Implementation of K-means algorithm in Python
K-means is a commonly used algorithm in machine learning. It is an unsupervised learning algorithm. It is often used for data clustering. You only need to specify the number of clusters for it to automatically aggregate data into multiple categories. , the data similarity in the same cluster is high, and the data similarity in different clusters is low.
The K-MEANS algorithm is an algorithm that inputs the number of clusters k and a database containing n data objects, and outputs k clusters that meet the minimum variance criterion. The k-means algorithm accepts an input quantity k; then divides n data objects into k clusters so that the obtained clusters satisfy: the similarity of objects in the same cluster is higher; and the similarity of objects in different clusters is smaller. This article will introduce to you the implementation of the K-means algorithm in Python.
Core idea
Iteratively find a partitioning scheme for k clusters, so that the mean value of these k clusters is used to represent the corresponding types of samples. The overall error is the smallest.
k clusters have the following characteristics: each cluster itself is as compact as possible, and each cluster is as separated as possible.
The basis of the k-means algorithm is the minimum error sum of squares criterion. The advantages and disadvantages of K-menas:
Advantages:
Simple principle
Fast speed
Good scalability for large data sets
Disadvantages:
Need to specify The number of clusters K
is sensitive to outliers
is sensitive to initial values
The clustering process of K-means
its The clustering process is similar to the gradient descent algorithm. A cost function is established and the value of the cost function becomes smaller and smaller through iteration.
Appropriately select the initial centers of c classes;
In the k-th iteration, for any For a sample, find the distance to c centers, and classify the sample into the class of the center with the shortest distance;
Use methods such as mean to update the center value of the class;
For all c cluster centers , if the value remains unchanged after updating using the iteration method of (2) (3), the iteration ends, otherwise the iteration continues.
The biggest advantage of this algorithm is its simplicity and speed. The key to the algorithm lies in the selection of the initial center and the distance formula.
K-means example shows
Some parameters of km in python:
sklearn.cluster.KMeans( n_clusters=8, init='k-means++', n_init=10, max_iter=300, tol=0.0001, precompute_distances='auto', verbose=0, random_state=None, copy_x=True, n_jobs=1, algorithm='auto' ) n_clusters: 簇的个数,即你想聚成几类 init: 初始簇中心的获取方法 n_init: 获取初始簇中心的更迭次数,为了弥补初始质心的影响,算法默认会初始10个质心,实现算法,然后返回最好的结果。 max_iter: 最大迭代次数(因为kmeans算法的实现需要迭代) tol: 容忍度,即kmeans运行准则收敛的条件 precompute_distances:是否需要提前计算距离,这个参数会在空间和时间之间做权衡,如果是True 会把整个距离矩阵都放到内存中,auto 会默认在数据样本大于featurs*samples 的数量大于12e6 的时候False,False 时核心实现的方法是利用Cpython 来实现的 verbose: 冗长模式(不太懂是啥意思,反正一般不去改默认值) random_state: 随机生成簇中心的状态条件。 copy_x: 对是否修改数据的一个标记,如果True,即复制了就不会修改数据。bool 在scikit-learn 很多接口中都会有这个参数的,就是是否对输入数据继续copy 操作,以便不修改用户的输入数据。这个要理解Python 的内存机制才会比较清楚。 n_jobs: 并行设置 algorithm: kmeans的实现算法,有:'auto', ‘full', ‘elkan', 其中 ‘full'表示用EM方式实现 虽然有很多参数,但是都已经给出了默认值。所以我们一般不需要去传入这些参数,参数的。可以根据实际需要来调用。
The following shows a code example
from sklearn.cluster import KMeans from sklearn.externals import joblib from sklearn import cluster import numpy as np # 生成10*3的矩阵 data = np.random.rand(10,3) print data # 聚类为4类 estimator=KMeans(n_clusters=4) # fit_predict表示拟合+预测,也可以分开写 res=estimator.fit_predict(data) # 预测类别标签结果 lable_pred=estimator.labels_ # 各个类别的聚类中心值 centroids=estimator.cluster_centers_ # 聚类中心均值向量的总和 inertia=estimator.inertia_ print lable_pred print centroids print inertia 代码执行结果 [0 2 1 0 2 2 0 3 2 0] [[ 0.3028348 0.25183096 0.62493622] [ 0.88481287 0.70891813 0.79463764] [ 0.66821961 0.54817207 0.30197415] [ 0.11629904 0.85684903 0.7088385 ]] 0.570794546829
For a more intuitive description, this time it is done on the picture A demonstration. Since it is more intuitive to draw two dimensions on the image, the data was adjusted to two dimensions. 100 points were selected to draw. The clustering category was 3 categories
from sklearn.cluster import KMeans from sklearn.externals import joblib from sklearn import cluster import numpy as np import matplotlib.pyplot as plt data = np.random.rand(100,2) estimator=KMeans(n_clusters=3) res=estimator.fit_predict(data) lable_pred=estimator.labels_ centroids=estimator.cluster_centers_ inertia=estimator.inertia_ #print res print lable_pred print centroids print inertia for i in range(len(data)): if int(lable_pred[i])==0: plt.scatter(data[i][0],data[i][1],color='red') if int(lable_pred[i])==1: plt.scatter(data[i][0],data[i][1],color='black') if int(lable_pred[i])==2: plt.scatter(data[i][0],data[i][1],color='blue') plt.show()
It can be seen that the clustering effect is still good. We conducted a test on the clustering efficiency of k-means and expanded the dimension to 50 dimensions
Data scale | Time consumption | Data dimensions |
---|---|---|
10000 items | 4s | 50dimensional |
100000 items | 30s | 50 Dimensions |
4'13s | 50 dimensions |
from sklearn.externals import joblib joblib.dump(km,"model/km_model.m")
Use k-means clustering algorithm to identify the main color of the picture
Use k-means clustering algorithm to identify the picture Main Color_PHP Tutorial
Image Understanding K-Means Algorithm
The above is the detailed content of Implementation of K-means algorithm in Python. For more information, please follow other related articles on the PHP Chinese website!

Hot AI Tools

Undresser.AI Undress
AI-powered app for creating realistic nude photos

AI Clothes Remover
Online AI tool for removing clothes from photos.

Undress AI Tool
Undress images for free

Clothoff.io
AI clothes remover

AI Hentai Generator
Generate AI Hentai for free.

Hot Article

Hot Tools

Notepad++7.3.1
Easy-to-use and free code editor

SublimeText3 Chinese version
Chinese version, very easy to use

Zend Studio 13.0.1
Powerful PHP integrated development environment

Dreamweaver CS6
Visual web development tools

SublimeText3 Mac version
God-level code editing software (SublimeText3)

Hot Topics



HadiDB: A lightweight, high-level scalable Python database HadiDB (hadidb) is a lightweight database written in Python, with a high level of scalability. Install HadiDB using pip installation: pipinstallhadidb User Management Create user: createuser() method to create a new user. The authentication() method authenticates the user's identity. fromhadidb.operationimportuseruser_obj=user("admin","admin")user_obj.
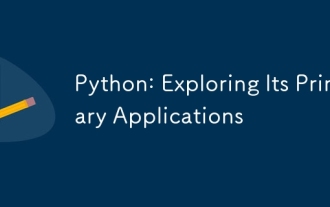
Python is widely used in the fields of web development, data science, machine learning, automation and scripting. 1) In web development, Django and Flask frameworks simplify the development process. 2) In the fields of data science and machine learning, NumPy, Pandas, Scikit-learn and TensorFlow libraries provide strong support. 3) In terms of automation and scripting, Python is suitable for tasks such as automated testing and system management.
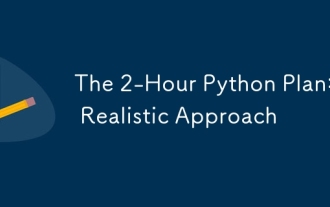
You can learn basic programming concepts and skills of Python within 2 hours. 1. Learn variables and data types, 2. Master control flow (conditional statements and loops), 3. Understand the definition and use of functions, 4. Quickly get started with Python programming through simple examples and code snippets.
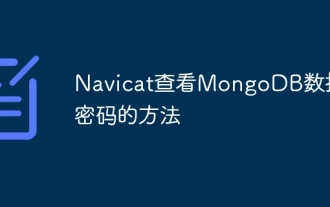
It is impossible to view MongoDB password directly through Navicat because it is stored as hash values. How to retrieve lost passwords: 1. Reset passwords; 2. Check configuration files (may contain hash values); 3. Check codes (may hardcode passwords).
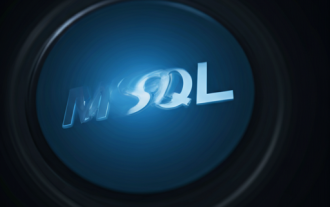
MySQL database performance optimization guide In resource-intensive applications, MySQL database plays a crucial role and is responsible for managing massive transactions. However, as the scale of application expands, database performance bottlenecks often become a constraint. This article will explore a series of effective MySQL performance optimization strategies to ensure that your application remains efficient and responsive under high loads. We will combine actual cases to explain in-depth key technologies such as indexing, query optimization, database design and caching. 1. Database architecture design and optimized database architecture is the cornerstone of MySQL performance optimization. Here are some core principles: Selecting the right data type and selecting the smallest data type that meets the needs can not only save storage space, but also improve data processing speed.
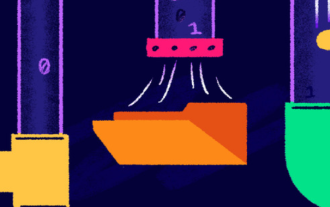
As a data professional, you need to process large amounts of data from various sources. This can pose challenges to data management and analysis. Fortunately, two AWS services can help: AWS Glue and Amazon Athena.
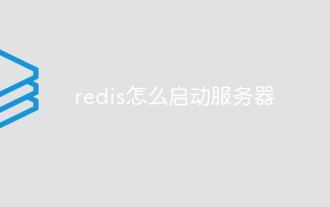
The steps to start a Redis server include: Install Redis according to the operating system. Start the Redis service via redis-server (Linux/macOS) or redis-server.exe (Windows). Use the redis-cli ping (Linux/macOS) or redis-cli.exe ping (Windows) command to check the service status. Use a Redis client, such as redis-cli, Python, or Node.js, to access the server.
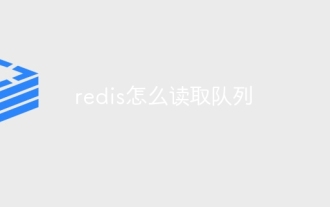
To read a queue from Redis, you need to get the queue name, read the elements using the LPOP command, and process the empty queue. The specific steps are as follows: Get the queue name: name it with the prefix of "queue:" such as "queue:my-queue". Use the LPOP command: Eject the element from the head of the queue and return its value, such as LPOP queue:my-queue. Processing empty queues: If the queue is empty, LPOP returns nil, and you can check whether the queue exists before reading the element.
