


Analysis of image processing skills in PHP introductory tutorial
本文实例讲述了PHP图像处理。分享给大家供大家参考,具体如下:
Demo1.php
<?php //一般生成的图像可以是 png,jpg,gif,bmp //jpeg,wbmp //第一步,设置文件MIME类型,输出类型 text/html 类型是网页类型,默认可以不写 //将输出类型改成图像流 header('Content-Type:image/png;'); //第二步,创建一个图形区域,图像背景 //有两种创建方式,资源类型,一般要加上 @ 符号,防止出错 //imagecreatetruecolor 返回的是一个资源句柄 //这个函数创建了一个图像的区域,没有进行填充的时候,背景默认是黑色的 $im = imagecreatetruecolor(200,200); //第三步,有空白图像区域,绘制颜色,文字叫,线条啊。。。 //填充色换掉,首先要有个颜色填充器 //imagecolorallocate -- 为一幅图像分配颜色 $blue = imagecolorallocate($im,0,102,255); //将这个 blue 颜色填充到背景上去 //imagefill -- 区域填充 imagefill($im,0,0,$blue); //第四部,在蓝色的背景上输入一些线条,文字等 $white = imagecolorallocate($im,255,255,255); //imageline -- 画一条线段 imageline($im,0,0,200,200,$white); imageline($im,200,0,0,200,$white); //imagestring -- 水平地画一行字符串 imagestring($im,5,80,20,'Mr.One',$white); //第五步,输出最终图形 //以 PNG 格式将图像输出到浏览器或文件 imagepng($im); //第六步,我要将所有的资源全部清空 imagedestroy($im); ?>
Demo2.php
<?php //src 可以插入各样类型的图片 //Demo1.php 其实就是一张 png 图片 header('Content-Type:text/html; charset=gbk'); echo '<img src="Demo1.php" alt="图形"/>'; ?>
Demo3.php
<?php //简单的验证码 //随机数 //为什么要循环 0-15 之间的数呢? //因为要实现最简单的字母和数字混搭 //十六进制 0-9 a-f //dechex -- 十进制转换为十六进制 //创建一个四位的验证码 for($i=0;$i<4;$i++){ $nmsg .= dechex(mt_rand(0,15)); } //echo $nmsg; header('Content-Type:image/png;'); $im = imagecreatetruecolor(75,25); $blue = imagecolorallocate($im,0,102,255); $white = imagecolorallocate($im,255,255,255); imagefill($im,0,0,$blue); imagestring($im,5,20,5,$nmsg,$white); imagepng($im); imagedestroy($im); ?>
Demo4.php
<?php define('__DIR__',dirname(__FILE__).'\\'); //加载已有的图像 header('Content-Type:image/png;'); //header('Content-Type:image/jpeg;'); //imagecreatefrompng -- 从 PNG 文件或 URL 新建一图像 //用 image 载入图像,是可以编辑图像 //在载入的图像中,加入一个小水印 $im = imagecreatefrompng(__DIR__.'ss.png'); //$im = imagecreatefromjpeg('xx.jpg'); $white = imagecolorallocate($im,255,255,255); imagestring($im,5,10,10,'http://www.oneStopWeb.cn',$white); imagepng($im); //imagejpeg($im); imagedestroy($im); ?>
Demo5.php
<?php define('__DIR__',dirname(__FILE__).'\\'); //加载已有的图像 header('Content-Type:image/png;'); //header('Content-Type:image/jpeg;'); //imagecreatefrompng -- 从 PNG 文件或 URL 新建一图像 //用 image 载入图像,是可以编辑图像 //在载入的图像中,加入一个小水印 $im = imagecreatefrompng(__DIR__.'ss.png'); //$im = imagecreatefromjpeg('xx.jpg'); $white = imagecolorallocate($im,255,255,255); imagestring($im,5,10,10,'http://www.oneStopWeb.cn',$white); //font 字体还必须支持中文 $font = 'C:\WINDOWS\Fonts\SIMHEI.TTF'; //字体文件 $text = iconv('gbk','utf-8','阅谁问君诵'); //采用系统提供的字体 //第二参数,是字体的大小,第三个参数是旋转角度,4,5参数是坐标 imagettftext($im,20,10,50,100,$white,$font,$text); imagepng($im); //imagejpeg($im); imagedestroy($im); ?>
Demo6.php
<?php //微缩图,不但表面的大小改变了,容量也改变了 //是真的改变了,不是表面的缩小 define('__DIR__',dirname(__FILE__).'\\'); header('Content-Type:image/png;'); //getimagesize -- 取得图像大小 //获取到了原图的长度和高度 list($width,$height) = getimagesize(__DIR__.'ss.png'); //将原图缩放成 40% $_width = $width * 0.4; $_height = $height * 0.4; //创建一个新图 $im = imagecreatetruecolor($_width,$_height); //下面的工作是,载入原图,将原图复制到新图上去 //载入原图 $_im = imagecreatefrompng(__DIR__.'ss.png'); //将原图重新采样,拷贝到新图上,最后按 0.4 的比例输出 //imagecopyresampled -- 重采样拷贝部分图像并调整大小 imagecopyresampled($im,$_im,0,0,0,0,$_width,$_height,$width,$height); //将新图输出 imagepng($im); //第二个参数不需要,直接 null 过度 //第三个参数,是 0-100 来调节 JPG 的清晰度 //如果是 imagepng,那么全部都是高清 //imagejpeg($im,null,50); //销毁 imagedestroy($im); imagedestroy($_im); ?>
希望本文所述对大家PHP程序设计有所帮助。
更多PHP入门教程之图像处理技巧分析相关文章请关注PHP中文网!

Hot AI Tools

Undresser.AI Undress
AI-powered app for creating realistic nude photos

AI Clothes Remover
Online AI tool for removing clothes from photos.

Undress AI Tool
Undress images for free

Clothoff.io
AI clothes remover

Video Face Swap
Swap faces in any video effortlessly with our completely free AI face swap tool!

Hot Article

Hot Tools

Notepad++7.3.1
Easy-to-use and free code editor

SublimeText3 Chinese version
Chinese version, very easy to use

Zend Studio 13.0.1
Powerful PHP integrated development environment

Dreamweaver CS6
Visual web development tools

SublimeText3 Mac version
God-level code editing software (SublimeText3)

Hot Topics


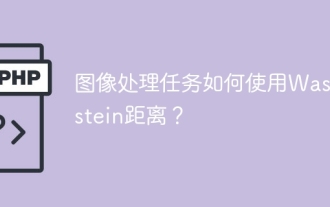
Wasserstein distance, also known as EarthMover's Distance (EMD), is a metric used to measure the difference between two probability distributions. Compared with traditional KL divergence or JS divergence, Wasserstein distance takes into account the structural information between distributions and therefore exhibits better performance in many image processing tasks. By calculating the minimum transportation cost between two distributions, Wasserstein distance is able to measure the minimum amount of work required to transform one distribution into another. This metric is able to capture the geometric differences between distributions, thereby playing an important role in tasks such as image generation and style transfer. Therefore, the Wasserstein distance becomes the concept
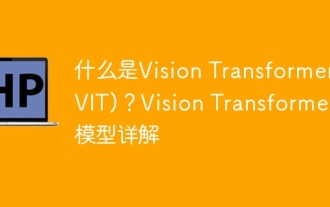
VisionTransformer (VIT) is a Transformer-based image classification model proposed by Google. Different from traditional CNN models, VIT represents images as sequences and learns the image structure by predicting the class label of the image. To achieve this, VIT divides the input image into multiple patches and concatenates the pixels in each patch through channels and then performs linear projection to achieve the desired input dimensions. Finally, each patch is flattened into a single vector, forming the input sequence. Through Transformer's self-attention mechanism, VIT is able to capture the relationship between different patches and perform effective feature extraction and classification prediction. This serialized image representation is
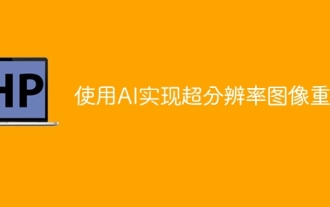
Super-resolution image reconstruction is the process of generating high-resolution images from low-resolution images using deep learning techniques, such as convolutional neural networks (CNN) and generative adversarial networks (GAN). The goal of this method is to improve the quality and detail of images by converting low-resolution images into high-resolution images. This technology has wide applications in many fields, such as medical imaging, surveillance cameras, satellite images, etc. Through super-resolution image reconstruction, we can obtain clearer and more detailed images, which helps to more accurately analyze and identify targets and features in images. Reconstruction methods Super-resolution image reconstruction methods can generally be divided into two categories: interpolation-based methods and deep learning-based methods. 1) Interpolation-based method Super-resolution image reconstruction based on interpolation
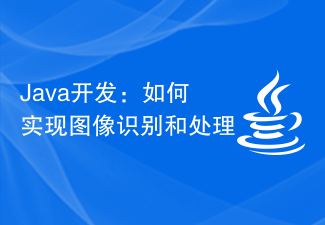
Java Development: A Practical Guide to Image Recognition and Processing Abstract: With the rapid development of computer vision and artificial intelligence, image recognition and processing play an important role in various fields. This article will introduce how to use Java language to implement image recognition and processing, and provide specific code examples. 1. Basic principles of image recognition Image recognition refers to the use of computer technology to analyze and understand images to identify objects, features or content in the image. Before performing image recognition, we need to understand some basic image processing techniques, as shown in the figure
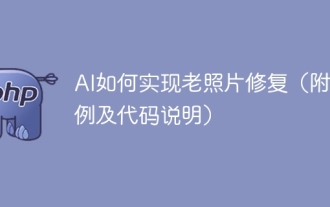
Old photo restoration is a method of using artificial intelligence technology to repair, enhance and improve old photos. Using computer vision and machine learning algorithms, the technology can automatically identify and repair damage and flaws in old photos, making them look clearer, more natural and more realistic. The technical principles of old photo restoration mainly include the following aspects: 1. Image denoising and enhancement. When restoring old photos, they need to be denoised and enhanced first. Image processing algorithms and filters, such as mean filtering, Gaussian filtering, bilateral filtering, etc., can be used to solve noise and color spots problems, thereby improving the quality of photos. 2. Image restoration and repair In old photos, there may be some defects and damage, such as scratches, cracks, fading, etc. These problems can be solved by image restoration and repair algorithms
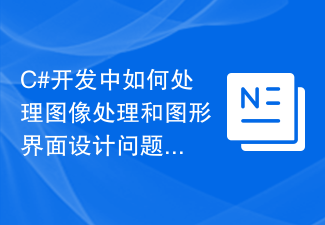
How to deal with image processing and graphical interface design issues in C# development requires specific code examples. Introduction: In modern software development, image processing and graphical interface design are common requirements. As a general-purpose high-level programming language, C# has powerful image processing and graphical interface design capabilities. This article will be based on C#, discuss how to deal with image processing and graphical interface design issues, and give detailed code examples. 1. Image processing issues: Image reading and display: In C#, image reading and display are basic operations. Can be used.N
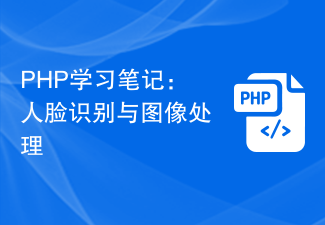
PHP study notes: Face recognition and image processing Preface: With the development of artificial intelligence technology, face recognition and image processing have become hot topics. In practical applications, face recognition and image processing are mostly used in security monitoring, face unlocking, card comparison, etc. As a commonly used server-side scripting language, PHP can also be used to implement functions related to face recognition and image processing. This article will take you through face recognition and image processing in PHP, with specific code examples. 1. Face recognition in PHP Face recognition is a
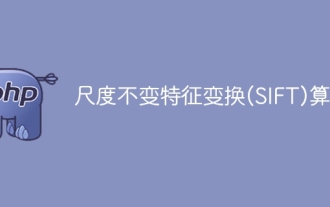
The Scale Invariant Feature Transform (SIFT) algorithm is a feature extraction algorithm used in the fields of image processing and computer vision. This algorithm was proposed in 1999 to improve object recognition and matching performance in computer vision systems. The SIFT algorithm is robust and accurate and is widely used in image recognition, three-dimensional reconstruction, target detection, video tracking and other fields. It achieves scale invariance by detecting key points in multiple scale spaces and extracting local feature descriptors around the key points. The main steps of the SIFT algorithm include scale space construction, key point detection, key point positioning, direction assignment and feature descriptor generation. Through these steps, the SIFT algorithm can extract robust and unique features, thereby achieving efficient image processing.
