What is closure in Python? What are the applications?
The content of this article is about what is closure in Python? What are the applications? It has certain reference value. Friends in need can refer to it. I hope it will be helpful to you.
1. Function as return value
Before introducing "closure", let's first understand the situation of function as return value.
In addition to receiving functions as parameters, higher-order functions can also return functions as result values. For example, in the decorator introduced before, functions are used as return values.
2. Closure
1. Conditions and effects of closure
What is closure?
When nesting another function within a function, closure may occur if the inner function refers to the variables of the outer function.
SoThe three conditions for closure generation (one is indispensable):
1. An internal function must be nested
2. The internal function must reference the variables of the external function
3. The external function must return the internal function
So why should we try closures and what are their functions?
1. Closures can obtain different results based on the local variables of external functions.
2. After the closure is executed, the The current running environment can be maintained, and the execution result depends on the last running result of the function
2. Closure example
Chestnut 1: Find the sum of the sequence
>>> def calc_sum(*args): ... ax = 0 ... for n in args: ... ax = ax + n ... return ax # 返回变量 ... >>> calc_sum(1,2,3) 6
However, if you do not need to obtain the summation result immediately, but calculate it as needed in the subsequent code, what should you do?
We can not return the summation result, but return the summation function, as follows:
>>>def lazy_sum(*args): ... def sum(): # sum()是内部函数,可以利用外部函数的参数 ... ax = 0 ... for n in args: # sum()中使用外部函数的局部变量 ... ax = ax + n ... return ax ... return sum # 形成闭包,此时,*args保存在返回的函数中 ... >>>f = lazy_sum(1,3,5,7,9) >>>f # 此时返回的是求和函数 >>> f() # 调用函数f()时,才真正计算求和的结果 25
Note:
The internal execution sequence of the lazy_sum() function, when executing f, runs At return sum, *args is stored in the return function, and what is returned is the sum() function. When executing f(), it is equivalent to executing sum() and includes *args.
When we call lazy_sun(), a new function will be returned every time. Even if the same parameters are passed in, the result of the f() call will not be affected.
Let’s verify the second point:
# 但是调用 f1() 与f2()的调用结果互不影响 >>> f1 = lazy_sum(1,3,5,7,9) >>> f2 = lazy_sum(1,3,5,7,9) >>> f1 <function lazy_sum.<locals>.sum at 0x013DD618> >>> f2 <function lazy_sum.<locals>.sum at 0x02F92DF8> >>> f1 == f2 False >>> f1() == f2() True >>> f1() 25 >>> f2() 25 >>> id(f1()) 1627215984 >>> id(f2()) 1627215984
Explanation: The positions of the return functions of f1 and f2 are different, so the return result of f1==f2 is False.
But it does not affect the final execution result. The execution results of f1() and f2() are both 25, and they can be viewed with id(), which points to the same area.
Chestnut 2:
def count(): fs = [] for i in range(1, 4): def f(): # 返回函数f()放在循环里 return i*i fs.append(f) return fs f1, f2, f3 = count()
The actual execution result is: f1=9 f2=9 f3=9
It may be a little different from what you actually thought ([1,4,9]) . Because the f() function is placed in the for loop, only when the loop ends, the execution result 9 of i=3 is finally returned.
So it is best not to reference any loop variables in the return function, or the amount that may change in the future. So how to modify it?
def count(): def f(j): def g(): return j*j # 形成闭包 return g fs = [] for i in range(1, 4): fs.append(f(i)) # 一个i值进入后,f(i)立刻被执行,并加入到fs中 return fs f1, f2, f3 = count() # 返回函数g没有引用j
Final result: [1,4,9] That is, f1=1 f2=4 f3=
3. Anonymous function lambda
Definition: Anonymous function refers to a type of function or subroutine that does not need to define an identifier function name. Python allows you to create anonymous functions using the lambda keyword.
Syntax: lambda parameter: expression
Or lambda formal parameter 1,..., formal parameter n: function (formal parameter), input parameter 1,..., input parameter n
Note : 1. The lambda function can receive any number of parameters and return the value of a single expression;
2. The lambda cannot contain commands and cannot return more than one expression.
Advantages: 1. The process of defining functions can be omitted and the code can be streamlined;
2. Some abstract functions that will not be reused can be defined with lambda.
Example:
>>> list( map( lambda x: x*x ,[1,2,3] ) ) [1, 4, 9]
Where lamdba x : x*x
implements: def f(x): return x*x
Function.
You can assign an anonymous function to a variable and then use the variable to call the function. For example:
>>> f = lambda x:x*x >>> f(5) # 调用 >>> g = lambda x,y=2 : x*y >>> g(2,4) 8 >>> g(2) # 默认y=2 4
You can return anonymous functions as return values, for example:
return lambda x:x*x
The above is the detailed content of What is closure in Python? What are the applications?. For more information, please follow other related articles on the PHP Chinese website!

Hot AI Tools

Undresser.AI Undress
AI-powered app for creating realistic nude photos

AI Clothes Remover
Online AI tool for removing clothes from photos.

Undress AI Tool
Undress images for free

Clothoff.io
AI clothes remover

AI Hentai Generator
Generate AI Hentai for free.

Hot Article

Hot Tools

Notepad++7.3.1
Easy-to-use and free code editor

SublimeText3 Chinese version
Chinese version, very easy to use

Zend Studio 13.0.1
Powerful PHP integrated development environment

Dreamweaver CS6
Visual web development tools

SublimeText3 Mac version
God-level code editing software (SublimeText3)

Hot Topics


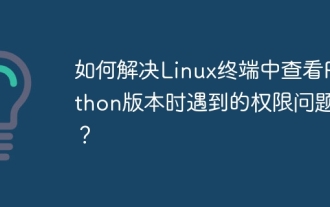
Solution to permission issues when viewing Python version in Linux terminal When you try to view Python version in Linux terminal, enter python...
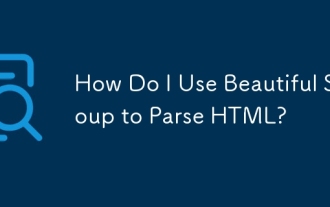
This article explains how to use Beautiful Soup, a Python library, to parse HTML. It details common methods like find(), find_all(), select(), and get_text() for data extraction, handling of diverse HTML structures and errors, and alternatives (Sel
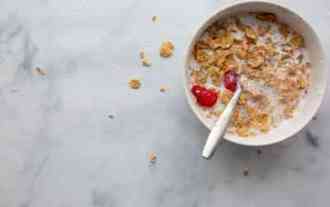
Serialization and deserialization of Python objects are key aspects of any non-trivial program. If you save something to a Python file, you do object serialization and deserialization if you read the configuration file, or if you respond to an HTTP request. In a sense, serialization and deserialization are the most boring things in the world. Who cares about all these formats and protocols? You want to persist or stream some Python objects and retrieve them in full at a later time. This is a great way to see the world on a conceptual level. However, on a practical level, the serialization scheme, format or protocol you choose may determine the speed, security, freedom of maintenance status, and other aspects of the program
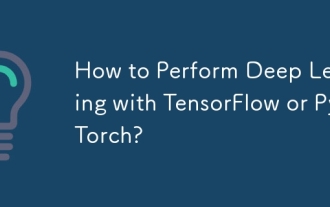
This article compares TensorFlow and PyTorch for deep learning. It details the steps involved: data preparation, model building, training, evaluation, and deployment. Key differences between the frameworks, particularly regarding computational grap
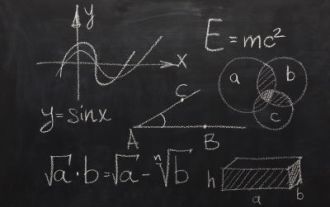
Python's statistics module provides powerful data statistical analysis capabilities to help us quickly understand the overall characteristics of data, such as biostatistics and business analysis. Instead of looking at data points one by one, just look at statistics such as mean or variance to discover trends and features in the original data that may be ignored, and compare large datasets more easily and effectively. This tutorial will explain how to calculate the mean and measure the degree of dispersion of the dataset. Unless otherwise stated, all functions in this module support the calculation of the mean() function instead of simply summing the average. Floating point numbers can also be used. import random import statistics from fracti
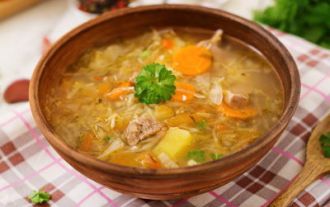
This tutorial builds upon the previous introduction to Beautiful Soup, focusing on DOM manipulation beyond simple tree navigation. We'll explore efficient search methods and techniques for modifying HTML structure. One common DOM search method is ex
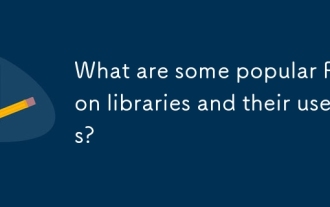
The article discusses popular Python libraries like NumPy, Pandas, Matplotlib, Scikit-learn, TensorFlow, Django, Flask, and Requests, detailing their uses in scientific computing, data analysis, visualization, machine learning, web development, and H
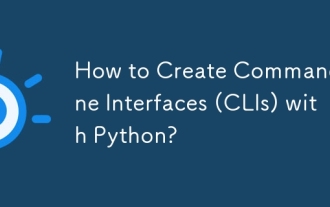
This article guides Python developers on building command-line interfaces (CLIs). It details using libraries like typer, click, and argparse, emphasizing input/output handling, and promoting user-friendly design patterns for improved CLI usability.
