Writing observer pattern structure in Python program
Observer pattern definition
defines one-to-many dependencies between objects, so that when an object changes state, all its dependencies will be collected to notifications and automatically updated. The Observer pattern provides an object design that provides loose coupling between subjects and observers.
Design principles
Strive for loose coupling design between interactive objects. The reason why loosely coupled design allows us to build a flexible system that can cope with changes is because the interdependence between objects is reduced to a minimum.
Mode structure and description
1.Subject: Topic (target) interface, topics can be registered and removed Observers who are interested in it can also notify these observers when their own data changes. A subject can have multiple observers, it is important to note that the order of observers should not matter. The subject is also called the "observable" (Observable)
2. All observers must implement the Observer interface so that they can be notified when the subject status changes
The observer pattern is a widely used, very An important design pattern that helps reduce coupling between objects while allowing objects to maintain a high degree of collaboration. The loose coupling characteristics of this pattern are reflected in:
1. Everything about the observer, the subject only knows that it implements the observer interface, and nothing else is known.
2. At any time, the subject's observer can be dynamically Adding and deleting
3. When a new type of observer appears, the code of the topic does not need to be modified
4. Modifying either the topic or the observer will not affect the other party
Example
You can understand it clearly through comments~
#observer pattern class subject(): def __init__(self,dat): self.listobj = list() #主题中的列表变量用来存储观察者 self.dat = dat #主题中的数据变化时,需要通知观察者 def registerObject(self,obj): self.listobj.append(obj) #实现订阅主题的函数 def removeObject(self,obj): pass def notifyObservers(self):#通知各个观察者数据已经发生变化,观察者相应需要更新自己的数据 for i in range(len(self.listobj)): self.listobj[i].update() def setdat(self,new_dat):#设置数据 if self.dat != new_dat: self.dat = new_dat self.notifyObservers() class observer():#观察者 def __init__(self,sub): #观察者初始化时,需要订阅主题 self.sub = sub self.sub.registerObject(self) self.number = self.sub.dat def update(self):#观察者更新 self.number = self.sub.dat def display(self): print self.number if __name__ =="__main__": su = subject(10) ob1 = observer(su) ob1.display() ob2 = observer(su) ob2.display() su.setdat(11) ob1.display() ob2.display()
More Python For articles related to writing observer pattern structures in programs, please pay attention to the PHP Chinese website!

Hot AI Tools

Undresser.AI Undress
AI-powered app for creating realistic nude photos

AI Clothes Remover
Online AI tool for removing clothes from photos.

Undress AI Tool
Undress images for free

Clothoff.io
AI clothes remover

AI Hentai Generator
Generate AI Hentai for free.

Hot Article

Hot Tools

Notepad++7.3.1
Easy-to-use and free code editor

SublimeText3 Chinese version
Chinese version, very easy to use

Zend Studio 13.0.1
Powerful PHP integrated development environment

Dreamweaver CS6
Visual web development tools

SublimeText3 Mac version
God-level code editing software (SublimeText3)

Hot Topics


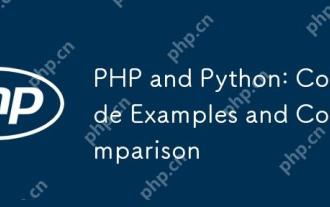
PHP and Python have their own advantages and disadvantages, and the choice depends on project needs and personal preferences. 1.PHP is suitable for rapid development and maintenance of large-scale web applications. 2. Python dominates the field of data science and machine learning.
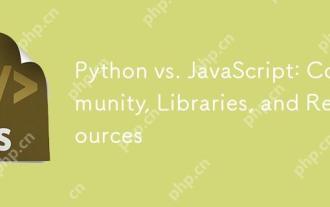
Python and JavaScript have their own advantages and disadvantages in terms of community, libraries and resources. 1) The Python community is friendly and suitable for beginners, but the front-end development resources are not as rich as JavaScript. 2) Python is powerful in data science and machine learning libraries, while JavaScript is better in front-end development libraries and frameworks. 3) Both have rich learning resources, but Python is suitable for starting with official documents, while JavaScript is better with MDNWebDocs. The choice should be based on project needs and personal interests.
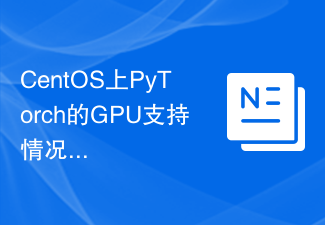
Enable PyTorch GPU acceleration on CentOS system requires the installation of CUDA, cuDNN and GPU versions of PyTorch. The following steps will guide you through the process: CUDA and cuDNN installation determine CUDA version compatibility: Use the nvidia-smi command to view the CUDA version supported by your NVIDIA graphics card. For example, your MX450 graphics card may support CUDA11.1 or higher. Download and install CUDAToolkit: Visit the official website of NVIDIACUDAToolkit and download and install the corresponding version according to the highest CUDA version supported by your graphics card. Install cuDNN library:
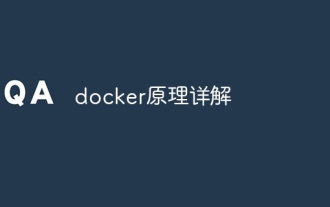
Docker uses Linux kernel features to provide an efficient and isolated application running environment. Its working principle is as follows: 1. The mirror is used as a read-only template, which contains everything you need to run the application; 2. The Union File System (UnionFS) stacks multiple file systems, only storing the differences, saving space and speeding up; 3. The daemon manages the mirrors and containers, and the client uses them for interaction; 4. Namespaces and cgroups implement container isolation and resource limitations; 5. Multiple network modes support container interconnection. Only by understanding these core concepts can you better utilize Docker.
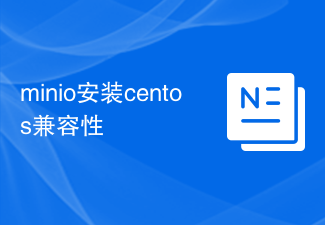
MinIO Object Storage: High-performance deployment under CentOS system MinIO is a high-performance, distributed object storage system developed based on the Go language, compatible with AmazonS3. It supports a variety of client languages, including Java, Python, JavaScript, and Go. This article will briefly introduce the installation and compatibility of MinIO on CentOS systems. CentOS version compatibility MinIO has been verified on multiple CentOS versions, including but not limited to: CentOS7.9: Provides a complete installation guide covering cluster configuration, environment preparation, configuration file settings, disk partitioning, and MinI
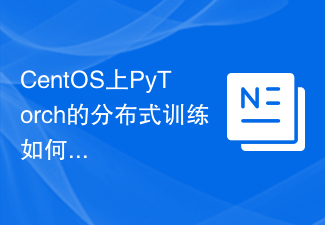
PyTorch distributed training on CentOS system requires the following steps: PyTorch installation: The premise is that Python and pip are installed in CentOS system. Depending on your CUDA version, get the appropriate installation command from the PyTorch official website. For CPU-only training, you can use the following command: pipinstalltorchtorchvisiontorchaudio If you need GPU support, make sure that the corresponding version of CUDA and cuDNN are installed and use the corresponding PyTorch version for installation. Distributed environment configuration: Distributed training usually requires multiple machines or single-machine multiple GPUs. Place
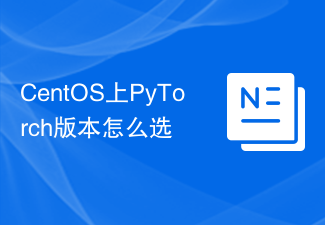
When installing PyTorch on CentOS system, you need to carefully select the appropriate version and consider the following key factors: 1. System environment compatibility: Operating system: It is recommended to use CentOS7 or higher. CUDA and cuDNN:PyTorch version and CUDA version are closely related. For example, PyTorch1.9.0 requires CUDA11.1, while PyTorch2.0.1 requires CUDA11.3. The cuDNN version must also match the CUDA version. Before selecting the PyTorch version, be sure to confirm that compatible CUDA and cuDNN versions have been installed. Python version: PyTorch official branch
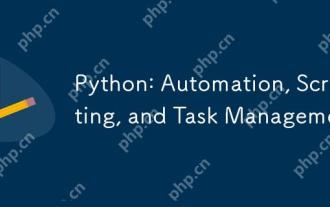
Python excels in automation, scripting, and task management. 1) Automation: File backup is realized through standard libraries such as os and shutil. 2) Script writing: Use the psutil library to monitor system resources. 3) Task management: Use the schedule library to schedule tasks. Python's ease of use and rich library support makes it the preferred tool in these areas.
