How to implement decision tree algorithm in python? (code)
The content of this article is about how to implement the decision tree algorithm in Python? (code). It has certain reference value. Friends in need can refer to it. I hope it will be helpful to you.
Data description
Each data item is stored in a list, and the last column stores the result
Multiple data items form a data set
data=[[d1,d2,d3...dn,result], [d1,d2,d3...dn,result], . . [d1,d2,d3...dn,result]]
Decision tree data structure
class DecisionNode: '''决策树节点 ''' def __init__(self,col=-1,value=None,results=None,tb=None,fb=None): '''初始化决策树节点 args: col -- 按数据集的col列划分数据集 value -- 以value作为划分col列的参照 result -- 只有叶子节点有,代表最终划分出的子数据集结果统计信息。{‘结果’:结果出现次数} rb,fb -- 代表左右子树 ''' self.col=col self.value=value self.results=results self.tb=tb self.fb=fb
The final result of the decision tree classification is to divide the data items into several subsets, where the results of each subset are The same, so here we use {'result': the number of occurrences of the result} to express each subset
def pideset(rows,column,value): '''依据数据集rows的column列的值,判断其与参考值value的关系对数据集进行拆分 返回两个数据集 ''' split_function=None #value是数值类型 if isinstance(value,int) or isinstance(value,float): #定义lambda函数当row[column]>=value时返回true split_function=lambda row:row[column]>=value #value是字符类型 else: #定义lambda函数当row[column]==value时返回true split_function=lambda row:row[column]==value #将数据集拆分成两个 set1=[row for row in rows if split_function(row)] set2=[row for row in rows if not split_function(row)] #返回两个数据集 return (set1,set2) def uniquecounts(rows): '''计算数据集rows中有几种最终结果,计算结果出现次数,返回一个字典 ''' results={} for row in rows: r=row[len(row)-1] if r not in results: results[r]=0 results[r]+=1 return results def giniimpurity(rows): '''返回rows数据集的基尼不纯度 ''' total=len(rows) counts=uniquecounts(rows) imp=0 for k1 in counts: p1=float(counts[k1])/total for k2 in counts: if k1==k2: continue p2=float(counts[k2])/total imp+=p1*p2 return imp def entropy(rows): '''返回rows数据集的熵 ''' from math import log log2=lambda x:log(x)/log(2) results=uniquecounts(rows) ent=0.0 for r in results.keys(): p=float(results[r])/len(rows) ent=ent-p*log2(p) return ent def build_tree(rows,scoref=entropy): '''构造决策树 ''' if len(rows)==0: return DecisionNode() current_score=scoref(rows) # 最佳信息增益 best_gain=0.0 # best_criteria=None #最佳划分 best_sets=None column_count=len(rows[0])-1 #遍历数据集的列,确定分割顺序 for col in range(0,column_count): column_values={} # 构造字典 for row in rows: column_values[row[col]]=1 for value in column_values.keys(): (set1,set2)=pideset(rows,col,value) p=float(len(set1))/len(rows) # 计算信息增益 gain=current_score-p*scoref(set1)-(1-p)*scoref(set2) if gain>best_gain and len(set1)>0 and len(set2)>0: best_gain=gain best_criteria=(col,value) best_sets=(set1,set2) # 如果划分的两个数据集熵小于原数据集,进一步划分它们 if best_gain>0: trueBranch=build_tree(best_sets[0]) falseBranch=build_tree(best_sets[1]) return DecisionNode(col=best_criteria[0],value=best_criteria[1], tb=trueBranch,fb=falseBranch) # 如果划分的两个数据集熵不小于原数据集,停止划分 else: return DecisionNode(results=uniquecounts(rows)) def print_tree(tree,indent=''): if tree.results!=None: print(str(tree.results)) else: print(str(tree.col)+':'+str(tree.value)+'? ') print(indent+'T->',end='') print_tree(tree.tb,indent+' ') print(indent+'F->',end='') print_tree(tree.fb,indent+' ') def getwidth(tree): if tree.tb==None and tree.fb==None: return 1 return getwidth(tree.tb)+getwidth(tree.fb) def getdepth(tree): if tree.tb==None and tree.fb==None: return 0 return max(getdepth(tree.tb),getdepth(tree.fb))+1 def drawtree(tree,jpeg='tree.jpg'): w=getwidth(tree)*100 h=getdepth(tree)*100+120 img=Image.new('RGB',(w,h),(255,255,255)) draw=ImageDraw.Draw(img) drawnode(draw,tree,w/2,20) img.save(jpeg,'JPEG') def drawnode(draw,tree,x,y): if tree.results==None: # Get the width of each branch w1=getwidth(tree.fb)*100 w2=getwidth(tree.tb)*100 # Determine the total space required by this node left=x-(w1+w2)/2 right=x+(w1+w2)/2 # Draw the condition string draw.text((x-20,y-10),str(tree.col)+':'+str(tree.value),(0,0,0)) # Draw links to the branches draw.line((x,y,left+w1/2,y+100),fill=(255,0,0)) draw.line((x,y,right-w2/2,y+100),fill=(255,0,0)) # Draw the branch nodes drawnode(draw,tree.fb,left+w1/2,y+100) drawnode(draw,tree.tb,right-w2/2,y+100) else: txt=' \n'.join(['%s:%d'%v for v in tree.results.items()]) draw.text((x-20,y),txt,(0,0,0))
Classify the test data (with the processing of missing data)
def mdclassify(observation,tree): '''对缺失数据进行分类 args: observation -- 发生信息缺失的数据项 tree -- 训练完成的决策树 返回代表该分类的结果字典 ''' # 判断数据是否到达叶节点 if tree.results!=None: # 已经到达叶节点,返回结果result return tree.results else: # 对数据项的col列进行分析 v=observation[tree.col] # 若col列数据缺失 if v==None: #对tree的左右子树分别使用mdclassify,tr是左子树得到的结果字典,fr是右子树得到的结果字典 tr,fr=mdclassify(observation,tree.tb),mdclassify(observation,tree.fb) # 分别以结果占总数比例计算得到左右子树的权重 tcount=sum(tr.values()) fcount=sum(fr.values()) tw=float(tcount)/(tcount+fcount) fw=float(fcount)/(tcount+fcount) result={} # 计算左右子树的加权平均 for k,v in tr.items(): result[k]=v*tw for k,v in fr.items(): # fr的结果k有可能并不在tr中,在result中初始化k if k not in result: result[k]=0 # fr的结果累加到result中 result[k]+=v*fw return result # col列没有缺失,继续沿决策树分类 else: if isinstance(v,int) or isinstance(v,float): if v>=tree.value: branch=tree.tb else: branch=tree.fb else: if v==tree.value: branch=tree.tb else: branch=tree.fb return mdclassify(observation,branch) tree=build_tree(my_data) print(mdclassify(['google',None,'yes',None],tree)) print(mdclassify(['google','France',None,None],tree))
Decision tree pruning
def prune(tree,mingain): '''对决策树进行剪枝 args: tree -- 决策树 mingain -- 最小信息增益 返回 ''' # 修剪非叶节点 if tree.tb.results==None: prune(tree.tb,mingain) if tree.fb.results==None: prune(tree.fb,mingain) #合并两个叶子节点 if tree.tb.results!=None and tree.fb.results!=None: tb,fb=[],[] for v,c in tree.tb.results.items(): tb+=[[v]]*c for v,c in tree.fb.results.items(): fb+=[[v]]*c #计算熵减少情况 delta=entropy(tb+fb)-(entropy(tb)+entropy(fb)/2) #熵的增加量小于mingain,可以合并分支 if delta<mingain: tree.tb,tree.fb=None,None tree.results=uniquecounts(tb+fb)
The above is the detailed content of How to implement decision tree algorithm in python? (code). For more information, please follow other related articles on the PHP Chinese website!

Hot AI Tools

Undresser.AI Undress
AI-powered app for creating realistic nude photos

AI Clothes Remover
Online AI tool for removing clothes from photos.

Undress AI Tool
Undress images for free

Clothoff.io
AI clothes remover

AI Hentai Generator
Generate AI Hentai for free.

Hot Article

Hot Tools

Notepad++7.3.1
Easy-to-use and free code editor

SublimeText3 Chinese version
Chinese version, very easy to use

Zend Studio 13.0.1
Powerful PHP integrated development environment

Dreamweaver CS6
Visual web development tools

SublimeText3 Mac version
God-level code editing software (SublimeText3)

Hot Topics


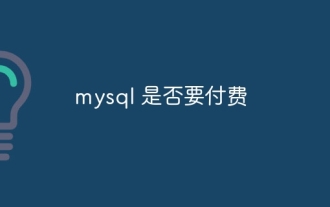
MySQL has a free community version and a paid enterprise version. The community version can be used and modified for free, but the support is limited and is suitable for applications with low stability requirements and strong technical capabilities. The Enterprise Edition provides comprehensive commercial support for applications that require a stable, reliable, high-performance database and willing to pay for support. Factors considered when choosing a version include application criticality, budgeting, and technical skills. There is no perfect option, only the most suitable option, and you need to choose carefully according to the specific situation.
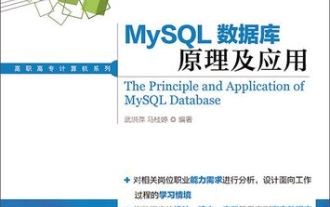
The article introduces the operation of MySQL database. First, you need to install a MySQL client, such as MySQLWorkbench or command line client. 1. Use the mysql-uroot-p command to connect to the server and log in with the root account password; 2. Use CREATEDATABASE to create a database, and USE select a database; 3. Use CREATETABLE to create a table, define fields and data types; 4. Use INSERTINTO to insert data, query data, update data by UPDATE, and delete data by DELETE. Only by mastering these steps, learning to deal with common problems and optimizing database performance can you use MySQL efficiently.
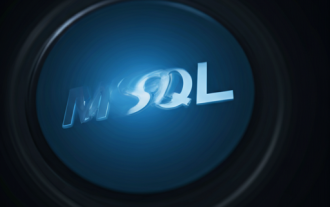
MySQL database performance optimization guide In resource-intensive applications, MySQL database plays a crucial role and is responsible for managing massive transactions. However, as the scale of application expands, database performance bottlenecks often become a constraint. This article will explore a series of effective MySQL performance optimization strategies to ensure that your application remains efficient and responsive under high loads. We will combine actual cases to explain in-depth key technologies such as indexing, query optimization, database design and caching. 1. Database architecture design and optimized database architecture is the cornerstone of MySQL performance optimization. Here are some core principles: Selecting the right data type and selecting the smallest data type that meets the needs can not only save storage space, but also improve data processing speed.

HadiDB: A lightweight, high-level scalable Python database HadiDB (hadidb) is a lightweight database written in Python, with a high level of scalability. Install HadiDB using pip installation: pipinstallhadidb User Management Create user: createuser() method to create a new user. The authentication() method authenticates the user's identity. fromhadidb.operationimportuseruser_obj=user("admin","admin")user_obj.
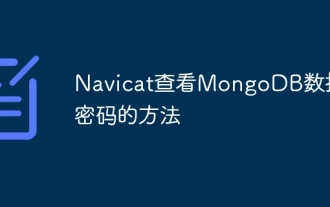
It is impossible to view MongoDB password directly through Navicat because it is stored as hash values. How to retrieve lost passwords: 1. Reset passwords; 2. Check configuration files (may contain hash values); 3. Check codes (may hardcode passwords).
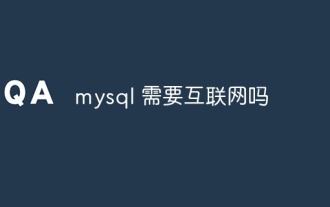
MySQL can run without network connections for basic data storage and management. However, network connection is required for interaction with other systems, remote access, or using advanced features such as replication and clustering. Additionally, security measures (such as firewalls), performance optimization (choose the right network connection), and data backup are critical to connecting to the Internet.
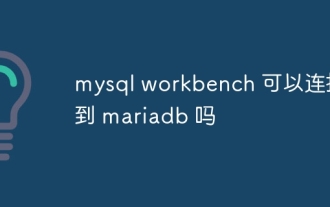
MySQL Workbench can connect to MariaDB, provided that the configuration is correct. First select "MariaDB" as the connector type. In the connection configuration, set HOST, PORT, USER, PASSWORD, and DATABASE correctly. When testing the connection, check that the MariaDB service is started, whether the username and password are correct, whether the port number is correct, whether the firewall allows connections, and whether the database exists. In advanced usage, use connection pooling technology to optimize performance. Common errors include insufficient permissions, network connection problems, etc. When debugging errors, carefully analyze error information and use debugging tools. Optimizing network configuration can improve performance
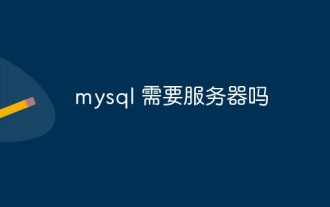
For production environments, a server is usually required to run MySQL, for reasons including performance, reliability, security, and scalability. Servers usually have more powerful hardware, redundant configurations and stricter security measures. For small, low-load applications, MySQL can be run on local machines, but resource consumption, security risks and maintenance costs need to be carefully considered. For greater reliability and security, MySQL should be deployed on cloud or other servers. Choosing the appropriate server configuration requires evaluation based on application load and data volume.
