NLTK learning: classifying and annotating vocabulary
[TOC]
Part-of-speech tagger
Many subsequent tasks require tagged words. nltk comes with its own English tagger
pos_tag
import nltk text = nltk.word_tokenize("And now for something compleyely difference")print(text)print(nltk.pos_tag(text))
annotation corpus
represents the tagged identifier: nltk.tag.str2tuple('word/type')
text = "The/AT grand/JJ is/VBD ."print([nltk.tag.str2tuple(t) for t in text.split()])
Read the annotated corpus
nltk corpus ue navel provides unification interface, you can ignore different file formats. Format:
Corpus.tagged_word()/tagged_sents()
. Parameters can specify categories and fields
print(nltk.corpus.brown.tagged_words())
Nouns, verbs, adjectives, etc.
Here we take nouns as an example
from nltk.corpus import brown word_tag = nltk.FreqDist(brown.tagged_words(categories="news"))print([word+'/'+tag for (word,tag)in word_tag if tag.startswith('V')])################下面是查找money的不同标注#################################wsj = brown.tagged_words(categories="news") cfd = nltk.ConditionalFreqDist(wsj)print(cfd['money'].keys())
Try to find the most frequent noun of each noun type
def findtag(tag_prefix,tagged_text): cfd = nltk.ConditionalFreqDist((tag,word) for (word,tag) in tagged_text if tag.startswith(tag_prefix))return dict((tag,list(cfd[tag].keys())[:5]) for tag in cfd.conditions())#数据类型必须转换为list才能进行切片操作tagdict = findtag('NN',nltk.corpus.brown.tagged_words(categories="news"))for tag in sorted(tagdict):print(tag,tagdict[tag])
Explore the annotated corpus
Required
nltk.bigrams()
andnltk.trigrams()
correspond to the 2-gram model and the 3-gram model respectively.
brown_tagged = brown.tagged_words(categories="learned") tags = [b[1] for (a,b) in nltk.bigrams(brown_tagged) if a[0]=="often"] fd = nltk.FreqDist(tags) fd.tabulate()
Automatic tagging
Default tagger
The simplest tagger is for each identifier Assign uniform tags. Below is a tagger that turns all words into NN. And use
evaluate()
to test. It facilitates first analysis and improves stability when many words are nouns.
brown_tagged_sents = brown.tagged_sents(categories="news") raw = 'I do not like eggs and ham, I do not like them Sam I am'tokens = nltk.word_tokenize(raw) default_tagger = nltk.DefaultTagger('NN')#创建标注器print(default_tagger.tag(tokens)) # 调用tag()方法进行标注print(default_tagger.evaluate(brown_tagged_sents))
Regular expression tagger
Note that the rules here are fixed (determined by yourself). As the rules become more and more complete, the accuracy becomes higher.
patterns = [ (r'.*ing$','VBG'), (r'.*ed$','VBD'), (r'.*es$','VBZ'), (r'.*','NN')#为了方便,只有少量规则] regexp_tagger = nltk.RegexpTagger(patterns) regexp_tagger.evaluate(brown_tagged_sents)
Query annotator
There is a difference between this and the book. It is different from python2. Pay attention to debugging. The query tagger stores the most likely tags, and the
backoff
parameter can be set. If the tag cannot be marked, use this tagger (this process is backoff)
fd = nltk.FreqDist(brown.words(categories="news")) cfd = nltk.ConditionalFreqDist(brown.tagged_words(categories="news"))##############################################python2和3的区别#########most_freq_words = fd.most_common(100) likely_tags = dict((word,cfd[word].max()) for (word,times) in most_freq_words)#######################################################################baseline_tagger = nltk.UnigramTagger(model=likely_tags,backoff=nltk.DefaultTagger('NN')) baseline_tagger.evaluate(brown_tagged_sents)
N-gram annotation
Basic unary annotator
The behavior of the unary annotator and the search annotator Very similar to the techniques used to build unary annotators, train for .
Here our annotator only memorizes the training set instead of building a general model. The agreement is very good, but it cannot be generalized to new texts.
size = int(len(brown_tagged_sents)*0.9) train_sents = brown_tagged_sents[:size] test_sents = brown_tagged_sents[size+1:] unigram_tagger = nltk.UnigramTagger(train_sents) unigram_tagger.evaluate(test_sents)
General N-gram tagger
N-gram tagger is to retrieve the word with index= n, and retrieve n-N< ;=index<=n-1 tag. That is, the tag of the current word is further determined through the tag tag of the previous word. Similar to
nltk.UnigramTagger()
, the built-in binary tagger is:nltk.BigramTagger()
The usage is consistent.
Combined Tagger
Many times, an algorithm with wider coverage is more useful than an algorithm with higher accuracy. Use
backoff
to specify the backoff annotator to implement the combination of annotators. If the parametercutoff
is explicitly declared as int type, contexts that only appear 1-n times will be automatically discarded.
t0 = nltk.DefaultTagger('NN') t1 = nltk.UnigramTagger(train_sents,backoff=t0) t2 = nltk.BigramTagger(train_sents,backoff=t1) t2.evaluate(test_sents)
It can be found that after comparing with the original, the accuracy is significantly improved
Cross-sentence boundary marking
For the beginning of the sentence of words, there are no first n words. Solution: Train the tagger with tagged tagged_sents.
Transformation-based annotation: Brill annotator
is superior to the above. The idea of implementation: start with a big stroke, then fix the details, and make detailed changes little by little.
Not only does it take up a small amount of memory, but it is also associated with the context, and corrects errors in real time as the problem becomes smaller, rather than static. Of course, the calls are different in python3 and python2.
from nltk.tag import brill brill.nltkdemo18plus() brill.nltkdemo18()
The above is the detailed content of NLTK learning: classifying and annotating vocabulary. For more information, please follow other related articles on the PHP Chinese website!

Hot AI Tools

Undresser.AI Undress
AI-powered app for creating realistic nude photos

AI Clothes Remover
Online AI tool for removing clothes from photos.

Undress AI Tool
Undress images for free

Clothoff.io
AI clothes remover

Video Face Swap
Swap faces in any video effortlessly with our completely free AI face swap tool!

Hot Article

Hot Tools

Notepad++7.3.1
Easy-to-use and free code editor

SublimeText3 Chinese version
Chinese version, very easy to use

Zend Studio 13.0.1
Powerful PHP integrated development environment

Dreamweaver CS6
Visual web development tools

SublimeText3 Mac version
God-level code editing software (SublimeText3)

Hot Topics


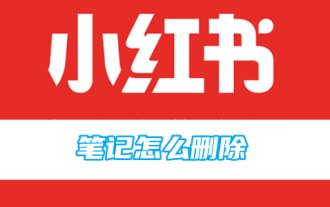
How to delete Xiaohongshu notes? Notes can be edited in the Xiaohongshu APP. Most users don’t know how to delete Xiaohongshu notes. Next, the editor brings users pictures and texts on how to delete Xiaohongshu notes. Tutorial, interested users come and take a look! Xiaohongshu usage tutorial How to delete Xiaohongshu notes 1. First open the Xiaohongshu APP and enter the main page, select [Me] in the lower right corner to enter the special area; 2. Then in the My area, click on the note page shown in the picture below , select the note you want to delete; 3. Enter the note page, click [three dots] in the upper right corner; 4. Finally, the function bar will expand at the bottom, click [Delete] to complete.
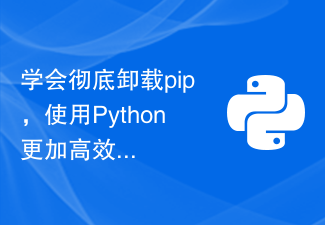
No more need for pip? Come and learn how to uninstall pip effectively! Introduction: pip is one of Python's package management tools, which can easily install, upgrade and uninstall Python packages. However, sometimes we may need to uninstall pip, perhaps because we wish to use another package management tool, or because we need to completely clear the Python environment. This article will explain how to uninstall pip efficiently and provide specific code examples. 1. How to uninstall pip The following will introduce two common methods of uninstalling pip.
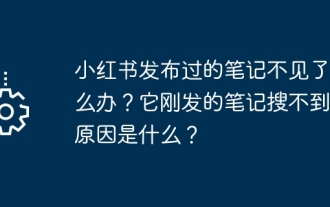
As a Xiaohongshu user, we have all encountered the situation where published notes suddenly disappeared, which is undoubtedly confusing and worrying. In this case, what should we do? This article will focus on the topic of "What to do if the notes published by Xiaohongshu are missing" and give you a detailed answer. 1. What should I do if the notes published by Xiaohongshu are missing? First, don't panic. If you find that your notes are missing, staying calm is key and don't panic. This may be caused by platform system failure or operational errors. Checking release records is easy. Just open the Xiaohongshu App and click "Me" → "Publish" → "All Publications" to view your own publishing records. Here you can easily find previously published notes. 3.Repost. If found
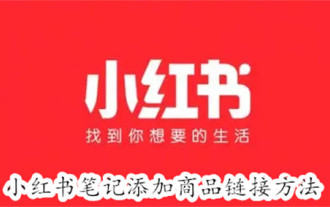
How to add product links in notes in Xiaohongshu? In the Xiaohongshu app, users can not only browse various contents but also shop, so there is a lot of content about shopping recommendations and good product sharing in this app. If If you are an expert on this app, you can also share some shopping experiences, find merchants for cooperation, add links in notes, etc. Many people are willing to use this app for shopping, because it is not only convenient, but also has many Experts will make some recommendations. You can browse interesting content and see if there are any clothing products that suit you. Let’s take a look at how to add product links to notes! How to add product links to Xiaohongshu Notes Open the app on the desktop of your mobile phone. Click on the app homepage
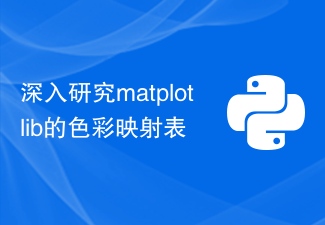
To learn more about the matplotlib color table, you need specific code examples 1. Introduction matplotlib is a powerful Python drawing library. It provides a rich set of drawing functions and tools that can be used to create various types of charts. The colormap (colormap) is an important concept in matplotlib, which determines the color scheme of the chart. In-depth study of the matplotlib color table will help us better master the drawing functions of matplotlib and make drawings more convenient.
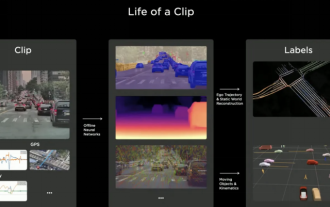
1. Problems faced by data annotation (especially based on BEV tasks) With the rise of BEV transformer-based tasks, the dependence on data has become heavier and heavier, and the annotation based on BEV tasks has also become more and more complex. important. At present, whether it is 2D-3D joint obstacle annotation, lane line annotation based on reconstructed point cloud clips or Occpuancy task annotation, it is still too expensive (compared with 2D annotation tasks, it is much more expensive). Of course, there are also many semi-automatic or automated annotation studies based on large models in the industry. On the other hand, the data collection cycle for autonomous driving is too long and involves a series of data compliance issues. For example, you want to capture the field of a flatbed truck across the camera.
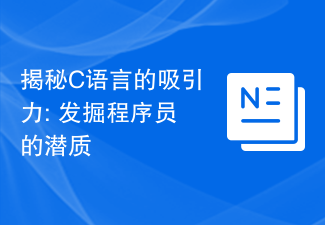
The Charm of Learning C Language: Unlocking the Potential of Programmers With the continuous development of technology, computer programming has become a field that has attracted much attention. Among many programming languages, C language has always been loved by programmers. Its simplicity, efficiency and wide application make learning C language the first step for many people to enter the field of programming. This article will discuss the charm of learning C language and how to unlock the potential of programmers by learning C language. First of all, the charm of learning C language lies in its simplicity. Compared with other programming languages, C language
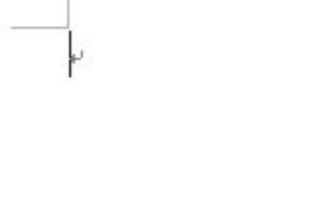
When editing text content in Word, you sometimes need to enter formula symbols. Some guys don’t know how to input the root number in Word, so Xiaomian asked me to share with my friends a tutorial on how to input the root number in Word. Hope it helps my friends. First, open the Word software on your computer, then open the file you want to edit, and move the cursor to the location where you need to insert the root sign, refer to the picture example below. 2. Select [Insert], and then select [Formula] in the symbol. As shown in the red circle in the picture below: 3. Then select [Insert New Formula] below. As shown in the red circle in the picture below: 4. Select [Radical Formula], and then select the appropriate root sign. As shown in the red circle in the picture below:
