


Introduction to the usage of Python descriptors (with examples)
This article brings you an introduction to the usage of Python descriptors (with examples). It has certain reference value. Friends in need can refer to it. I hope it will be helpful to you.
As a python user, you may have been using python for a while, but you may not have used descriptors in python. Next is an introduction to the use of descriptors
Scene introduction
In order to introduce the use of descriptors, we first design a very simple class:
class Product(): def __init__(self,name,quantity,price): self.name = name self.quantity = quantity self.price = price
This is a commodity class that stores the name, quantity and price of the commodity.
For a product, we generally expect that its quantity and price will not be negative. In order to avoid this situation, we can add some judgments during initialization, such as the following:
class Product(): def __init__(self,name,quantity,price): self.name = name if quantity<0: raise ValueError('quantity must be >= 0') self.quantity = quantity if quantity<0: raise ValueError('price must be >= 0') self.price = price
But there is also a disadvantage in that this judgment is only added during initialization, and then when assigning attributes to the class instance, there is still no guarantee that the assigned value is greater than 0
So we can use 'features' to solve this problem:
class Product(): def __init__(self,name,quantity,price): self.name = name self.quantity = quantity self.price = price @property def quantity(self): return self._quantity @quantity.setter def quantity(self,value): if value < 0: raise ValueError('quantity must be >= 0') else: self._quantity = value @property def price(self): return self._price @price.setter def price(self, value): if value < 0: raise ValueError('price must be >= 0') else: self._price = value book = Product('mybook',6,30) print(book.quantity)
The @property and @quantity.setter here are two decorators, which can set the reading and writing of properties, which is equivalent to reading and writing properties. , but it actually executes a function. You can look it up by yourself for the specific introduction of the features. The main purpose here is to elicit the descriptor.
Through attributes, you can add judgment when assigning values to attributes. But when there are more attributes in a class, and many attributes also need to add checks for non-negative assignments, using attributes will be too cumbersome, there will be a lot of code duplication, and a lot of decorators will be added. You can use descriptors to solve this problem.
Using descriptors
First look at the concept of descriptors
A descriptor is an object attribute of "binding behavior". In the descriptor protocol, it can be passed Method fills access to properties. These methods include get(), set(), and delete(). If any of these methods is defined in an object, the object is a descriptor
(These methods are special methods, double The underline is not displayed due to conversion)
We first modify the product class above according to the use of descriptors:
class NotNegative(): def __init__(self,name): self.name = name def __set__(self, instance, value): if value < 0: raise ValueError(self.name+' must be >= 0') else: instance.__dict__[self.name] = value class Product(): quantity = NotNegative('quantity') price = NotNegative('price') def __init__(self,name,quantity,price): self.name = name self.quantity = quantity self.price = price book = Product('mybook',2,5)
NotNegative is the descriptor class, which is the class attribute of the Product class
In this example, if book.quantity=3 is executed, the interpreter will first search for the instance attributes and find that there is a quantity attribute, but the interpreter also finds that there is also a class attribute that is a descriptor, so the interpreter will eventually choose to go Descriptor for this path. Then because it is a descriptor, the set special method in the descriptor will be executed.
The parameters of the set special method in the descriptor are
self: It is the descriptor instance
instance: It is equivalent to the instance book
## in the example #value: It is the value to be assigned Since these attributes have no special requirements for the value, the get special method is not implemented in the example. The get method also has 3 parameters: self, instance, owner. self, instance are the same as those in set, and owner is the Product class in the example Next, we will mainly look at the operations performed in the else part of the descriptor set methodinstance.__dict__[self.name] = value
The above is the detailed content of Introduction to the usage of Python descriptors (with examples). For more information, please follow other related articles on the PHP Chinese website!

Hot AI Tools

Undresser.AI Undress
AI-powered app for creating realistic nude photos

AI Clothes Remover
Online AI tool for removing clothes from photos.

Undress AI Tool
Undress images for free

Clothoff.io
AI clothes remover

AI Hentai Generator
Generate AI Hentai for free.

Hot Article

Hot Tools

Notepad++7.3.1
Easy-to-use and free code editor

SublimeText3 Chinese version
Chinese version, very easy to use

Zend Studio 13.0.1
Powerful PHP integrated development environment

Dreamweaver CS6
Visual web development tools

SublimeText3 Mac version
God-level code editing software (SublimeText3)

Hot Topics


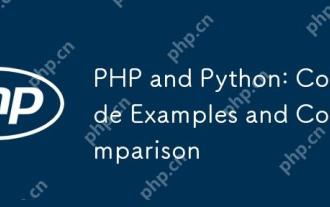
PHP and Python have their own advantages and disadvantages, and the choice depends on project needs and personal preferences. 1.PHP is suitable for rapid development and maintenance of large-scale web applications. 2. Python dominates the field of data science and machine learning.
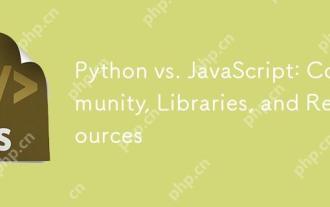
Python and JavaScript have their own advantages and disadvantages in terms of community, libraries and resources. 1) The Python community is friendly and suitable for beginners, but the front-end development resources are not as rich as JavaScript. 2) Python is powerful in data science and machine learning libraries, while JavaScript is better in front-end development libraries and frameworks. 3) Both have rich learning resources, but Python is suitable for starting with official documents, while JavaScript is better with MDNWebDocs. The choice should be based on project needs and personal interests.
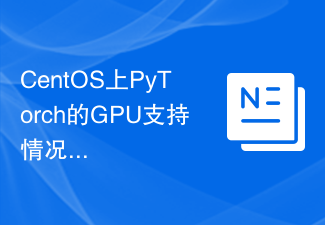
Enable PyTorch GPU acceleration on CentOS system requires the installation of CUDA, cuDNN and GPU versions of PyTorch. The following steps will guide you through the process: CUDA and cuDNN installation determine CUDA version compatibility: Use the nvidia-smi command to view the CUDA version supported by your NVIDIA graphics card. For example, your MX450 graphics card may support CUDA11.1 or higher. Download and install CUDAToolkit: Visit the official website of NVIDIACUDAToolkit and download and install the corresponding version according to the highest CUDA version supported by your graphics card. Install cuDNN library:
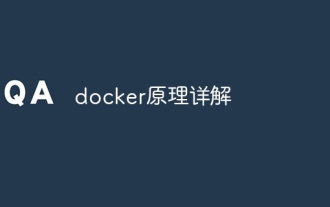
Docker uses Linux kernel features to provide an efficient and isolated application running environment. Its working principle is as follows: 1. The mirror is used as a read-only template, which contains everything you need to run the application; 2. The Union File System (UnionFS) stacks multiple file systems, only storing the differences, saving space and speeding up; 3. The daemon manages the mirrors and containers, and the client uses them for interaction; 4. Namespaces and cgroups implement container isolation and resource limitations; 5. Multiple network modes support container interconnection. Only by understanding these core concepts can you better utilize Docker.
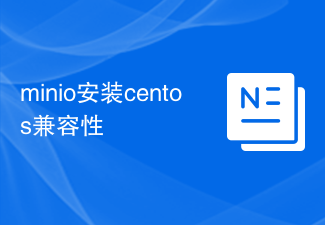
MinIO Object Storage: High-performance deployment under CentOS system MinIO is a high-performance, distributed object storage system developed based on the Go language, compatible with AmazonS3. It supports a variety of client languages, including Java, Python, JavaScript, and Go. This article will briefly introduce the installation and compatibility of MinIO on CentOS systems. CentOS version compatibility MinIO has been verified on multiple CentOS versions, including but not limited to: CentOS7.9: Provides a complete installation guide covering cluster configuration, environment preparation, configuration file settings, disk partitioning, and MinI
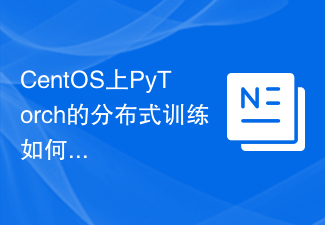
PyTorch distributed training on CentOS system requires the following steps: PyTorch installation: The premise is that Python and pip are installed in CentOS system. Depending on your CUDA version, get the appropriate installation command from the PyTorch official website. For CPU-only training, you can use the following command: pipinstalltorchtorchvisiontorchaudio If you need GPU support, make sure that the corresponding version of CUDA and cuDNN are installed and use the corresponding PyTorch version for installation. Distributed environment configuration: Distributed training usually requires multiple machines or single-machine multiple GPUs. Place
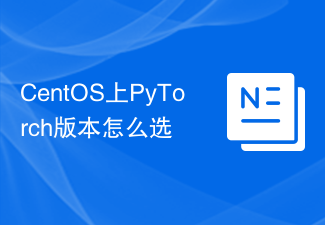
When installing PyTorch on CentOS system, you need to carefully select the appropriate version and consider the following key factors: 1. System environment compatibility: Operating system: It is recommended to use CentOS7 or higher. CUDA and cuDNN:PyTorch version and CUDA version are closely related. For example, PyTorch1.9.0 requires CUDA11.1, while PyTorch2.0.1 requires CUDA11.3. The cuDNN version must also match the CUDA version. Before selecting the PyTorch version, be sure to confirm that compatible CUDA and cuDNN versions have been installed. Python version: PyTorch official branch
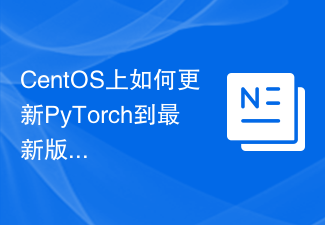
Updating PyTorch to the latest version on CentOS can follow the following steps: Method 1: Updating pip with pip: First make sure your pip is the latest version, because older versions of pip may not be able to properly install the latest version of PyTorch. pipinstall--upgradepip uninstalls old version of PyTorch (if installed): pipuninstalltorchtorchvisiontorchaudio installation latest
