Comprehensive Guide on Reranker for RAG
Retrieval Augmented Generation (RAG) systems are transforming information access, but their effectiveness hinges on the quality of retrieved data. This is where rerankers become crucial – acting as a quality filter for search results to ensure only the most relevant information contributes to the final output.
This article delves into the world of rerankers, examining their importance, application scenarios, potential limitations, and various types. We'll also guide you through selecting the best reranker for your RAG system and evaluating its performance.
Table of Contents:
- What is a Reranker in RAG?
- Why Use a Reranker in RAG?
- Types of Rerankers
- Selecting the Optimal Reranker
- Recent Research
- Conclusion
What is a Reranker in RAG?
A reranker is a vital component of information retrieval systems, functioning as a secondary filter. Following an initial search (using techniques like semantic or keyword search), it receives a set of documents and reorders them based on relevance to a specific query. This process refines the search results, prioritizing the most pertinent information. Rerankers achieve this balance between speed and accuracy by employing more sophisticated matching methods than the initial retrieval phase.
This diagram illustrates a two-step search process. Reranking, the second step, refines the initial search results (based on semantic or keyword matching) to significantly enhance the relevance and order of the final results, providing a more precise and useful response to the user's query.
Why Use a Reranker in RAG?
Consider your RAG system as a chef, and the retrieved documents as ingredients. A delicious dish (accurate answer) requires the best ingredients. However, irrelevant or inappropriate ingredients can ruin the dish. Rerankers prevent this!
Here's why you need a reranker:
- Reduced Hallucinations: Rerankers filter out irrelevant documents that can lead to inaccurate or nonsensical LLM outputs (hallucinations).
- Cost Optimization: By focusing on the most relevant documents, you minimize the LLM's processing load, saving on API calls and computing resources.
Understanding Embedding Limitations:
Relying solely on embeddings for retrieval has limitations:
- Limited Semantic Nuance: Embeddings sometimes miss subtle contextual differences.
- Dimensionality Issues: Representing complex information in low-dimensional embedding space can lead to information loss.
- Generalization Challenges: Embeddings may struggle to accurately retrieve information outside their training data.
Reranker Advantages:
Rerankers overcome these embedding limitations by:
- Bag-of-Embeddings Approach: Processing documents as smaller, contextualized units rather than single vector representations.
- Semantic Keyword Matching: Combining the power of encoder models (like BERT) with keyword-based techniques for both semantic and keyword relevance.
- Improved Generalization: Handling unseen documents and queries more effectively due to the focus on smaller, contextualized units.
This image shows a query searching a vector database, retrieving the top 25 documents. These are then passed to a reranker module, which refines the results, selecting the top 3 for the final output.
Types of Rerankers:
The field of rerankers is constantly evolving. Here are the main types:
Approach | Examples | Access Type | Performance Level | Cost Range |
---|---|---|---|---|
Cross Encoder | Sentence Transformers, Flashrank | Open-source | Excellent | Moderate |
Multi-Vector | ColBERT | Open-source | Good | Low |
Fine-tuned LLM | RankZephyr, RankT5 | Open-source | Excellent | High |
LLM as a Judge | GPT, Claude, Gemini | Proprietary | Top-tier | Very Expensive |
Reranking API | Cohere, Jina | Proprietary | Excellent | Moderate |
Selecting the Optimal Reranker:
Choosing the right reranker involves considering:
- Relevance Enhancement: Measure the improvement in relevance using metrics like NDCG.
- Latency: The additional time the reranker adds to the search process.
- Contextual Understanding: The reranker's ability to handle varied context lengths.
- Generalization: The reranker's performance across different domains and datasets.
Recent Research:
Recent studies highlight the effectiveness and efficiency of cross-encoders, especially when combined with robust retrievers. Cross-encoders often outperform many LLMs in reranking, while maintaining better efficiency.
Conclusion:
Selecting the appropriate reranker is crucial for optimizing RAG systems and ensuring accurate search results. Understanding the different types of rerankers and their strengths and weaknesses is essential for building effective and efficient RAG applications. Careful selection and evaluation will lead to improved accuracy and performance.
The above is the detailed content of Comprehensive Guide on Reranker for RAG. For more information, please follow other related articles on the PHP Chinese website!

Hot AI Tools

Undresser.AI Undress
AI-powered app for creating realistic nude photos

AI Clothes Remover
Online AI tool for removing clothes from photos.

Undress AI Tool
Undress images for free

Clothoff.io
AI clothes remover

Video Face Swap
Swap faces in any video effortlessly with our completely free AI face swap tool!

Hot Article

Hot Tools

Notepad++7.3.1
Easy-to-use and free code editor

SublimeText3 Chinese version
Chinese version, very easy to use

Zend Studio 13.0.1
Powerful PHP integrated development environment

Dreamweaver CS6
Visual web development tools

SublimeText3 Mac version
God-level code editing software (SublimeText3)

Hot Topics
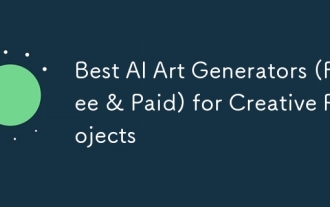
The article reviews top AI art generators, discussing their features, suitability for creative projects, and value. It highlights Midjourney as the best value for professionals and recommends DALL-E 2 for high-quality, customizable art.
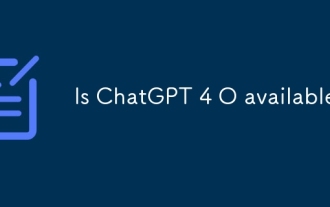
ChatGPT 4 is currently available and widely used, demonstrating significant improvements in understanding context and generating coherent responses compared to its predecessors like ChatGPT 3.5. Future developments may include more personalized interactions and real-time data processing capabilities, further enhancing its potential for various applications.
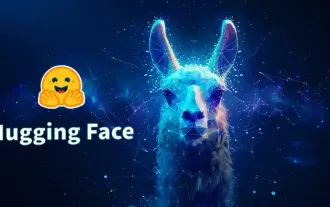
Meta's Llama 3.2: A Leap Forward in Multimodal and Mobile AI Meta recently unveiled Llama 3.2, a significant advancement in AI featuring powerful vision capabilities and lightweight text models optimized for mobile devices. Building on the success o
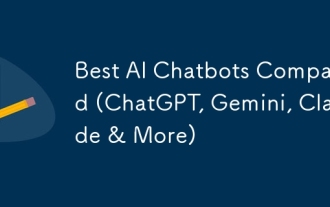
The article compares top AI chatbots like ChatGPT, Gemini, and Claude, focusing on their unique features, customization options, and performance in natural language processing and reliability.
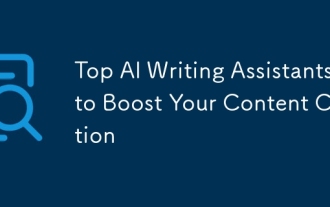
The article discusses top AI writing assistants like Grammarly, Jasper, Copy.ai, Writesonic, and Rytr, focusing on their unique features for content creation. It argues that Jasper excels in SEO optimization, while AI tools help maintain tone consist
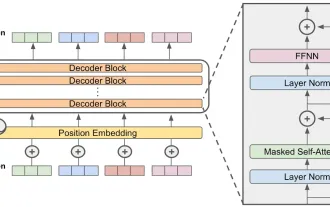
Falcon 3: A Revolutionary Open-Source Large Language Model Falcon 3, the latest iteration in the acclaimed Falcon series of LLMs, represents a significant advancement in AI technology. Developed by the Technology Innovation Institute (TII), this open
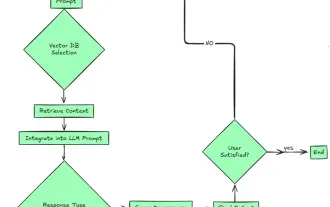
2024 witnessed a shift from simply using LLMs for content generation to understanding their inner workings. This exploration led to the discovery of AI Agents – autonomous systems handling tasks and decisions with minimal human intervention. Buildin
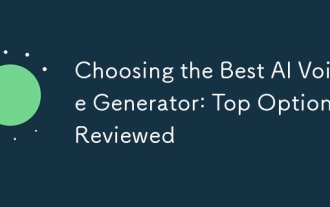
The article reviews top AI voice generators like Google Cloud, Amazon Polly, Microsoft Azure, IBM Watson, and Descript, focusing on their features, voice quality, and suitability for different needs.
