


Introduction to the method of reading custom data for Tensorflow classifier project (code example)
This article brings you an introduction to the method of reading custom data for the Tensorflow classifier project (code example). It has certain reference value. Friends in need can refer to it. , hope it helps you.
Tensorflow classifier project custom data reading
After typing the code of the classifier project according to the demo on the Tensorflow official website, the operation was successful. The result Not bad. But in the end, I still have to train my own data, so I tried to prepare to load custom data. However, fashion_mnist.load_data() only appeared in the demo without a detailed reading process. Then I found some information and explained the reading process. Recorded here.
First mention the modules you need to use:
import os import keras import matplotlib.pyplot as plt from PIL import Image from keras.preprocessing.image import ImageDataGenerator from sklearn.model_selection import train_test_split
Image classifier project, first determine what the resolution of the image you want to process will be, the example here is 30 pixels:
IMG_SIZE_X = 30 IMG_SIZE_Y = 30
Secondly determine the directory of your pictures:
image_path = r'D:\Projects\ImageClassifier\data\set' path = ".\data" # 你也可以使用相对路径的方式 # image_path =os.path.join(path, "set")
The structure under the directory is as follows:
The corresponding label.txt is as follows:
动漫 风景 美女 物语 樱花
Next is connected to labels.txt, as follows:
label_name = "labels.txt" label_path = os.path.join(path, label_name) class_names = np.loadtxt(label_path, type(""))
For the sake of simplicity, numpy's loadtxt function is directly used to load directly.
After that, the image data is officially processed, and the comments are written inside:
re_load = False re_build = False # re_load = True re_build = True data_name = "data.npz" data_path = os.path.join(path, data_name) model_name = "model.h5" model_path = os.path.join(path, model_name) count = 0 # 这里判断是否存在序列化之后的数据,re_load是一个开关,是否强制重新处理,测试用,可以去除。 if not os.path.exists(data_path) or re_load: labels = [] images = [] print('Handle images') # 由于label.txt是和图片防止目录的分类目录一一对应的,即每个子目录的目录名就是labels.txt里的一个label,所以这里可以通过读取class_names的每一项去拼接path后读取 for index, name in enumerate(class_names): # 这里是拼接后的子目录path classpath = os.path.join(image_path, name) # 先判断一下是否是目录 if not os.path.isdir(classpath): continue # limit是测试时候用的这里可以去除 limit = 0 for image_name in os.listdir(classpath): if limit >= max_size: break # 这里是拼接后的待处理的图片path imagepath = os.path.join(classpath, image_name) count = count + 1 limit = limit + 1 # 利用Image打开图片 img = Image.open(imagepath) # 缩放到你最初确定要处理的图片分辨率大小 img = img.resize((IMG_SIZE_X, IMG_SIZE_Y)) # 转为灰度图片,这里彩色通道会干扰结果,并且会加大计算量 img = img.convert("L") # 转为numpy数组 img = np.array(img) # 由(30,30)转为(1,30,30)(即`channels_first`),当然你也可以转换为(30,30,1)(即`channels_last`)但为了之后预览处理后的图片方便这里采用了(1,30,30)的格式存放 img = np.reshape(img, (1, IMG_SIZE_X, IMG_SIZE_Y)) # 这里利用循环生成labels数据,其中存放的实际是class_names中对应元素的索引 labels.append([index]) # 添加到images中,最后统一处理 images.append(img) # 循环中一些状态的输出,可以去除 print("{} class: {} {} limit: {} {}" .format(count, index + 1, class_names[index], limit, imagepath)) # 最后一次性将images和labels都转换成numpy数组 npy_data = np.array(images) npy_labels = np.array(labels) # 处理数据只需要一次,所以我们选择在这里利用numpy自带的方法将处理之后的数据序列化存储 np.savez(data_path, x=npy_data, y=npy_labels) print("Save images by npz") else: # 如果存在序列化号的数据,便直接读取,提高速度 npy_data = np.load(data_path)["x"] npy_labels = np.load(data_path)["y"] print("Load images by npz") image_data = npy_data labels_data = npy_labels
At this point, the processing and preprocessing of the original data has been completed. Only the last step is needed, just like in the demo fashion_mnist.load_data()
The results returned are the same. The code is as follows:
# 最后一步就是将原始数据分成训练数据和测试数据 train_images, test_images, train_labels, test_labels = \ train_test_split(image_data, labels_data, test_size=0.2, random_state=6)
The method of printing relevant information is also attached here:
print("_________________________________________________________________") print("%-28s %-s" % ("Name", "Shape")) print("=================================================================") print("%-28s %-s" % ("Image Data", image_data.shape)) print("%-28s %-s" % ("Labels Data", labels_data.shape)) print("=================================================================") print('Split train and test data,p=%') print("_________________________________________________________________") print("%-28s %-s" % ("Name", "Shape")) print("=================================================================") print("%-28s %-s" % ("Train Images", train_images.shape)) print("%-28s %-s" % ("Test Images", test_images.shape)) print("%-28s %-s" % ("Train Labels", train_labels.shape)) print("%-28s %-s" % ("Test Labels", test_labels.shape)) print("=================================================================")
Don’t forget to normalize after that:
print("Normalize images") train_images = train_images / 255.0 test_images = test_images / 255.0
Finally, the method of printing the relevant information is attached: Complete code defining data:
import os import keras import matplotlib.pyplot as plt from PIL import Image from keras.layers import * from keras.models import * from keras.optimizers import Adam from keras.preprocessing.image import ImageDataGenerator from sklearn.model_selection import train_test_split os.environ['TF_CPP_MIN_LOG_LEVEL'] = '2' # 支持中文 plt.rcParams['font.sans-serif'] = ['SimHei'] # 用来正常显示中文标签 plt.rcParams['axes.unicode_minus'] = False # 用来正常显示负号 re_load = False re_build = False # re_load = True re_build = True epochs = 50 batch_size = 5 count = 0 max_size = 2000000000 IMG_SIZE_X = 30 IMG_SIZE_Y = 30 np.random.seed(9277) image_path = r'D:\Projects\ImageClassifier\data\set' path = ".\data" data_name = "data.npz" data_path = os.path.join(path, data_name) model_name = "model.h5" model_path = os.path.join(path, model_name) label_name = "labels.txt" label_path = os.path.join(path, label_name) class_names = np.loadtxt(label_path, type("")) print('Load class names') if not os.path.exists(data_path) or re_load: labels = [] images = [] print('Handle images') for index, name in enumerate(class_names): classpath = os.path.join(image_path, name) if not os.path.isdir(classpath): continue limit = 0 for image_name in os.listdir(classpath): if limit >= max_size: break imagepath = os.path.join(classpath, image_name) count = count + 1 limit = limit + 1 img = Image.open(imagepath) img = img.resize((30, 30)) img = img.convert("L") img = np.array(img) img = np.reshape(img, (1, 30, 30)) # img = skimage.io.imread(imagepath, as_grey=True) # if img.shape[2] != 3: # print("{} shape is {}".format(image_name, img.shape)) # continue # data = transform.resize(img, (IMG_SIZE_X, IMG_SIZE_Y)) labels.append([index]) images.append(img) print("{} class: {} {} limit: {} {}" .format(count, index + 1, class_names[index], limit, imagepath)) npy_data = np.array(images) npy_labels = np.array(labels) np.savez(data_path, x=npy_data, y=npy_labels) print("Save images by npz") else: npy_data = np.load(data_path)["x"] npy_labels = np.load(data_path)["y"] print("Load images by npz") image_data = npy_data labels_data = npy_labels print("_________________________________________________________________") print("%-28s %-s" % ("Name", "Shape")) print("=================================================================") print("%-28s %-s" % ("Image Data", image_data.shape)) print("%-28s %-s" % ("Labels Data", labels_data.shape)) print("=================================================================") train_images, test_images, train_labels, test_labels = \ train_test_split(image_data, labels_data, test_size=0.2, random_state=6) print('Split train and test data,p=%') print("_________________________________________________________________") print("%-28s %-s" % ("Name", "Shape")) print("=================================================================") print("%-28s %-s" % ("Train Images", train_images.shape)) print("%-28s %-s" % ("Test Images", test_images.shape)) print("%-28s %-s" % ("Train Labels", train_labels.shape)) print("%-28s %-s" % ("Test Labels", test_labels.shape)) print("=================================================================") # 归一化 # 我们将这些值缩小到 0 到 1 之间,然后将其馈送到神经网络模型。为此,将图像组件的数据类型从整数转换为浮点数,然后除以 255。以下是预处理图像的函数: # 务必要以相同的方式对训练集和测试集进行预处理: print("Normalize images") train_images = train_images / 255.0 test_images = test_images / 255.0
The above is the detailed content of Introduction to the method of reading custom data for Tensorflow classifier project (code example). For more information, please follow other related articles on the PHP Chinese website!

Hot AI Tools

Undresser.AI Undress
AI-powered app for creating realistic nude photos

AI Clothes Remover
Online AI tool for removing clothes from photos.

Undress AI Tool
Undress images for free

Clothoff.io
AI clothes remover

AI Hentai Generator
Generate AI Hentai for free.

Hot Article

Hot Tools

Notepad++7.3.1
Easy-to-use and free code editor

SublimeText3 Chinese version
Chinese version, very easy to use

Zend Studio 13.0.1
Powerful PHP integrated development environment

Dreamweaver CS6
Visual web development tools

SublimeText3 Mac version
God-level code editing software (SublimeText3)

Hot Topics
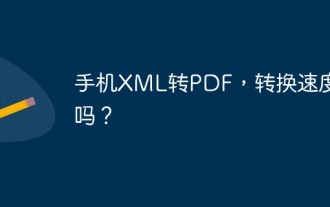
The speed of mobile XML to PDF depends on the following factors: the complexity of XML structure. Mobile hardware configuration conversion method (library, algorithm) code quality optimization methods (select efficient libraries, optimize algorithms, cache data, and utilize multi-threading). Overall, there is no absolute answer and it needs to be optimized according to the specific situation.
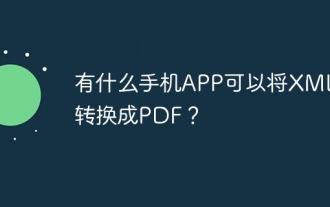
An application that converts XML directly to PDF cannot be found because they are two fundamentally different formats. XML is used to store data, while PDF is used to display documents. To complete the transformation, you can use programming languages and libraries such as Python and ReportLab to parse XML data and generate PDF documents.
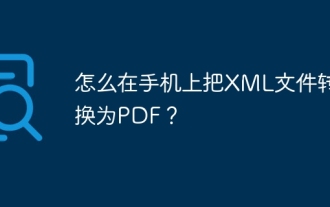
It is impossible to complete XML to PDF conversion directly on your phone with a single application. It is necessary to use cloud services, which can be achieved through two steps: 1. Convert XML to PDF in the cloud, 2. Access or download the converted PDF file on the mobile phone.
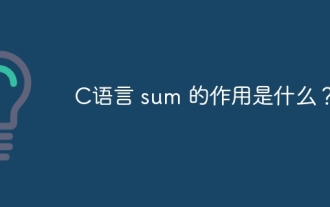
There is no built-in sum function in C language, so it needs to be written by yourself. Sum can be achieved by traversing the array and accumulating elements: Loop version: Sum is calculated using for loop and array length. Pointer version: Use pointers to point to array elements, and efficient summing is achieved through self-increment pointers. Dynamically allocate array version: Dynamically allocate arrays and manage memory yourself, ensuring that allocated memory is freed to prevent memory leaks.
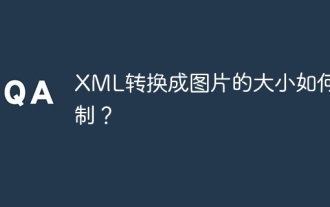
To generate images through XML, you need to use graph libraries (such as Pillow and JFreeChart) as bridges to generate images based on metadata (size, color) in XML. The key to controlling the size of the image is to adjust the values of the <width> and <height> tags in XML. However, in practical applications, the complexity of XML structure, the fineness of graph drawing, the speed of image generation and memory consumption, and the selection of image formats all have an impact on the generated image size. Therefore, it is necessary to have a deep understanding of XML structure, proficient in the graphics library, and consider factors such as optimization algorithms and image format selection.
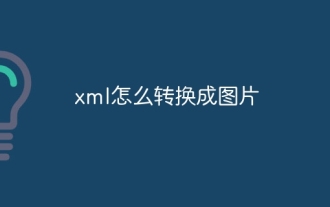
XML can be converted to images by using an XSLT converter or image library. XSLT Converter: Use an XSLT processor and stylesheet to convert XML to images. Image Library: Use libraries such as PIL or ImageMagick to create images from XML data, such as drawing shapes and text.
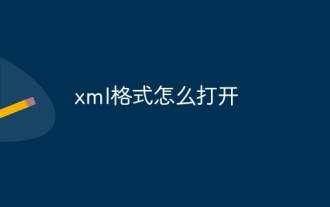
Use most text editors to open XML files; if you need a more intuitive tree display, you can use an XML editor, such as Oxygen XML Editor or XMLSpy; if you process XML data in a program, you need to use a programming language (such as Python) and XML libraries (such as xml.etree.ElementTree) to parse.
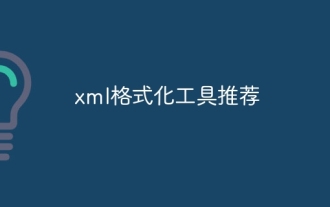
XML formatting tools can type code according to rules to improve readability and understanding. When selecting a tool, pay attention to customization capabilities, handling of special circumstances, performance and ease of use. Commonly used tool types include online tools, IDE plug-ins, and command-line tools.
