enhances reasoning capabilities, allowing it to think through problems mor
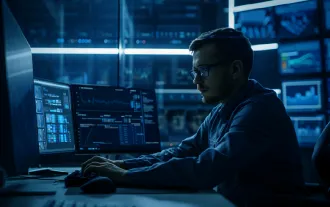
SQL's ALTER TABLE Statement: Dynamically Adding Columns to Your Database In data management, SQL's adaptability is crucial. Need to adjust your database structure on the fly? The ALTER TABLE statement is your solution. This guide details adding colu
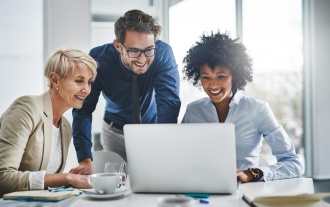
For those of you who might be new to my column, I broadly explore the latest advances in AI across the board, including topics such as embodied AI, AI reasoning, high-tech breakthroughs in AI, prompt engineering, training of AI, fielding of AI, AI re
